Fig. 15.1
Overview of analytical approaches in clinical research
Observational Studies
The value of case reports has been the subject of considerable debate among clinical researchers. Primarily, this negative attitude comes from a preponderance of cases that provide limited insight into the nature of the disease entity under investigation; these vignettes are simply “me too” reports of well-studied clinical conditions for which a minor variation in presentation or outcome is of some educational interest. On the other hand, documentation of a single case of an entirely novel clinical condition ultimately may lead to studies yielding potentially valuable insights into epidemiologic issues related to this condition. These could include both numerical estimates of the prevalence or incidence of the condition and its association with exposures from the environment, pathophysiological or pharmacologic risks, and infectious agents.
Hierarchically, the next category of observational studies is the case series. As the name implies, this group of studies presents detailed accounts of multiple cases (typically three or more) of a given condition. For epidemiological utility, the cases within the series usually have some degree of commonality beyond the clinical outcome. Thus, in their entirety, they present a cohesive group of entities which are, not only, related to outcome but by the presence of one or more potentially common exposures. Of course, the great danger in attempting to relate these exposures to the outcomes presented by these cases is the temptation to attribute the status of association or, worse yet, of causality to these observations. However, careful documentation of a series of related cases can be valuable in identifying epidemiological factors related to the disease under investigation. Thus, a carefully presented case series is an important first step in the analytical study of diseases because of its ability to generate one or more hypotheses, which can be tested in a formal, analytical manner. This leads to the next group of observational designs, the hypothesis testing or analytical studies. In this group of observational studies, formal statistical approaches are used to glean information on the occurrence and on the associations of the outcomes with exposures that might ultimately be found to be risk factors for a given outcome.
The most basic of the analytical designs is the case–control study (Fig. 15.2). In this model, the individuals with a given clinical outcome (symptom, disease, or syndrome), i.e., cases, are compared in some manner with a control group of usually healthy individuals or individuals not exhibiting any symptomatology similar to the case group. Thus, the comparison is made between the two groups and subject inclusion in either group is based simply on which group the individual belongs. Once the groups have been delineated, the investigator examines such issue differences in numerical occurrence between the groups (incidence or prevalence), quantitative differences between observed exposures or putative risk factors, and associations between these exposures and the occurrence of disease. Lam et al. [1] effectively used this design to examine association of daytime sleepiness and restless legs syndrome with type 2 myotonic dystrophy (DM2).


Fig. 15.2
Basic flow chart for the case–control study. Assignment to group is based on the presence (cases) or absence (controls) of the clinical entity of interest
Most case–control studies are retrospective, but this is not an absolute: Subjects can be recruited into the assigned groups in a prospective fashion. Case–control studies have the advantage of being able to study relatively rare conditions in which the numbers of cases are small. To increase the statistical power of the study (more on this, later), the number of controls is often increased from two to ten times the number of cases. For example, in the previously cited paper [1], there were 54 DM2 subjects enrolled in the study, but these patients were compared with a control group of 104 individuals drawn from the general clinic population of the authors’ institution.
Case–control studies, especially retrospective ones, however, have several drawbacks. The degree of risk imposed by a given exposure is difficult to ascertain. In fact, there is no way of determining whether a control will eventually exhibit symptoms suggesting of the disease state present in the cases. Moreover, the primary patient record, which is surveyed for the pertinent data, may not have included relevant information—or may have large amounts of data excluded. Therefore, in case–control studies, the odds ratio (OR) rather than relative risk (or risk ratio, RR) is used to provide an estimate of the magnitude of effect. Another drawback of case–control studies is the difficulty in obtaining longitudinal data; the data obtained from most case–control studies is cross–sectional, based on observations of simultaneously occurring events such as outcomes and exposures. All these factors lead to the consideration of the case–control study as a relatively weak design from the point of view of strength of medical evidence. The aforementioned technique of increasing the size of the control group (or having a large number of cases) and the use of matching for age, gender, or other potential confounders can increase the evidentiary value of the study.
A far stronger and more versatile group of studies are the cohort studies (Fig. 15.3). These start with a group of individuals with a common attribute or clinical finding. For example, the cohort might be drawn from the general public or some subset of the general public. This would be considered to be a population–based cohort study such as that conducted by Lai et al. [2] on the risk of type 2 diabetes mellitus (DM type 2) in subjects with non-apnea sleep disorders. In this study, the cohort was drawn from a large health insurance database of Taiwanese subjects. Another possibility would be to select an at-risk cohort sharing some common clinical entity, e.g., congestive heart failure, DM type 2, or obstructive sleep apnea. Cleator et al. [3] developed a cohort based on severe obesity (BMI > 40 kg/m2) in a group of British subjects. They examined point prevalence and differences in characteristics such as age, gender and data from several questionnaire-based instruments (e.g., the Pittsburgh Sleep Quality Index, the Epworth Sleepiness Score, and the night eating questionnaire). In either type of cohort study, the cohort can be divided into two or more comparative groups based on some exposure including genotypic or phenotypic biomarkers, (Fig. 15.3a) or by the presence or absence of some additional symptomatology or comorbidity (Fig. 15.3b). The latter study is referred to as a cohort–nested (or just, “nested”) case–control study.


Fig. 15.3
Flow charts for cohort studies. a The cohort is bifurcated into subjects with and subjects without a putative risk factor (exposure). b The cohort is divided based on the presence or absence of a given outcome or symptomatology. This is referred to as cohort-nested case–control study
Cohort studies may be either retrospective or prospective and have several advantages over case–control studies. Cohort studies, as noted above, offer a degree of versatility difficult to obtain in case–control studies. They may, for example, contain several arms, i.e., essentially substudies examining more than one facet of the cohort. They also provide longitudinal data in a far more facile manner than case–control studies. Also, in the case of prospective cohort studies, potential biases can be negated by careful planning and early consideration of confounding factors. An important thing to remember in the case of all observational studies is that association, except in several very rare cases, never implies causality. Cause-and-effect is virtually always the domain of the interventional study. This leads to an examination of that group of studies.
Interventional Studies
Among interventional studies, as noted in Fig. 15.1, we consider several designs, experimental and quasi–experimental. In both types, the investigator provides one or more independent variables, almost always related to treatment (the intervention or interventions) and examines one or more dependent variables, related to outcomes.
In medical disciplines, the experimental design is typified by the randomized controlled trial (RCT). As the name implies, the subjects are randomly assigned to groups. The manner in which they are randomized may vary from simple randomization methods, requiring nothing more than a coin toss (although assignment in modern studies is based not on the heads or tails result of a flipped coin but rather on the use of a computer-generated random number) to more complex randomization schemes. These include several variations of randomization in blocks of various sizes used to avoid unequal distributions of one or more potential confounders, variables which might introduce a bias into the study.
Another issue in the RCT is the comparison group. As noted above, by virtual definition, the group receiving the active intervention will be compared with the control group. In the past, the expectation in examining most RCTs was that the control group would be given a placebo, essentially a mimic of the active treatment that is expected to lack any activity, in and of itself. However, with the plethora of treatment options available today, the use of a placebo renders the ethical use of an expectedly ineffective placebo ethically dubious, particularly when effective therapy is available to clinicians. Thus, a technique that is used in many modern studies is to compare the experimental intervention with a therapy of established efficacy. Chihara et al. [4] randomized ninety-three subjects to three groups: Auto-adjusting positive airway pressure (APAP) was applied to one group, and APAP with pressure flexing devices (A-Flex and C-Flex) were provided to the other two groups.
Occasionally, evaluations are often performed initially with a non–inferiority study such as the study by White and Shafazand [5] which examined the hypothesis that treatment with a mandibular advancement device is just as effective as continuous positive airway pressure). As the name implies, the object of this type of study is to establish that the experimental treatment performs no poorer than the treatment with established efficacy by a level known as the non–inferiority margin [6]. As will be discussed further in this chapter, the statistical approach used in the analysis of non-inferiority trials is somewhat different than a “head-to-head” trial in that the investigators, a priori, account only for the possibility that the experimental maneuver will be no worse than the comparator or control modality.
At this point, several additional aspects of the RCT should be mentioned. Investigators are often faced with the need to control for differences in dosing schedule or route of administration between the experimental intervention and the comparator. In this case, the need to include placebos for both differences becomes apparent. This maneuver, called a double–dummy, is frequently employed in the RCT and is exemplified by the study by Valente et al. [7] comparing sublingual and oral Zolpidem to initiate sleep onset in healthy subjects.
In addition to the RCT, other interventional models are occasionally used in interventional studies. Foremost among these are quasi–experimental studies, in which the subjects are not randomized [8]. One of the most commonly used quasi-experimental approaches is the pre– and post-model, in which the individual essentially acts as his or her own control. Such a study was conducted by Wei et al. [9] to determine the value of implanting blue-light blocking intraocular lenses in improvement of the quality of sleep in cataract patients. It should be recognized that in the absence of an external control, differences in the dependent variable before treatment and after treatment may be solely a function of some unknown confounder, e.g., time. Thus, a study such as this can be strengthened by the addition of a control group in which the difference in the dependent variable is also measured.
In terms of analyzing the result of an interventional study, the research team needs to determine whether only subjects who completed the study, as designed, should be included in the data analysis—or whether all subjects initially enrolled should be included. The former analytical approach is called per protocol analysis, and the latter, intention-to-treat (ITT) analysis. In that, it gives a better gauge of the overall utility of a given treatment, and most studies, today, use ITT as the primary approach to analysis.
Factors Involved in Determining Validity
Validity, in essence, deals with the correctness of conclusions drawn from an experiment. Various facets (construct validity, content validity, face validity, and predictive validity) can be examined separately; however, in most sleep studies (as is the case with most examples of clinical research), two main components of validity are examined: internal validity and external validity.
When designing a study, invariably a group or groups of subjects are examined in terms of their response (a dependent variable) to an intervention (an independent variable) or the association or differences of some outcome variable with respect to some exposure or predictor variable (more on this further in this chapter). The first issue relating to the process of relating dependent variables or outcomes to independent variables or exposures is the correctness of the process, i.e., getting the right answer about the individuals that the researchers, i.e., studied. This is internal validity. However, the ultimate goal is to determine whether that answer is applicable to the population which is represented by the sample used in the research. The ability to generalize from the sample to the population is external validity. In order to achieve external validity, the investigators must, first and foremost, apply appropriate inclusion and exclusion criteria to the process of subject recruitment. Simply put, the sample must be representative of the population with which the study deals. Clearly, then, the sample should have the demographic and clinical characteristics of the population of interest (inclusion criteria) and avoid subjects with demographic or clinical characteristics that render them different (exclusion criteria) from the population of interest.
Basics of Statistical Analysis in Clinical Research
Fundamentally, the application of statistical methods in clinical research serves two purposes: first, to compare the result of an observation or experiment with that result which would occur purely by chance, and second, to determine the magnitude of the observed effect so that the clinician can interpret just how useful the information garnered from a study might be to the provider as well as the patient. This section will review the basic steps that are taken in the analysis of different types of data emanating from research studies.
Variables in Clinical Studies
The data from clinical studies can be categorized as variables in terms of both how the investigator obtained it (a relational description) and the kind of information it provides (a functional description). In the first case, the question asked is “did the observer provide, as part of the protocol, the variable in question or was that variable the expected result of the experiment?” If the former, the variable is an independent variable; if the latter, it is a dependent variable. It is easy to see how this applies in an interventional study: The intervention is the independent variable and the result, as measured, is the dependent variable, but what of observational studies, in which the investigator does not apply the intervention? In this case, one may reasonably consider the construct in which the study exists as an experiment of nature. In other words, the experiment has already occurred through genetic, pathophysiologic, nutritional, or other epidemiological forces. Thus, the role of the researcher is to simply analyze the result, in terms of some independent variable (as noted above, the exposure), and a dependent variable, the outcome.
Variables are also described in terms of their functionality as measurements. This is often called the levels of measurement. A convenient approach is to consider three broad categories of data: quantitative, qualitative, and ordinal. In the former, two subtypes are included: interval and ratio data. Interval data are those which provide numerical values with a continuum that lacks a set zero value or for which a zero value is arbitrary. Temperature scales other than absolute are examples of this type of data. Ratio data have a true zero value and numbers in a ratio data set are referenced directly to that zero value. So, temperatures from the Celsius or Fahrenheit scale being interval in nature and not referenced to a non-arbitrary zero point are interval in nature: 40 °C is not half as warm as 80 °C. On the other hand, a serum analyte, e.g., glucose of 200 mg/dl is, indeed, twice the actual concentration of a serum glucose level of 100 mg/dl. These are ratio data because they can be referenced to a theoretical level of 0 mg/dl. Another set of descriptors used for quantitative data (not included in Fig. 15.3) is continuous and discreet. Continuous data are those which are infinitely divisible, limited only by our instruments of measurement. Discreet data are indivisible: They are either naturally discreet (number of siblings or copies of an amplified gene) or conveniently discreet such as age, most often recorded to the completed year of life. For all of the aforementioned quantitative data, descriptive statistics reasonably include properties of their distribution including measures of the central tendency (mean, median, and mode) and measures of dispersion (“variability,” to be discussed later in this chapter).
Quantitative data are easily recognized in that rather than having numerical values, they have names, i.e., are nominal or categorical. They can range from dichotomous (dead, alive, or exposure present/absent) to categories with multiple descriptive choices such as race and ethnicity. In describing these data, only a number of subjects with the quality apply.
Ordinal data represent a sort of hybrid: They are described with numbers but the numerical values are purely representative of degree rather than any true mathematical relationship. Several types of ordinal data are encountered in clinical studies. Most commonly used are the visual analog scale (VAS) and the Likert scale. In a VAS, the numerical values represent the intensity of a measurement on a mathematical continuum and, as conceptually analog, can be subdivided to some degree. An example of a VAS is the commonly used clinical pain scale which ranges from 0 (no pain) to 10 (worst pain imaginable). In this scale, being conceptually analog, a patient’s response such as “5.5” would be acceptable (however, would ordinarily be given a value of 5 or 6). In a Likert scale, commonly used in survey instruments such as questionnaires, an item is generally given a numerical value indicating a property such as frequency, with “never” being assigned a value of 0 and “always” having a value of 5. Likert items are virtually always treated as discreet values. It is easy to recognize that ordinal numbers are purely representative and arbitrary. An individual who describes his or her pain as an “8” does not necessarily have twice as much pain as an individual whose pain is described as “4.” This being the case, we treat the numerical values emanating from an ordinal scale differently than we do true quantitative data. In terms of descriptive statistics, the central tendency of an ordinal data set is best described by the median and the dispersion by some measure of range, such as minimum–maximum or interquartile range (IQR), the 25th to 75th percentile of values.
There are also clinical scales, such as the Acute Physiology and Chronic Health Evaluation (APACHE II) and the Pneumonia Severity Index (PSI) which are based on the totality of a group of clinical signs and symptoms. There is no consensus as to whether these should be treated as discreet interval data or ordinal data; however, the wide range of values attributable to these scales suggests that there is an inherent mathematical relationship among scores from these clinical scales. Moreover, global scores from questionnaires utilizing Likert items are frequently treated as interval data.
Descriptive Statistics
That branch of biostatistics that examines how values of a variable are distributed is called descriptive statistics. In the case of qualitative data, descriptive statistics, as previously noted, is limited simply to counts, i.e., number of subjects exhibiting a particular quality. Of course, a necessary outcome of that description, albeit secondary, is the proportion (percentage or percent) of subjects with that quality.
Quantitative data, however, are examined in terms of how they are distributed. They are described in terms of their central tendencies and dispersion. Precisely, how they are distributed generally determines the choice of central tendency and unit(s) of dispersion.
It is extremely important to recognize whether a set of quantitative data is distributed normally (described by a Gaussian distribution). The reason for this is because when inferential statistical methods (collectively, those statistical tests which provide information about how likely or unlikely what we have observed has occurred purely by chance) are applied, the nature of the distribution will bear on this choice in nearly all cases.
The normal distribution is easily recognized as the classic “bell curve,” i.e., it has two “tails” set about a central tendency. Examples of this distribution abound in the literature and can easily be located in elementary statistics texts and on the Internet. The tails are symmetrical, that is they lack skewness and are neither too peaked nor too flat (the degree of “peakedness” or flatness is called kurtosis). The range of values between the points of inflection on both the rising and falling sides of the distribution curve is equivalent to roughly 67 % of all the values in the data set. This is ±1 standard deviation (SD). Taking twice this value, 2 SD, yields approximately 95 % of the values and, at 3 SD, over 99 % of the values are accounted for. For data which are normally distributed, the branch of inferential statistical tests known as parametric (because they are based on the actual value of the parameter or variable) methods can be applied. With the exception of extremely large data sets for which the choice is unnecessary, those data sets that are not normally distributed should be subjected to nonparametric tests, which are generally based on rank order of the parameter rather than the parameter itself. Ordinal data are always treated with nonparametric methods, as the parameter is essentially meaningless from a mathematical standpoint.
The question then arises, how is one to determine whether a data set is normally distributed or at least approximates a normal distribution well enough to warrant the application of parametric tests? Simply graphing the values as a differential distribution plot (number or percentage of subjects vs. the incremental value of the variable), in most cases, requires a very large sample to visually observe a normal distribution. This conundrum has been addressed many times in the statistics literature, and the approach most often taken is to apply one of the more commonly used “normality tests,” collectively, a group of statistical maneuvers designed to determine how much the distribution of a variable differs from a normal distribution. Some of the most common tests are based on the comparison of a simulated distribution best fit to a normal distribution with the actual distribution of the data. A good example of this is the Lilliefors (Kolmogorov–Smirnov) test, which dates back to 1967 [10]. The normality tests developed by D’Agostino and Pearson [11] take a different approach, in which a parameter, K 2, summarizes the skewness and kurtosis of the distribution. Thus, the fit to normality is based on the actual shape of the distribution.
At this point, it might be wise to recognize that remarkably few physiologic parameters distribute normally. In many cases, a log–normal distribution is observed, in which case the distribution exhibits considerable skew to the right, i.e., toward increasing values of the variable. In this case, simply transforming the data by taking the log of the variable will provide a reasonable fit to normality. Another approach is the power–normal or Box–Cox transformation [12] that will occasionally provide a better fit than a simple log transformation. In Fig. 15.4, a large database of over 44,000 serum total cholesterol samples is depicted prior to transformation (Fig. 15.4a) and after log (Fig. 15.4b) and Box-Cox (Fig. 15.4c) transformations. In Fig. 15.4d, the Box-Cox transformed data distribution is compared with the best-fitted Gaussian distribution. The fit to normality is extremely strong, as demonstrated by the R 2 value of 0.998 (more on the “R 2” later in this chapter).
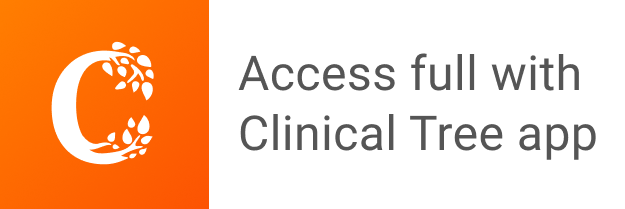