Sleep Technology: A Global Perspective
Tripat Deep Singh
LEARNING OBJECTIVES
On completion of this chapter, the reader should be able to:
1. Discuss new developments in sleep technology and sleep diagnostics.
2. Describe the role of computer-assisted scoring and interscorer reliability (ISR).
3. Discuss the role of consumer sleep technologies (CSTs).
4. Describe the role of home sleep apnea testing (HSAT).
5. Describe the development of the global sleep medicine and technology field.
KEY TERMS
Chemogenetics
CRISPR-Cas system
Gene therapy
Home sleep apnea testing (HSAT)
Optogenetics
INTRODUCTION
Sleep technology has evolved rapidly in the last 50 years. Polysomnography (PSG) has transitioned from analog to digital machines, positive airway pressure (PAP) therapy was identified as a treatment for obstructive sleep apnea (OSA), and various advanced forms of PAP therapy and interfaces were introduced to treat sleep-disordered breathing (SDB). In the last decade with advancements in mobile phone technology, mobile apps and wearables have been marketed to monitor health including sleep.
The first professional bodies involved in the development of sleep medicine and sleep technology were established in the United States and Europe. In the past 20 years, additional professional bodies have formed including in many countries that are performing important work in the development of sleep medicine and technology.
MECHANISMS OF SLEEP AND WAKEFULNESS
Hans Berger, a German psychiatrist, discovered electroencephalography (EEG) in 1929 (1), which later led to the study of sleep states in humans and the discovery of rapid eye movement (REM) sleep (2). Jouvet, Bremer, and Moruzzi used brain cuttings to study sleep and wakefulness in animals (3, 4). To study the role of specific cell groups and neurochemicals, researchers used various techniques like cell-specific lesions (5, 6), cellular electrophysiology (7), and measurement of neurotransmitters (8, 9, 10). With c-Fos technique, it became clear that these neurons were embedded in groups of neurons controlling other behaviors, but how they also controlled specific sleep behaviors was unclear (11, 12). Newer techniques like genetic approaches, gene therapy, optogenetics, and chemogenetics and clustered regularly interspaced short palindromic repeats (CRISPR)-Cas now allow researchers to understand the finer details of sleep-wake regulation and are also assisting to better understand the causes and treatments of sleep disorders.
The genetic approach has improved understanding of two sleep disorders: narcolepsy and advanced sleep phase syndrome. Emmanuel Mignot found mutation in hypocretin receptor 2 in canine narcolepsy using forward genetics, and Masashi Yanagisawa’s group found narcoleptic symptoms in orexin knockout mouse (13). In advanced sleep phase syndrome, forward genetics revealed that individuals with this disorder have a mutation in the hPer2 gene (14), and reverse genetics established that mice with this mutation also demonstrated a shorter period of sleep-activity cycle (15).
Optogenetics
Optogenetics (“opto” for optical stimulation and “ genetics” for genetically targeted cell types) uses light-sensitive proteins channelrhodopsin-2 (ChR2) and halorhodopsin (NpHR) to either activate or inhibit, respectively, the activity of neurons in which they are targeted (16). The ChR2-expressing cells depolarize when exposed to blue light, and NpHR-expressing cells hyperpolarize when exposed to yellow light. These two proteins can be inserted into specific cells, which allow to control the activity of a particular cell in neural network with millisecond precision.
Optogenetics has helped resolve the mystery of which neurons, cholinergic or γ-aminobutyric acid (GABA), in the basal forebrain produce wakefulness. GABA neurons in the basal forebrain are active during wakefulness (17) and hypocretin cells function to promote arousal (18, 19). Optogenetic stimulation helped to identify specific cell groups in the basal forebrain that promote nonrapid eye movement (NREM) sleep; optogenetic stimulation of GABA+ somatostatin neurons in the basal forebrain produced transition from wakefulness to NREM sleep and also increased the amount of NREM sleep (17).
Melanin-concentrating hormone (MCH) neurons fire during NREM sleep and become most active during REM sleep (20). Optogenetic technique provided new evidence about the role of MCH neurons in sleep; MCH neurons increased both NREM and REM sleep and delta power. They also increased sleep at night, suggesting that MCH neurons inhibit arousal-promoting circuits and drive sleep in mammals (21, 22). Activation of MCH neurons during NREM sleep produces transition to REM sleep (23).
Optogenetics has highlighted the role of the ventral medulla in the control of REM sleep. Optogenetically activating GABA cells in the ventral medulla increase the probability of REM sleep episodes, and chemogenetic inactivation of these same cells reduced REM sleep (24). For the first time with optogenetic stimulation of non-neural structure, glial cells in the hypothalamus show that glial cell activation increases both NREM and REM sleep (25). It is thought that it is glial rather than neuronal adenosine that may regulate the homeostatic sleep drive response before waking (26).
Astrocytes are a key component of a glymphatic system in the central nervous system that is responsible for waste disposal and distribution of lipids, glucose, and other nutrients (27). The glymphatic system is active during natural sleep and anesthesia but not during wakefulness (28). The glymphatic system also clears brain lactate that accumulates during wakefulness (29). These studies provide the first direct evidence of the role of sleep in clearing brain waste accumulated during wakefulness. One of the drawbacks of optogenetics is that this technique generates heat, and this may be problematic if cells under study are heat sensitive. Also, if cells under study are scattered, the light may not be able to reach all the opsin-containing cells.
Chemogenetics
Also called pharmacogenetics, a drug-based approach is used to control cell activity. Chemogenetics differs from optogenetics in that it can activate or suppress the activity of broadly scattered neuron populations, which makes it a more useful approach than optogenetics for manipulating distributed cell groups. This technique has contributed to the understanding of sleep-wake mechanisms including the following:
Activation of hypocretin neurons increasing wakefulness (30)
Activation of GABAergic neurons in the medullary parafacial zone rapidly inducing NREM sleep regardless of circadian time (33)
Inactivation of GABA cells in the ventral medulla increasing wakefulness and decreasing NREM and REM sleep (24)
Activation of MCH neurons leading to increase in slow-wave sleep (SWS) depth and acceleration in the extinction of SWS episodes. These two processes facilitate the transition from SWS to REM sleep and assist in REM sleep onset (34).
Gene Therapy
CRISPR-Cas System
The CRISPR-Cas system is a new gene-editing tool that allows researchers to delete, silence, enhance, or insert genes at a precise location in the genome (39, 40, 41). CRISPR is a segment of DNA containing short repetitions of base sequences, with each repeat sequence followed by pieces of spacer DNA. This technique has been used to show the role of the entire N-methyl-D-aspartate receptor family in sleep regulation in mice (42). This technique can be applied across all eukaryotes including humans and reduces the time, cost, and effort compared with conventional genetic strategies of gene manipulation.
TECHNOLOGY AND SLEEP DIAGNOSTICS
Computer-Assisted Sleep Scoring and Interscorer Reliability
Manual scoring of sleep and associated events has been the standard worldwide, and interscorer reliability (ISR) is an important issue in quality control. The American Academy of Sleep Medicine (AASM) frequently revises the scoring manual, the latest manual being released in April 2018, to refine the scoring rules and improve ISR.
Younes et al. have highlighted that inattention errors and bias contribute little to interscorer variability (43); rather, the difference is due to equivocal epochs that can be assigned one or two sleep stages by trained technologists (43). They indicate digital information regarding key sleep staging variables (spindles, delta wave duration, and objective sleep depth) is needed to reduce scoring variability and that better training or refining the scoring rules is not going to improve ISR (43). As a continuation to this study, Younes et al. showed that providing digital information regarding key sleep staging variables (spindles, delta wave duration, and objective sleep depth) improved ISR (44). Younes et al. also showed that manual scoring of NREM sleep stages is highly variable and unreliable among experienced sleep technologists (45). Technologists varied widely in scoring N1 and N3 sleep stages (45). The AASM also reported the lowest agreement in scoring of N1 and N3 sleep among different scorers globally in their ISR program (46). They also identified that scoring the first epoch of N3 after N2 sleep has low agreement among scorers and suggested the use of automated analysis of slow-wave activity as a potential solution (46). Several computer-assisted sleep scoring systems have been validated against human scoring, which has not only improved interscorer variability but also shortened the time required to score one sleep study considerably (43, 44, 47, 48). Recently, researchers in Singapore developed a real-time automatic sleep staging system that can be cloud based, allowing multiple users to perform real-time sleep staging at the same time (49). This system can score an entire night’s sleep recording with an accuracy on par with expert human scorers but much faster (5 seconds compared with 30 to 60 minutes). This system also facilitates the automatic delivery of acoustic stimuli at the targeted phase of slow-sleep oscillations to enhance SWS (49).
It requires considerable practice and knowledge to learn manual sleep staging. There are very few technologists trained in manual scoring of sleep stages in developing countries, which is a limiting factor in starting more sleep labs in these countries. Computer-assisted sleep scoring systems make scoring simple and may be very useful in extending the reach of sleep facilities to patients in developing countries.
Automated scoring of respiratory events faces several impediments: determining the baseline to compute a 30% or 90% reduction in flow amplitude to score hypopneas or apneas, measuring airflow with nasal pressure and not with a pneumotachograph, and therefore overestimating the flow signal at small amplitudes with oronasal interfaces and difficulty automating methods (flow morphology, paradox between thorax and abdominal belts) on which manual scoring of obstructive versus central hypopnea depends (50).
Two methods have been described to automate the scoring of EEG arousals based on absolute power of EEG (51, 52). Both methods are limited because the absolute power of EEG may be affected by scalp properties and impedance values (43, 44, 47, 48). These computer-assisted scoring systems should not be a threat to sleep technologists. The sleep technologist can focus more on patient-centric activities like educating the patients about disease, explaining PAP therapy, proper mask fit, and troubleshooting their issues with PAP therapy. The role of experienced technologists is indispensable even if these new technologies are adopted in the day-to-day practice of sleep labs.
Consumer Sleep Technologies
Consumer sleep technologies (CSTs) have become very popular within our society for self-monitoring sleep. They are primarily used for sleep induction, wake induction, self-guided sleep assessment, entertainment, social connection, information sharing, and sleep education (53). A recent review classified the primary delivery platforms for CSTs into five categories:
Mobile device platforms,
Wearable platforms,
Embedded platforms,
Desktop or website platforms, and
Accessory appliance platforms (53).
The manufacturers of these technologies update them at a rapid pace and the medical community cannot keep up. By the time any study is published validating the technology, a new version of the technology has already been released.
A recent study reported that only 32.9% of sleep apps contained information supporting their claims, 15.8% included clinical input, and 13.2% contained links to sleep literature. Also, apps contained information on how sleep is affected by alcohol or drugs (23.7%), food, daily activities, and stress (13.2%). Users gave a high rating to apps that contained a sleep tip function (54). However, no guidelines or validation of data generated by CSTs shows how sleep is affected by different disease states and how to use the data to manage different disease states long term.
Recently, a study protocol published studying the clinical applicability of a wearable device (Fitbit Charge HR or Fitbit Charge 2) generated data on the management of thyrotoxicosis by analyzing continuously monitored data for heart rate, physical activity, and sleep in patients with thyrotoxicosis during their clinical course after treatment (55). In patients with major depressive disorders, Fitbit Flex (FBF) in a normal setting significantly overestimated sleep time and efficiency and displayed poor ability to correctly identify wake epochs.
In the sensitive setting, the FBF significantly underestimated sleep time and efficiency relative to PSG (56).
In the sensitive setting, the FBF significantly underestimated sleep time and efficiency relative to PSG (56).
Validation of data across different age groups is not available, and scant available data show different results across age groups. In healthy adults, Fitbit Charge 2 when compared with gold standard PSG overestimated total sleep time (TST) and time spent in N1 + N2 sleep stage and underestimated sleep onset latency and stage N3 but did not differ in estimation of wake time after sleep onset and time spent in REM sleep. Fitbit Charge 2 correctly identified 82% of PSG-defined NREM-REM sleep cycles across the night in healthy adults and also in subjects with periodic leg movements during sleep (57). In the pediatric population (3 to 17 years), Fitbit Ultra significantly overestimated TST (41 minutes) and sleep efficiency (SE) (8%) in normal mode and underestimated TST (105 minutes) and SE (21%) in sensitive mode (58). In adolescents, Fitbit Charge HR significantly but negligibly overestimated TST by 8 minutes and sleep efficiency by 1.8% and underestimated wake time after sleep onset by 5.6 minutes (p < 0.05) (59, 60).
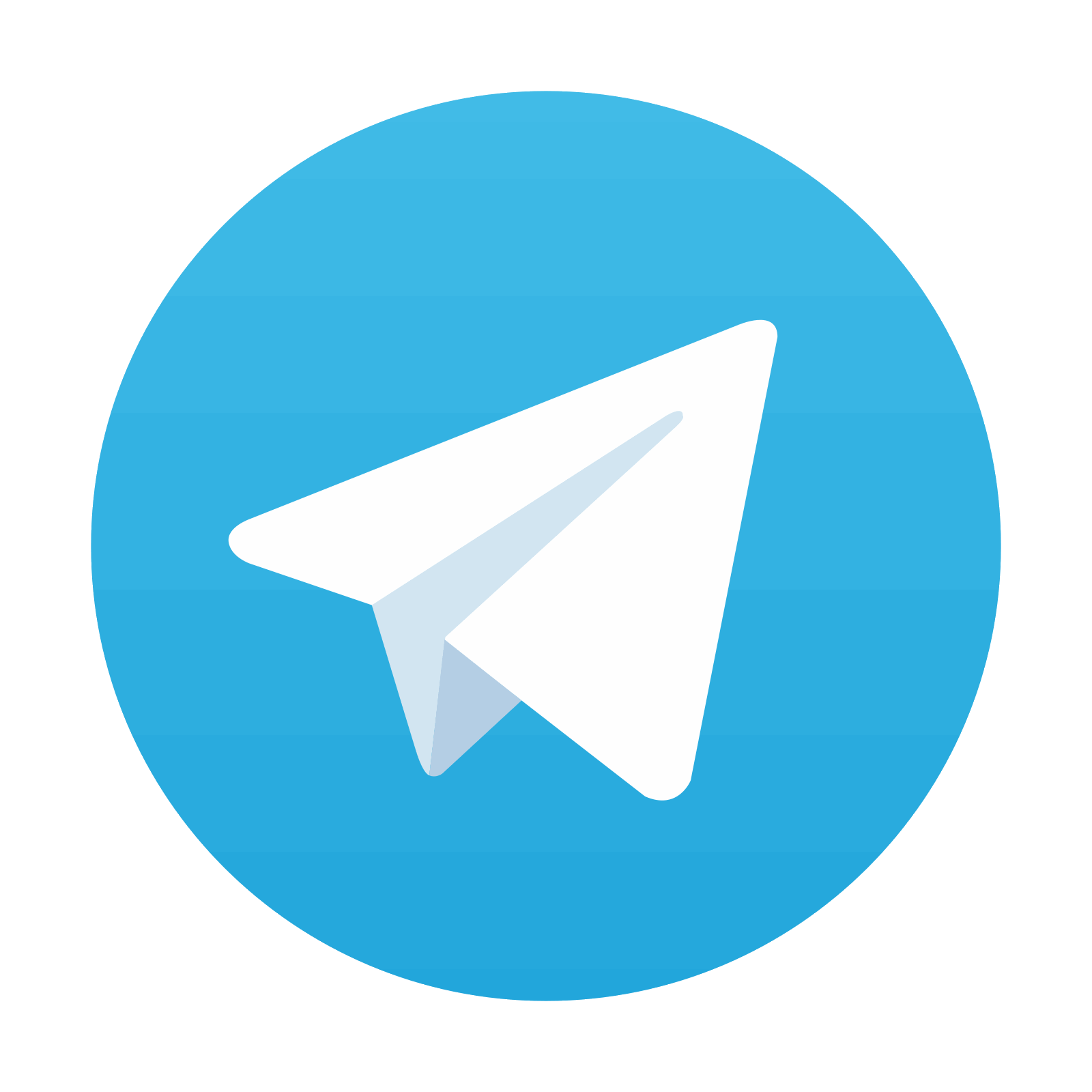
Stay updated, free articles. Join our Telegram channel

Full access? Get Clinical Tree
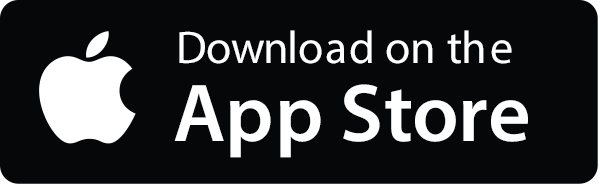
