Fig. 1
Functional neuroanatomy of SWS and REM sleep. Brain sections showing brain areas where regional cerebral blood flow (rCBF) was negatively correlated with the density of slow oscillations (delta power) during SWS (left panel) or increased during REM sleep as compared to wakefulness (right panel). Adapted with permission from Dang-Vu et al. (2005) and Maquet et al. (1996)
1.2 Rapid Eye Movements Sleep
In contrast to NREM sleep, REM sleep and wakefulness are characterized by sustained, desynchronized neuronal activity (Steriade and McCarley 1990) associated with high energy demands. Therefore, activity as measured using PET should be increased in brain regions actively involved in REM sleep as compared to NREM sleep, even above activity levels observed during wakefulness. Accordingly, regional patterns of activation have been observed during REM sleep using H2 15O-PET (Braun et al. 1997; Maquet et al. 1996). These studies evidenced markedly increased rCBF in the mesopontine tegmentum, the thalamic nuclei, limbic (amygdaloid complexes, hippocampal formation, anterior cingulate cortex) and temporo-occipital areas (Fig. 1). Conversely, activity in the dorso-lateral prefrontal cortex, parietal cortex, as well as the posterior cingulate cortex and precuneus was reduced as compared to the awake resting-state. These results confirmed animal reports (e.g. Lydic et al. 1991) suggesting a heterogeneous and regionally specific distribution of telencephalic activity during REM sleep. Additionally, temporal correlations between neural activity in the amygdala and neuronal activity in the occipito-temporal areas during REM sleep suggested a modulation of these cortical areas by the limbic system (Maquet and Phillips 1998), which might participate in the mechanisms underlying dreaming activity (Schwartz and Maquet 2002) and emotional memory processing. H2 15O PET results also supported the hypothesis of the existence of ponto-geniculo-occipital (PGO) waves in human (Peigneux et al. 2001). As evidenced in animal studies, PGO waves are responsible for the generation of REMs, and trigger cellular processes thought to favour brain plasticity during REM sleep (Datta 1999).
2 Transient and Oscillatory Processes in Sleep
Beyond regional patterns of sustained cerebral activity as evidenced by PET, functional magnetic resonance imaging (fMRI) has become a powerful tool for the description of the functional neuroanatomy of transient and oscillatory processes in sleep. fMRI, which records cerebral activity at the scale of the second, provides a more accurate temporal resolution than PET together with a good localizing power in the whole brain. A main disadvantage is the subject’s discomfort in the scanner, due to elevated noise levels and the restricted space within the magnet. Nowadays, technical difficulties to get suitable EEG signal needed for the identification of sleep stages and characterization of sleep events in the fMRI environment are no longer an obstacle to research.
2.1 Stimulating the Brain to Understand the Neurophysiology of Sleep
Functional MRI studies initially focused on the cerebral correlates of auditory stimulations as a probe for the investigation of sleep neuroanatomy (Czisch et al. 2002, 2004; Kaufmann et al. 2006; Portas et al. 2000; Wehrle et al. 2007). The choice of auditory stimulations in sleep was mostly for practical and technical reasons, as it is rather easy to deliver sounds at non-awakening threshold in the context of the magnetic environment, as compared to e.g. tactile stimulations. Results of these studies mostly confirmed PET findings in terms of sleep stage-specific (Czisch et al. 2002, 2004; Kaufmann et al. 2006; Portas et al. 2000; Wehrle et al. 2007) and PGO-related (Kaufmann et al. 2006) activations patterns, further disclosing auditory reactivity differences in specific brain networks between phasic and tonic REM sleep episodes (Wehrle et al. 2007).
2.2 Endogenous Brain Stimulation: The up and down of Slow Oscillations and Spindles
Simultaneous EEG/fMRI recordings have enabled researchers to revisit the PET-based concept of SWS as a stage of brain quiescence, reputedly characterized by global and regional decreases of brain activity. Indeed, animal research taught us that cortico-thalamocortical loops are involved in the generation of EEG spindles (11–15 Hz) and delta waves (0.5–4 Hz), which in turn are organized by cortically generated slow oscillations (<1 Hz; Steriade 2001), and that these rhythms (under the control of brainstem and forebrain modulatory systems) are combined within specific time windows corresponding to the depolarizing phase (up state) of the slow oscillations (Steriade 2005). Dang-Vu et al. (2008) used an endogenous event-related approach to investigate using fMRI the transient changes in regional cerebral activity specifically associated with slow waves (<1 Hz; >140 µV) and delta waves (0.5–4 Hz; 75–140 µV) during NREM sleep. That is, the events of interest were defined based on the participant’s own neurophysiological activity, e.g. the rising slope of each slow wave during SWS, rather than on the occurrence of an externally delivered, exogenous stimulus (like for instance a sound). Using this approach, they found increased activity coincident with the active depolarized (“up”) state of oscillations (as indexed by peak negativity in the EEG) in several cortical areas including the inferior frontal and medial prefrontal cortices, and the precuneus and posterior cingulate areas, both for slow and delta waves. Besides these commonalities, slow oscillations (<1 Hz) were associated with increased activity in the parahippocampal gyrus, the cerebellum and the brainstem, whereas delta waves were specifically associated with increased activity in frontal areas (Fig. 2). Another study found infraslow (<0.1 Hz) oscillations in the EEG band positively correlated with brain activity mostly in subcortical regions including cerebellum, thalamus, basal ganglia and hippocampus, whereas these infraslow oscillations negatively correlated with cortical activity (Picchioni et al. 2011). Additionally in the Dang-Vu et al. (2008) study, increased activity associated with slow oscillations was found in the midbrain and the pontine tegmentum, a region that includes critical structures involved in the regulation of sleep and wakefulness, and this activation encompassed the noradrenergic locus coeruleus (LC). In line with animal data (Eschenko et al. 2012), these findings suggest that pontine structures are active in phase with slow oscillations, possibly to allow the brain periodically restoring microwake-like activity patterns facilitating neuronal interactions (Dang-Vu et al. 2008). Hence, EEG/fMRI studies confirm that human NREM sleep genuinely is an active state during which phasic increases in brain activity are synchronized to slow oscillations.
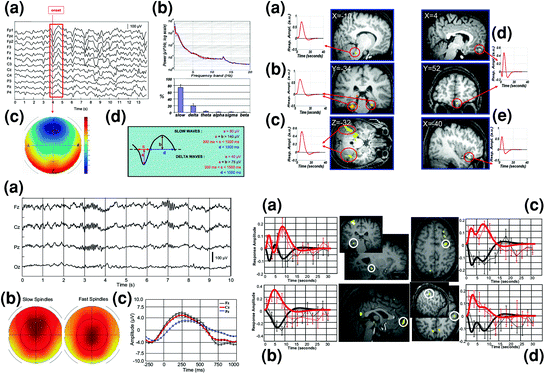
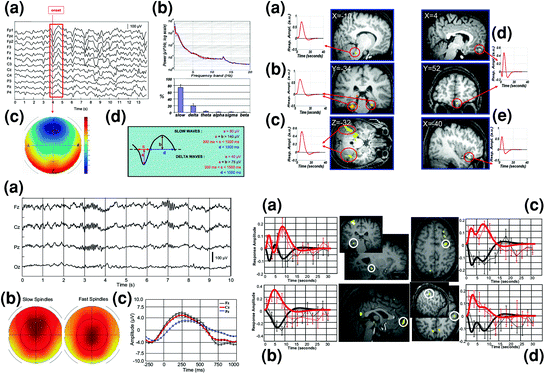
Fig. 2
Slow oscillations and spindles during NREM sleep investigated using endogenous event-related EEG-fMRI. Left top panel shows a detection of the onset of sleep EEG slow oscillations (peak negativity for sample wave framed in red); b EEG power spectrum; c topographical distribution of slow wave sleep epochs; and d criteria for separate detection of slow and delta waves. Right top panel shows brain regions specifically activated in relation to high amplitude slow waves (<1 Hz) (a Pontine tegmentum, b Parahippocampal gyrus, c Cerebellum) or in relation to delta waves (1–4 Hz) (d Medial prefrontal cortex, e Inferior frontal gyrus). Adapted with permission from Dang-Vu et al. 2008. Left bottom panel shows EEG characterization of sleep spindles with a representative spindles on a typical stage 2 sleep EEG recording after correction for fMRI-related artifacts; b topographical distribution of slow (11–13 Hz) and fast (13–15 Hz) spindles; and c EEG data (0.5–4 Hz) averaged with respect to the onset of all sleep spindles, showing that spindles start (time 0) on the depolarizing phase of the oscillation. Right bottom panel depicts differential fMRI activity (BOLD response) between fast and slow spindles. Larger brain responses for fast (red) than slow (black) spindles are revealed in the hippocampus (a), mesial prefrontal cortex (b), precentral gyrus (c), and postcentral gyrus (d). Side panels show the differential evolution of BOLD responses in fast and slow spindles. Adapted with permission from Schabus et al. 2007
Another endogenous event-related fMRI study showed that the occurrence of phasic sleep spindles during NREM sleep is associated with increased brain activity in a cortico-subcortical network including the thalamus, paralimbic areas, and superior temporal gyri (Schabus et al. 2007). Besides, activity in different thalamo-cortical networks was associated with fast (13–15 Hz) and slow (11–13 Hz) spindles. Indeed, whereas slow spindles were associated with activation of the superior temporal gyrus, fast spindles preferentially recruited hippocampal and sensorimotor cortical areas, further supporting the hypothesis that fast and slow spindles rely upon two functionally separated systems (Fig. 2). Another study similarly found increased spindle-related activity in the thalami, putamen, posterior cingulate, paracentral and temporal cortices, and an additional association between the occurrence of K-complexes and increased activity in the thalami, superior temporal and medial occipital, parietal and frontal areas (Caporro et al. 2012).
2.3 Endogenous Activity Modulates Brain Responsiveness to External Stimulation
Both spindles and slow oscillations can modulate brain responsiveness to auditory stimulation during NREM sleep. Two studies showed persistent wake-like responses during NREM sleep except when spindles were present (Dang-Vu et al. 2011; Schabus et al. 2012) or stimulations occurred in the downward slope of the slow oscillation (Schabus et al. 2012), during which responses became less consistent or even absent. Together with distinct N550 EEG responses to tones during sleep spindles (Schabus et al. 2012), this suggests that the brain is more responsive during the upward slope of the slow oscillation during deep NREM sleep. The authors propose that the presence of short temporal windows during which the brain is open to external stimuli during NREM sleep is consistent with the fact that even during deep sleep meaningful events can be detected.
2.4 Dynamic Interactions in REM Sleep
Although REM sleep has been less thoroughly investigated using this technique, fMRI resting-state connectivity analyses showed that activity in the so-called default-mode network (Raichle et al. 2001) is functionally uncoupled during NREM sleep (i.e. there is diminished connectivity) then recoupled during REM sleep where it is similar to wakefulness (Chow et al. 2013). Additionally however, REM sleep was specifically characterized by a widespread, temporally dynamic interaction between unimodal sensorimotor areas and higher-order association cortices (including in the default-mode network). Indeed, the two systems become anticorrelated and fluctuated rhythmically, reciprocally alternating epochs with a frequency ranging from 0.1 to 0.01 Hz. The functionality of these slowly alternating activation patterns remains unknown.
2.5 Phasic REM Events and the Source of Spindles
Finally, magnetoencephalography (MEG) is distinct from fMRI in that it is noise-free and operates on a neuronal timescale, i.e. within the scale of milliseconds while having good localizing power. Hence, its features offer interesting opportunities to track the time course and spatial evolution of the magnetic correlates of cerebral activity associated with both endogenous and exogenous events during sleep. For instance, MEG was used to investigate cortical responses to diverse sensory and/or noxious stimuli during sleep (Kakigi et al. 2003; Wang et al. 2004). Furthermore, MEG allowed the differentiation of cerebral networks activated before, during and after ocular saccades initiation during REMs in REM sleep (Corsi-Cabrera et al. 2008; Ioannides et al. 2004), or to compute the source location of maximal spindle activity, which was found in precentral and/or postcentral areas (Gumenyuk et al. 2009). Finally, it is worth noting that combined EEG and MEG studies have revealed heterogeneous MEG sources for human sleep spindles suggesting that multiple generators are active, whereas sleep spindles as measured by the EEG seem to be generated by a different, diffusely synchronous system (Dehghani et al. 2010a, b). These results are in line with animal studies that identified two thalamo-cortical systems, i.e. the core and matrix systems, which produce focal or diffuse activation (Jones 1998). Further imaging (Dehghani et al. 2011) and computational (Bonjean et al. 2012) investigations suggest that synchronous spindles captured by the EEG emerge from asynchronous spindles recorded using MEG, in line with the hypothesis that the spatial coherence for spindles in the EEG is actually a consequence of diffuse matrix projections of the thalamus to layer 1 compared with the focal projections of the core pathway to layer 4 recorded in the MEG (Destexhe and Sejnowski 2003). Hence, MEG represents a complementary and useful non-invasive tool to improve our understanding of the complex neurophysiological mechanisms that subtend sleep phenomena.
3 Neural Models of Memory Consolidation During Sleep
Memory consolidation refers to a temporal process lasting from a few minutes to several years by which initially fragile memory traces undergo a series of transformations eventually leading to their strengthening into long-term memory stores (McGaugh 1966). Memory consolidation and the progressive modification of the acquired information are instances of brain plasticity, i.e. the capacity of the brain to adapt its function and structure over time to accommodate novel experience. Thus reorganization of cortical networks, disinhibition of neuronal assemblies, modification and remodelling of synaptic connections can be seen as instances of memory formation and consolidation processes. In this respect, experimental evidence suggests that the neurophysiological conditions of sleep are favourable for the consolidation of novel memories and their durable inscription in long-term memory stores (Peigneux et al. 2011).
3.1 Neuronal Replay and the Hippocampal-Neocortical Dialogue
It has been proposed that memory consolidation relies on complementary learning systems subtended by different neuroanatomical structures (Marr 1971; McClelland 1994). In the first step, information is quickly learned but only temporarily held into a transitory memory store. Robust encryption then takes place at a slower rate in lasting memory stores. Repetitive interactions between transitory and lasting memory stores allow connections subtending new memory items to be gradually reorganized and reinforced. Hence, two-stage models assume a progressive transfer of the learned information from transitory to lasting memory stores, where it will be progressively integrated into pre-existing networks. Expanding on this framework, the hippocampal-cortical dialogue model (Buzsaki 1996) proposes that memory consolidation takes place through repeated interactions between transitory and lasting long-term memory stores across multiple iterations of the sleep-wake cycle. During wakefulness, learning-related information collected at the neocortical level is transferred and transiently stored in the hippocampus. During NREM sleep, characterized by decreased cholinergic activity, information preferentially flows in the opposite direction, from hippocampal to neocortical stores (Hasselmo 1999).
Consequently, repeated activation of the acquired information in the hippocampus during post-learning NREM sleep will eventually lead to a progressive transfer toward neocortical long-term memory stores. Conversely, higher cholinergic levels during REM sleep would promote information feedback toward hippocampal repositories. Memories will thus be stored and consolidated for the long-term across repeated SWS-REM sleep cycles. It is surmised here that neuronal patterns in hippocampal and neocortical stores are synchronized by the alternation of “up” and “down” states of slow oscillations during SWS (Fig. 3), an interpretation in line with neuroimaging findings reporting differential brain responsiveness between these two states (e.g. see above Dang-Vu et al. 2008, 2011). More precisely, the high-frequency bursts of neuronal activity in the hippocampus (i.e. the sharp wave ripples) and the thalamo-cortical spindles are triggered during the depolarizing (“up”) phase of the slow oscillations. These sharp waves ripples synchronize with spindles that then propagate within the neocortex, inducing long-term potentiation (LTP) processes that would eventually modify synaptic strengths in interconnected neuronal networks (Rosanova and Ulrich 2005), hence promoting a progressive transfer of hippocampus-dependent memories to neocortical stores. It remains debated whether the consolidated information is erased from hippocampal stores upon transfer, or whether hippocampal pointers still contribute retrieving the information stored in long-term memory as proposed by the multiple trace theory (Nadel et al. 2000). It is worth noting here that the hippocampal-neocortical model associates the consolidation process mostly with SWS and hippocampus-dependent declarative and spatial memories. A broader, less-specific variant called the “neuronal replay hypothesis” proposes that cortical or subcortical activation patterns associated with novel learning are reactivated during subsequent sleep stages (either NREM or REM stages), eventually leading to the replay of the learned information and its gradual integration and/or transfer into long-term memory stores, an assumption supported by neuroimaging data showing reactivation of learning-related activity both during REM (Maquet et al. 2000; Peigneux et al. 2003) and NREM sleep (Peigneux et al. 2004; Rasch et al. 2007).
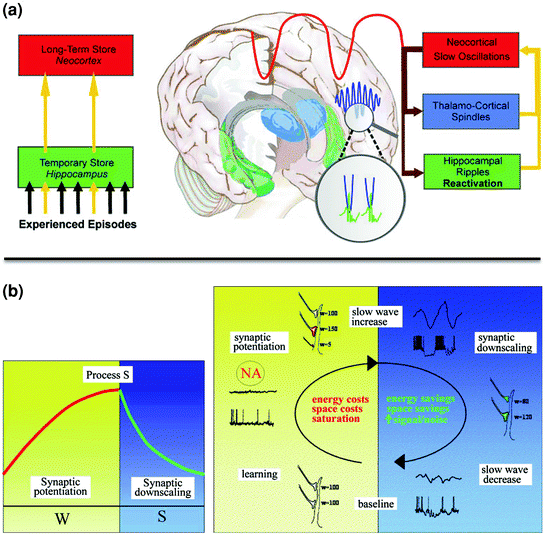
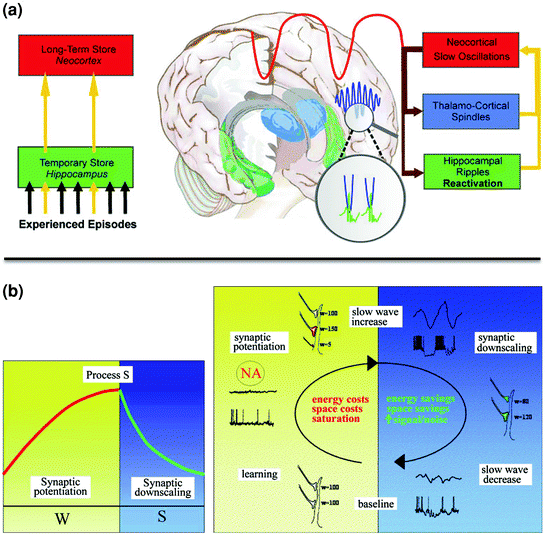
Fig. 3
Neural models of memory consolidation during sleep. a Hippocampal-neocortical dialogue. Information gained during novel experience is transiently stored in the hippocampus, and then progressively transferred into neocortical long-term memory stores. Transfer of information from hippocampal to neocortical areas during NREM sleep is promoted by SWS oscillations. Hippocampal ripples associated with the reactivation of the learned information are triggered during the depolarizing (“up”) phase of the slow oscillations, and synchronized with thalamo-cortical spindles. Reprinted with permission from Born and Wilhelm 2011. b Synaptic homeostasis theory (SHY). Memory acquisition during wakefulness is associated with locally increased synaptic potentiation and elevated energy and space costs, eventually leading to the saturation of the learning-related neuronal network and decreased plasticity. Local neuronal saturation then leads to proportionally increased slow oscillatory activity during post-training NREM sleep, providing the necessary conditions to downscale synaptic strength to baseline level. This depotentiation process improves the signal-to-noise ratio in learning-related neuronal ensembles, consolidating the learned experience. Reprinted with permission from Tononi and Cirelli 2006
3.2 Synaptic Homeostasis and Memory Consolidation
An alternative, but not exclusive model for memory consolidation during sleep is the synaptic homeostasis theory (SHY) (Tononi and Cirelli 2006). The theory proposes that memory consolidation benefits from the local use-dependent synaptic downscaling phenomenon that takes place during sleep, as a secondary consequence of the growth of synaptic connections associated with novel learning at wake. This model posits that memory acquisition during wakefulness is associated with locally increased synaptic potentiation and elevated energy and space costs, eventually leading to the saturation of the learning-related neuronal network and decreased plasticity. Local neuronal saturation will then lead to proportionally increased slow oscillatory activity during post-training NREM sleep (Fig. 3). According to this model, homeostatically regulated slow oscillations during SWS offer optimal conditions to downscale synaptic strength to their baseline level, thus restoring the conditions for synaptic plasticity, while preserving the necessary differentiation between learning-related potentiated and other non-potentiated synapses. Hence slow oscillations would refine the synaptic weight and indirectly improve the signal-to-noise ratio in learning-related neuronal ensembles. In this context, weak memory traces will be removed and stronger traces remain, without the need for a transfer between remote brain structures. Consequently, this theory might be more appropriate to account for the consolidation of memories that are not dependent of the hippocampus, e.g. perceptual or motor memories processed at the neocortical level. In man, support for this theory was found in EEG studies showing locally increased slow oscillations during post-training NREM sleep in brain areas involved in a motor adaptation process (Huber et al. 2004; Landsness et al. 2009).
3.3 Dissociated and Integrated Memory Consolidation Processes Reconciled?
Despite different mechanisms and predictions, the hippocampo-neocortical and the synaptic homeostasis theories should not be seen as conflicting, as they more likely represent complementary phenomena in memory consolidation processes. For instance, increased hippocampal activity was observed using fMRI during the acquisition of an oculomotor sequence in fast-learning participants, who subsequently exhibited overnight improvement in performance, contrarily to slow-learning participants who did not initially engage hippocampal activity during learning and failed to improve performance overnight (Albouy et al. 2008). One can surmise that hippocampal tagging in fast learners actually reflects an initial homeostatic-like synaptic potentiation in hippocampal areas that might then trigger further processes possibly leading to the hippocampo-neocortical information transfer. Additionally, it must be mentioned that the role of REM sleep in the consolidation of recent memories is not considered much in these conceptualisations (although admittedly envisioned in the Buzsaki 1996 model), thus not entirely accounting for experimental evidence. For instance, combined EEG and fMRI data have yielded evidence for links between an overnight improvement of visual discrimination skills and the number of slow waves initiated in lateral occipital areas during post-training NREM sleep. This is paralleled by an association between the duration of REM sleep and increased oxygen consumption [i.e. higher Blood Oxygen Level Dependent (BOLD) response] at overnight retesting (Mascetti et al. 2013).
In this respect, the dual process (Plihal and Born 1997) and the sequential process (Giuditta 1984) hypotheses might more specifically acknowledge for the fact that both NREM and REM sleep stages are likely to contribute in the cascade of neuronal events eventually leading to memory consolidation. According to the dual process hypothesis, NREM sleep would be especially suited for the consolidation of declarative, hippocampus-dependent memories, whereas REM sleep would subtend the consolidation of procedural (hippocampus-independent) memories (Plihal and Born 1997, 1999). However, this marked distinction between declarative and procedural memory processes as a function of sleep stages is far to be supported by the existing literature, that relates the consolidation of information belonging to a wide range of memory domains (from verbal to motor material) with NREM sleep-related processes (see Sect. 4). Alternatively, the sequential hypothesis proposes that effective memory consolidation actually requires different steps of information processing during NREM then REM sleep episodes (Ficca et al. 2000; Giuditta 1984), for instance protection of recent memories against interference during NREM then their consolidation during REM sleep (Scrima 1982).
4 Brain Plasticity and Memory Consolidation During Sleep
The contribution of sleep to memory consolidation is now widely acknowledged in the literature. Animal studies have supported the hypothesis that post-training sleep activity recorded in learning-related brain areas might represent the neural signature of memory-related cognitive processes. For instance, cell recording studies in the rat hippocampus and cortex following exposure to a spatial environment (Kudrimoti et al. 1999; Lee and Wilson 2002; Louie and Wilson 2001; Nadasdy et al. 1999; Pavlides and Winson 1989; Wilson and Mcnaughton 1994) and in the song area of young zebra finches (e.g. Dave et al. 1998; Dave and Margoliash 2000; Margoliash 2001) revealed neuronal replay phenomenon during sleep, suggesting the reactivation of neural activity associated with previous experience.
4.1 REM Sleep and the Neuronal Replay of Procedural Memories
In humans, evidence supporting the neural replay hypothesis was initially found using H2 15O-PET. Brain areas activated during practice on a probabilistic serial reaction time (SRT) task, a form of implicit motor sequence learning (Cleeremans and McClelland 1991; Peigneux et al. 2000) were found more active during subsequent REM sleep in subjects previously trained on the task than in untrained subjects (Maquet et al. 2000; Fig. 4). In addition, rCBF in the left premotor cortex was correlated with activity in the pre-supplementary motor area and in the posterior parietal cortex involved in sequence learning, much more during post-training REM sleep than during REM sleep without a prior sequence learning experience (Laureys et al. 2001). This suggests that motor memory traces are replayed in the cortical network during REM sleep. Furthermore, reactivation in learning-related areas during REM sleep was specific to the reprocessing of the implicit rules driving the succession of the displayed locations in the sequence, more than to the mere optimization of basic visuomotor skills. Indeed, reactivation in learning-related areas during REM sleep was only found in subjects trained to the rules-based SRT task, but not in other participants trained on the same task but using a random material (Peigneux et al. 2003). These results indicate that the reactivation of local brain activity during REM sleep was related to the implicit acquisition of the probabilistic rules that defined stimulus sequences. Furthermore, learning-related cuneus activity during REM sleep also correlated with rCBF variations in the striatum, a key area in probabilistic sequence learning (Peigneux et al. 2000), significantly more in participants trained to the probabilistic than in those trained to the random SRT task (Fig. 4). Finally, the level of sequence learning achieved prior to sleep also correlated with increases in rCBF during REM sleep, suggesting that post-training cerebral reactivation is modulated by the strength of the memory traces developed during learning (Peigneux et al. 2003). None of these post-SRT learning effects was observed during NREM sleep. As a whole, these results support the hypothesis that REM sleep is involved in the reprocessing and optimization of the high-order, complex information contained in the material to be learned.
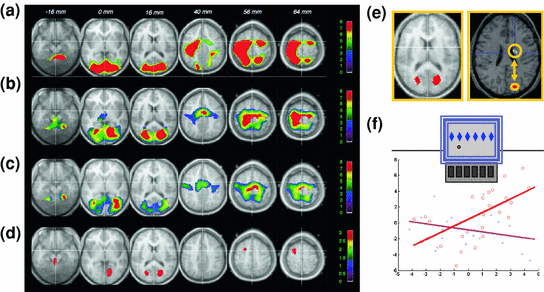
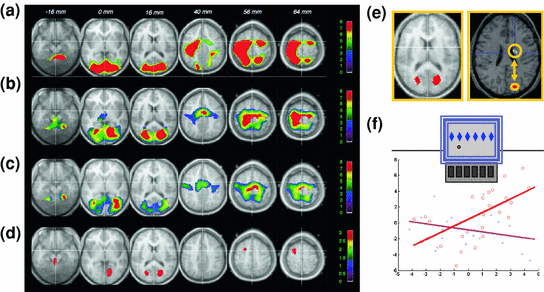
Fig. 4
Neuronal reactivation during REM sleep. a Brain areas where activity during active wakefulness is associated with learning on a motor procedural SRT task; b Brain areas active during REM sleep after learning on the SRT task; c Brain areas active during baseline REM sleep (no prior learning experience); d Brain areas where activity is significantly higher during REM sleep after SRT practice than during baseline REM sleep, and already active during learning; e Higher activity in cuneus during REM sleep after SRT practice than after practice of randomly presented stimuli; and f temporal correlations during post-training REM sleep between cuneus and striatum activity after practice on the probabilistic (red) but not on the random (blue) SRT. Adapted with permission from Maquet et al. 2000 and Peigneux et al. 2003
4.2 NREM Sleep and Reactivation of Hippocampus-Dependent Memories
Using this same probabilistic SRT task, no effects were observed during NREM sleep periods. This result is seemingly contradictory with animal findings that yielded evidence for neuronal reactivations during NREM sleep (but see e.g. Poe et al. 2000 and Louie and Wilson 2001 for experience-dependent changes in neuronal firing during REM sleep). One possibility for this apparent discrepancy is that learning complex sequential regularities in the probabilistic SRT task is dependent upon the activity of striato-cortical networks (Peigneux et al. 2000), whereas reactivations studies in rodents mostly used hippocampus-dependent spatial orientation tasks (e.g. Wilson and Mcnaughton 1994). In line with this interpretation, activity in hippocampal and medial temporal areas subtending navigation learning in a virtual town (i.e. a spatial, hippocampus-dependent learning task) was enhanced during subsequent NREM sleep, and mostly SWS (Peigneux et al. 2004), but not after training on the SRT task. In this PET study, hippocampal activity during post-training SWS was proportional to the overnight improvement in performance in the navigation task. These results suggest that learning-dependent modulation in hippocampal activity during human sleep actually reflects the offline processing of recent episodic and spatial memory traces, eventually leading to the plastic changes underlying subsequent improvements in performance. As a whole, PET studies indicate that distinct memory traces pertaining to different cerebral structures and memory systems, acquired under different learning conditions, are reactivated during subsequent and distinct sleep stages. Although these results seemingly concur with the dual process hypothesis positing that NREM sleep subtend consolidation of declarative, hippocampus-dependent memories whereas REM sleep subtend consolidation of procedural memories (e.g. Plihal and Born 1997, 1999), such conclusion should be taken thoughtfully. Indeed, the demonstration of segregated patterns of neuronal activity during different stages of sleep associated with the consolidation of different memory systems does not invalidate other interpretations. For instance, other neurophysiological mechanisms contributing to the memory consolidation processes might not have been detectable using the PET technique, limiting possible interpretations. Hence, these results do not allow rejecting alternative accounts, for instance, the sequential hypothesis (Giuditta 1984) assuming that effective memory consolidation requires information processing steps first during NREM then REM sleep episodes.
4.3 Neuronal Reactivations in the Light of Combined EEG/FMRI
Functional MRI also evidenced learning-related cerebral activation during sleep. After intensive training on a perceptual texture discrimination task, increased activity was observed during post-training SWS (versus the night before learning) in stimulated areas of the primary visual cortex (V1). Overnight improvements in behavioural performance additionally correlated with the amplitude of the BOLD signal in trained regions during SWS (Yotsumoto et al. 2009), in line with EEG findings of locally increased initiation of slow waves after training on visual orientation discrimination task (Mascetti et al. 2013). Similarly, sleep spindle-related reactivation after learning face-scene paired associates was observed in category-specific cortical areas during NREM sleep (Bergmann et al. 2012). In this EEG/fMRI study, learning face-scene associations triggered a stronger combined activation of neocortical and hippocampal regions during subsequent sleep, as compared to visuomotor practice. Also, reactivations were in temporal synchrony with spindle events and tuned by ongoing variations in spindle amplitude, but restricted to the face- and scene-selective visual cortical areas activated during pre-sleep learning. Finally, participant’s performance at the end of learning was correlated with spindle-coupled hippocampal activation (Fig. 5). These results can be also interpreted in the framework of a prior EEG study showing that fast spindles are driven by the depolarizing up state of neocortical slow oscillations and enhance the likelihood of succeeding slow oscillations together with slow spindles. As prior learning enhanced this pattern, it suggests that slow oscillation-spindle cycles and fast spindles contribute in sleep-dependent memory and consolidation processes (Molle et al. 2011). Altogether, spontaneous reactivation studies suggest the reprocessing of previously learned information during post-learning sleep, a reactivation possibly organized by sleep spindles and slow oscillations in the case of hippocampal-neocortical memories.
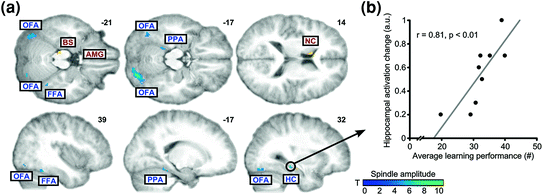
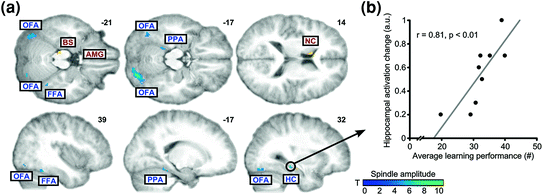
Fig. 5
Spindles, memory for face-scene associations and neuronal reactivation in the light of combined EEG-fMRI. a Brain areas showing learning-specific increases in coupling to spindle amplitude (cold colours) relative to control condition (BS brainstem; FFA fusiform face area; HC hippocampus; NC caudate nucleus; OFA occipital face area; PPA parahippocampal place area). b Correlation between learning performance and increased spindle-related hippocampal activity during subsequent sleep. Adapted with permission from Bergmann et al. 2012
4.4 Cueing Memories During Sleep
In a complementary approach, researchers have assumed that presenting learning-related cues during post-training sleep would trigger the reactivation of previously associated memory traces. Accordingly, non-awakening presentation during SWS of an odour associated with the learning of cards positions in the game “Memory” enhanced subsequent retrieval performance above levels achieved after a normal, undisturbed night of sleep (Rasch et al. 2007). Presentation of another odour was ineffective, demonstrating the specificity of the cueing effect. As well, presentation of the associated odour during REM sleep or wakefulness did not enhance performance. Functional MRI recordings additionally showed that cueing the associated odour during post-learning SWS triggered hippocampal BOLD responses, associated with performance improvement in the declarative memory task. Cueing-related consolidation of a finger-tapping motor procedural memory task was tested in the same study. At variance with the declarative paired association task however, re-presentation of the cue odour during SWS or REM sleep was ineffective to improve memory. This result is discrepant with other data suggesting that presentation of cues coincidently with REMs actually boost procedural learning. For instance, prior studies (Guerrien et al. 1989; Smith and Weeden 1990) found that cueing during post-training REM sleep using auditory stimulations delivered during learning improves behavioural performance when tested on the next day. Since odour pathways directly link to the hippocampus, it is possible that odour stimulation is not appropriate to trigger non hippocampus-dependent procedural memories, and that other sensory modalities should be exploited. Accordingly, another behavioural/EEG study trained participants to play two melodies on a keyboard. Auditory presentation of one of the two melodies during a post-learning subsequent nap resulted in higher performance improvement for the sleep-replayed than for the other melody (Antony et al. 2012), suggesting that auditory cueing during sleep is beneficial for sensorimotor memories to the same extent than odour cueing for non-declarative memories. However, auditory stimulations were delivered during NREM sleep again in this latter study, leaving under discussion the possibility to trigger memory consolidation processes during REM sleep. Notwithstanding, other studies confirmed that sounds are as effective as odours to trigger memory consolidation processes. In an EEG study (Rudoy et al. 2009), participants learned the location of different objects, each object being associated with a congruent sound (e.g. a bark associated with the image of a dog). During the subsequent nap, half of the object-associated sounds were delivered. Recall was higher for the cued than the non-cued images, again suggesting specificity in the triggered reactivations and in the ensuing consolidation processes. Similarly, participants were scanned during SWS using fMRI while presented the object-associated auditory cues (van Dongen et al. 2012). Although memory performance was similar in the experimental and control conditions, evoked BOLD responses in the right parahippocampal area were higher during SWS for the cued than for the control sounds. Furthermore, the connectivity between the parahippocampal and the posterior visual areas increased upon presentation of the cue sounds. According to the authors, this suggests that cue-related evoked responses during sleep are not merely limited to the processing of auditory stimulations, but actually generalize to the visual areas recruited during the task. This effect is congruent with the report of spindle-related reactivation of paired associates both in hippocampal and task-specific face or locations-related areas (Bergmann et al. 2012).
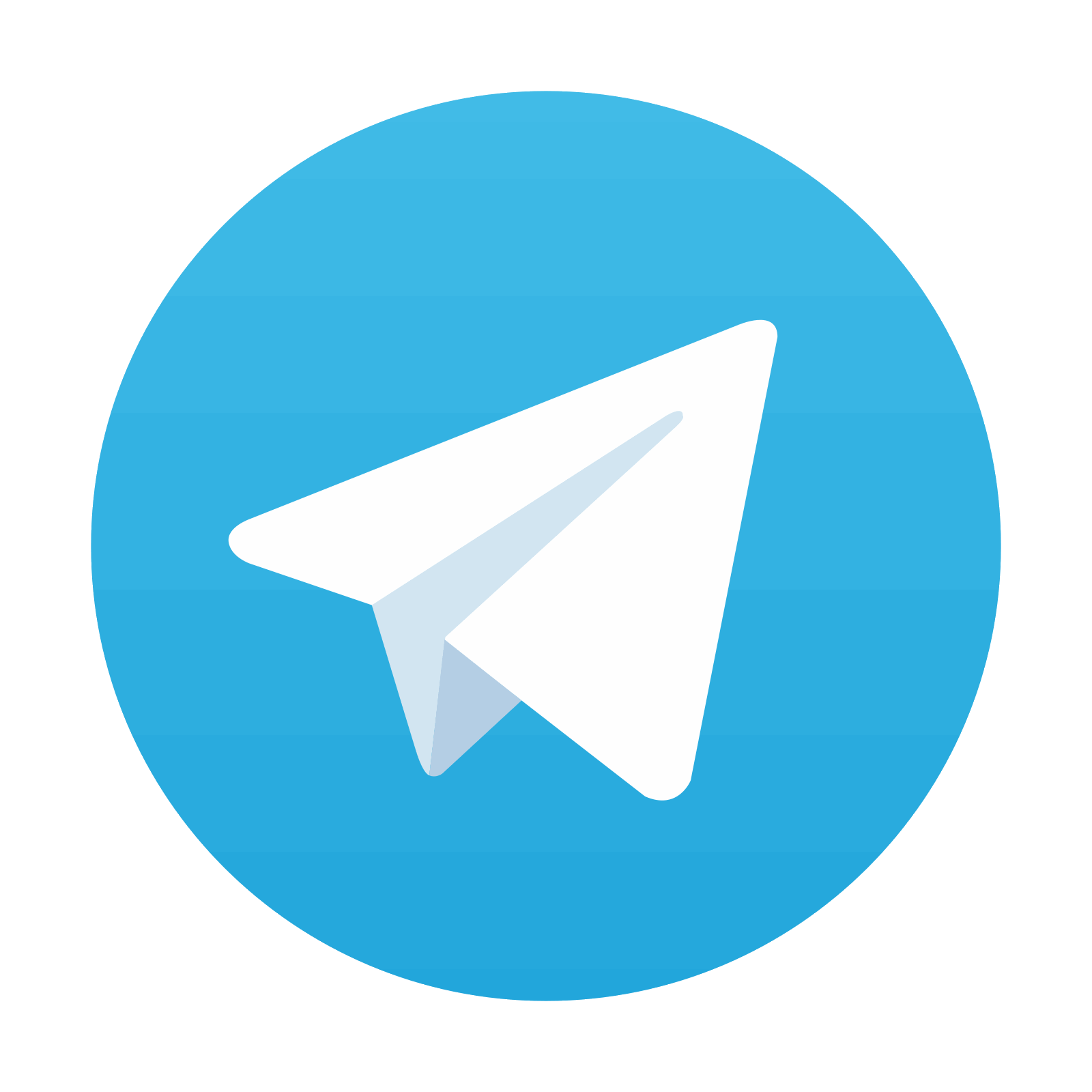
Stay updated, free articles. Join our Telegram channel

Full access? Get Clinical Tree
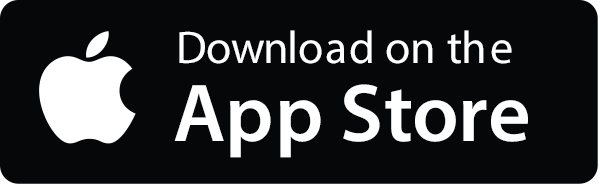
