The Neurophysiology of Voluntary Movement in Nonhuman Primates: Accumulator Models of Decision and Action in Relation to Psychogenic Movement Disorders
Steven P. Wise
Jerald D. Kralik
ABSTRACT
According to some recent models of decision, choice, and action, distinct neural networks accumulate evidence in favor of making a potential movement. Voluntary movements arise when one of these “accumulator networks” reaches its threshold for producing an output. Sluggish operations in these networks could prevent an intended movement or make one difficult. Hyperactive accumulators might generate unintended movements. Other networks operate through similar accumulator mechanisms to “veto” movements, and hyperactive operations in these networks could also prevent intended movements. Taken together with the idea that people might be aware of some of the “evidence” for making or vetoing a movement, but not all of it, these models provide useful insights into psychogenic movement disorders.
INTRODUCTION
“Accumulator-racetrack models” of decision and choice have gained support from recent neurophysiologic experiments in monkeys. According to these models, voluntary movements occur when certain premotor neural networks reach a threshold for producing an output. These networks, which we call “commanding accumulators,” act like leaky integrators, gathering evidence in favor of the action that they command. Their output leads to a cascade of events that ends in the generation of a movement, either by triggering activity in motor pattern generators or releasing them from tonic inhibition. Commanding accumulators work in parallel and in competition with each other, with the one (or ones) reaching threshold first, controlling movement in a winner-take-all manner.
Any pathology that undermines the ability of these networks to reach their threshold could either prevent an intended movement entirely or require that the accumulators receive unusually large or abnormally sustained inputs to reach their threshold. The former possibility might be relevant to psychogenic paralysis, the latter to psychogenic weakness. A different kind of accumulator network has outputs that serve to prevent or “veto” movements. Undesired or uncontrolled output from these networks, which we call “countermanding accumulators,” could also lead to negative psychogenic movement disorders such as paralysis or weakness.
Conversely, a pathologic increase in the output of commanding accumulators, the failure of countermanding accumulators, or some dysfunction of the winner-take-all mechanism might lead to unintended movements, as in positive psychogenic movement disorders such as dystonia, tremor, or myoclonus.
This chapter presents these concepts and then develops them in the context of two ideas about the functional organization of primate brains: (i) Primate brains have separate neural systems for perception and action, and (ii) their prefrontal cortex mediates a form of behavioral inhibition. The first idea holds that many apparently voluntary actions are actually controlled subconsciously by automatic sensorimotor transforms called “autopilot mechanisms.” The second holds, by analogy, that the process of vetoing or suppressing movements may also proceed without conscious mediation. Our goal is to provide a conceptual “toolbox” for thinking about the pathophysiology of psychogenic movement disorders and discussing their potential causes with patients and family members. (Some readers may choose to pass over most of the next section, which deals with philosophic and conceptual issues that arise in studying voluntary movements in monkeys or other nonhuman animals. For such readers, the subsection entitled “Terminology,” which defines the terms used here, picks up the presentation from this point.)
WHAT IS A VOLUNTARY MOVEMENT AND DO MONKEYS MAKE ANY?
Are Studies in Monkeys Relevant to Psychogenic Motor Disorders?
Monkeys never appear in a clinic complaining of a psychogenic movement disorder; they neither claim nor deny making voluntary movements. So one might reasonably doubt the relevance of behavioral neurophysiology, as studied in monkeys, to disorders of voluntary movement.
Fundamental biologic principles suggest, however, that the insights gained from behavioral neurophysiology in monkeys have some bearing on understanding voluntary movements in humans. We share with monkeys approximately 500 million of our 530 million years of vertebrate history. From the inception of vertebrates, our common ancestors made movements based on decisions about what to do, when to do it, and whether it should be done at all. The vertebrate brain may have evolved specifically for this function (1). Much later, 55 to 65 million years ago, our most distant primate ancestors built on that heritage by evolving mechanisms for grasping fruit, nectar, and leaves from the distal end of branches (2). These “aboreal graspers” eventually gave rise to monkeys, apes, and humans. New World monkeys—which include three kinds of monkeys that will be mentioned in this chapter: cottontop tamarins, squirrel monkeys, and common marmosets—went their separate way from the ape-human lineage approximately 35 million years ago. Another group of monkeys, Old World monkeys, includes the mainstay of behavioral neurophysiology, the rhesus monkey. Old World monkeys diverged from the ape-human lineage about 30 million years ago. That lengthy period saw the evolution of upright posture, bipedal gait, language, and extensive tool use. So a lot happened in those 30 to 35 million years. Nevertheless, the ape-human lineage inherited many of the mechanisms for choosing and guiding actions that both we and monkeys retain. Still, our separate history and the taciturn nature of our fellow primates raises questions about whether monkeys make voluntary movements in the same sense that we do—or, at least, in the same sense that we think we do (3).
What Is a Voluntary Movement?
In his seminal monograph on The Frontal Lobes and Voluntary Action, Passingham (4) defined voluntary movements as actions that are learned, attended, and based on a comparison among alternatives. We will use his definition in this chapter, but not without explicitly recognizing its problems.
The biggest problem is that for people, we usually accept a symbolic report—usually, but not necessarily, verbal—about whether a movement is voluntary. Alternatively, we
see that a person does as he or she is instructed, again usually verbally. So how could anyone know if a monkey, which cannot make such reports or comprehend verbal instructions, is making a voluntary movement? Using Passingham’s definition solves the problem operationally but not satisfyingly. To appreciate the source of this dissatisfaction, imagine that a person sees some “thing”—so far away that it could be either a person or a robot—reach to an object. On the assumption that the “thing” is a person, most people would suppose that he or she had just made a conscious decision to reach to the object and was, therefore, making a voluntary movement. On the assumption that the “thing” is a robot, however, most people would be reluctant to draw the same conclusion. They might imagine that the robot guides those movements through a complex computation of sensorimotor transforms, but they would not think that the robot had made a conscious decision, and they would not consider it to have made a voluntary movement.
see that a person does as he or she is instructed, again usually verbally. So how could anyone know if a monkey, which cannot make such reports or comprehend verbal instructions, is making a voluntary movement? Using Passingham’s definition solves the problem operationally but not satisfyingly. To appreciate the source of this dissatisfaction, imagine that a person sees some “thing”—so far away that it could be either a person or a robot—reach to an object. On the assumption that the “thing” is a person, most people would suppose that he or she had just made a conscious decision to reach to the object and was, therefore, making a voluntary movement. On the assumption that the “thing” is a robot, however, most people would be reluctant to draw the same conclusion. They might imagine that the robot guides those movements through a complex computation of sensorimotor transforms, but they would not think that the robot had made a conscious decision, and they would not consider it to have made a voluntary movement.
But what if the person made a movement in a “robotlike” way, using “autopilot” control mechanisms divorced from conscious awareness? As discussed later in this chapter and by Zeman (Chapter 24), people perform many complex, purposive behaviors without awareness, including sleepwalking, reaching to objects in “blindsight” (5), and performing sequences of movement (6). These actions look just like voluntary movements. So how could anyone verify the voluntary nature of a monkey’s movement?
The answer is that it cannot be done, at least not for certain. The problem is, of course, that of animal consciousness. To say that expert opinion remains divided on the topic of animal consciousness is to say the least. There are books in which the author of one chapter obsesses about whether chimpanzees have anything remote like human consciousness, while the author of another chapter discusses his or her studies of conscious knowledge in rats without the slightest hint that the issue might be controversial. Without a verbal or symbolic report, there is no straightforward way of telling whether a movement is voluntary in the sense used in ordinary conversation. For this reason and others—some of which stem from the continuing legacy of radical behaviorism in contemporary neuroscience—many neuroscientists are “afraid to discuss” topics related to consciousness, including voluntary movement (Chapter 22). Indeed, most discussions of knowledge and goal-oriented action in nonhuman animals keep the problem of consciousness securely in the closet and use a broad array of proxy terms instead. Conscious knowledge is called “declarative” when applied to memory systems or “explicit” when applied to tasks. By exclusion, information that has no access to conscious awareness is often termed implicit or procedural. Some psychologists use the term “habit” interchangeably for procedural knowledge, even though they often do so in a way that is inconsistent with the concept as defined in formal animal learning theory (7). Unfortunately, the evidence for explicit knowledge in nonhuman primates is, as yet, inconclusive (8). Despite more than a decade of research into imitation, ape “language,” deception, theory of mind, and related issues, opinion remains divided on whether nonhuman primates, in general, or monkeys, in particular, show any of these traits. So while we can accept Passingham’s (4) definition of voluntary action for the purposes of discussion, the problem of animal consciousness needs to be acknowledged, and, for the present purposes, this means being as explicit as possible (no pun intended) about the terminology used here.
Terminology
We have defined the “voluntary” part of voluntary movement above, following Passingham (4), subject to the inherent limitations on speculating about the conscious life of monkeys. The “voluntary” part implies a movement that is learned, attended, and based on an evaluation of alternative actions and their potential outcomes. Involuntary movements are thus defined, by exclusion, as movements that are either unattended (and are thus on “autopilot” control), do not have to be learned or are learned virtually automatically (and are thus “prepotent responses”), or require no evaluation of potential outcomes (and are thus “habits”). The “movement” part of voluntary movement also deserves some elaboration. We usually think of voluntary movement as a positive act, depending on muscle activity. However, deciding to do something inevitably means deciding not to do many other things. Not only does decision-making require the selection of one voluntary movement instead of others, but it also requires the inhibition of competing involuntary actions. And the decision not to do something—or anything—may be as voluntary as the decision to make a movement. So for the purposes of this chapter we construe “movement” very broadly to include stable posture and, therefore, the withholding, suppression, or vetoing of movement. And we also use the term “perception” in a particular way in this chapter, which differs from common usage. We restrict use of the term perception to instances of conscious awareness and do not use it as a synonym for sensation or sensory input, as is often the case.
THE NEUROPHYSIOLOGY OF DECISIONS: ACCUMULATOR-RACETRACK MODELS
Accumulator-Racetrack Models in Theory
Although studies of cognition in nonhuman primates and other animals to date have not resolved the question of whether they have explicit knowledge, awareness, or consciousness, their capacity for expectation and prediction is not in question. Accordingly, animal learning theory—which depends on the concepts of expectation and prediction—has contributed importantly to the understanding of voluntary
behavior (although many of its practitioners would be reluctant to call it that). In animal learning theory, voluntary action is considered to result from a “decision-making” process. Animals appear to make responses based on a choice among a (large) set of possible responses, and this decision appears to be based on a comparison of the expected outcomes, in terms of biologic value, of alternative actions. The assessed value of each response alternative can be quantified, including the relative influence of variables such as reinforcement rate, reward magnitude, certainty, effort, and delay. These ideas have recently been successfully applied to behavioral neurophysiology in monkeys (9,10). In this section, therefore, we describe some key results and ideas coming from this work, and, in the final section of this chapter, we attempt to place these ideas in the context of psychogenic movement disorders.
behavior (although many of its practitioners would be reluctant to call it that). In animal learning theory, voluntary action is considered to result from a “decision-making” process. Animals appear to make responses based on a choice among a (large) set of possible responses, and this decision appears to be based on a comparison of the expected outcomes, in terms of biologic value, of alternative actions. The assessed value of each response alternative can be quantified, including the relative influence of variables such as reinforcement rate, reward magnitude, certainty, effort, and delay. These ideas have recently been successfully applied to behavioral neurophysiology in monkeys (9,10). In this section, therefore, we describe some key results and ideas coming from this work, and, in the final section of this chapter, we attempt to place these ideas in the context of psychogenic movement disorders.
Significant progress has been made toward understanding the neurophysiology of decisions that monkeys make about the stimuli they see and the actions that they perform. Recent findings indicate that neural networks called accumulators, which act something like leaky integrators, gather evidence about the world or gather evidence for making a given response. Because both of these processes involve accumulating information, these theories of decision have been termed accumulator models. In some accumulator models, competing accumulators generate different decisions, and the first accumulator to reach a threshold for producing its output “wins.” For that reason such models are also called racetrack models. To capture both ideas, we call them accumulator-racetrack models. (In other versions, the accumulator that gets “far enough” ahead of the others wins, or a given accumulator weighs both the evidence for and against a particular decision.)
Accumulator-racetrack models posit that neural integrators accumulate evidence; if this evidence eventually reaches a threshold, the accumulators generate a decision. The evidence is usually considered to be of a sensory nature, but these models can be extended to include internally generated signals, as well. Decisions can relate to the nature of the sensory evidence alone, as in sensory perception, or to a choice among potential responses, as in voluntary movement. Motor decisions can be both positive and negative: They can lead to a chosen movement or to the inhibition of unchosen ones. Typically, accumulator-racetrack models divide decisions and choices into phases, as illustrated in Figure 23.1, with the early phases dealing primarily with the evaluation of sensory evidence, and later phases, usually thought to overlap in time with the earlier ones, dealing with decisions about and choices of potential actions (11). According to these models, the central nervous system makes decisions at both stages: first deciding what the sensory inputs represent and somewhat later choosing what action to perform.
The model illustrated in Figure 23.1 has a “front end” component that corresponds to the several related concepts that are usually subsumed by the term “attention” (11). Consider a task in which someone must respond to a number, presented on a screen, by saying whether it is odd or even. In the model depicted in Figure 23.1, a bias parameter (also known as a response set) might push decisions toward a response in terms of “odd versus even” numbers, as opposed to other response rules or “sets,” such as “prime versus nonprime,” “larger versus smaller” than 5, and so forth. Another attentional factor involves which features are extracted from the environment. And a third attentional factor is a priority parameter, sometimes called a stimulus set.
For example, imagine that someone needs to say whether a number is odd or even, but there are two odd numbers on a screen, one above the other. According to the model in Figure 23.1, a priority parameter predisposes the system to assign its response, oddity, to the top number, for example. Together, these attentional factors modulate the influence of evidence on the accumulators involved in decisions and choices.
For example, imagine that someone needs to say whether a number is odd or even, but there are two odd numbers on a screen, one above the other. According to the model in Figure 23.1, a priority parameter predisposes the system to assign its response, oddity, to the top number, for example. Together, these attentional factors modulate the influence of evidence on the accumulators involved in decisions and choices.
Although accumulator-racetrack models might seem highly theoretical, recent neurophysiologic research has provided evidence for these mechanisms. Accordingly, we next consider neurophysiologic evidence for neurons (i) accumulating evidence for a movement, (ii) accumulating sensory evidence, (iii) accumulating equivocal evidence, and (iv) accumulating evidence against a movement.
Neurophysiology of Accumulator-Racetrack Models
To understand the neurophysiologic data, it is important to bear in mind that the vast majority of neurons in the cortex are “tuned” for a particular region of space or some other set of stimulus parameters. The concept of a receptive field captures this idea for the sensory system. One way of looking at spatial tuning is that, due to their synaptic inputs, neurons “monitor” some region of space. Sometimes this takes the form of a circumscribed region of space, and such cells often show neuronal tuning that approximates a Gaussian function of the central point in that space. In other instances, spatial tuning involves only one dimension, such as direction (independent of amplitude) from the current location of the hand, for example.
Figure 23.2 illustrates some of the first neurophysiologic evidence for accumulator-racetrack models; it shows the activity of a neuron in the frontal eye field (FEF) of a monkey. This cell changed its firing rate 50 to 100 ms before the onset of a saccadic eye movement up and to the right. Schall et al. (12) studied its activity when they presented several stimuli of one type in the visual field (e.g., seven green squares), along with one stimulus of a different type (e.g., a red square). In their experiment, the red stimulus served as the target for a saccadic eye movement. The representation of the target emerged gradually as neural signals progressed through the visual system, from occipital to frontal cortex. When the target appeared up and to the right from the monkey’s fixation point, the FEF neuron illustrated in Figure 23.2 accumulated inputs up to its threshold for generating a saccade to that target (13). When the neuron reached its threshold quickly, the movement began with a relatively short reaction time (left arrow in Fig. 23.2). If the neuron took longer to reach its threshold, a slower response resulted (middle and right arrows in Fig. 23.2). Thus, accumulator models accord well with classic reaction-time theories (14), as extended to include neurophysiologic concepts, which hold that variation in reaction time depends upon stochastic variability in a neural integrator.
The same ideas apply to the accumulation of sensory evidence, even when that evidence is less directly linked to movement. Neurons in visual, posterior parietal (15), and prefrontal (16) areas appear to accumulate evidence about sensory inputs. Figure 23.3 illustrates neuronal activity in the middle temporal area (MT), which subserves motion vision. To study the activity of MT neurons, Roitman and Shadlen (17) used an experimental paradigm pioneered by Newsome and his colleagues (18). In these experiments, a monkey viewed a screen that has a small field of moving light spots (Fig. 23.3A). For people, if many light spots move in the same direction at the same speed, they can easily detect the coherent motion. If only a small proportion of the spots move coherently, with the rest moving randomly, detecting the coherent motion becomes more difficult. In that case, it takes longer to accumulate evidence and the reaction time increases. In applying this experiment to monkeys, the investigators made the reasonable assumption that the stimuli look the same to the monkey as to human observers. In monkeys, however, the report required some kind of movement, typically an eye movement, so the experiment could not distinguish perceptions, per se, from sensorimotor transforms. The monkey observed the stimuli for as long as necessary to make a decision, then initiated a “report” in the form of a saccadic eye movement. Near the perceptual threshold of 6% to 7% coherence, the monkeys took up to a second to respond, but with substantial variation (17,19). The experimenters later divided the trials into those that had faster reaction times (solid line in Fig. 23.3B) and those that had slower ones (dashed line). In accord with accumulator-racetrack models, a more rapid increase in neuronal activity was found to correspond to the faster reaction times, and vice versa, much like the cell activity illustrated in Figure 23.2.
Also in accord with accumulator-racetrack models, experimental results from monkeys show that sensory evidence for making a given decision acts against alternative decisions. In an experiment by Ditterich et al. (20), electrical stimulation was used to activate cells near an electrode that also recorded neuronal activity. As the monkey viewed a spot display with a moderate amount of either upward or downward motion, small amounts of current were injected in the vicinity of neurons that accumulated evidence for upward motion. The stimulation speeded up the monkey’s “report” of upward motion (Fig. 23.3C, top right). Thus, by exciting neurons thought to accumulate evidence for upward motion, the threshold of the network was attained more quickly than occurred without such artificial excitation. Importantly, the same stimulation also had an effect on the reaction time for downward motion. Excitation of neurons accumulating evidence for upward motion slowed reports of downward motion (Fig. 23.3C, bottom right). The results from this experiment show that accumulator networks appear to inhibit each other. Such inhibition would promote a winner-take-all system, in which evidence for one decision counts as evidence against others.
Figures 23.2 and 23.3 show that when accumulator networks reach their threshold for producing an output faster, reaction time decreases and vice versa. Figure 23.4 presents data showing that sometimes, in the presence of equivocal evidence, the accumulators fail to reach threshold, and therefore no movement occurs. To study these properties, Schall et al. (21) trained a monkey to make a saccadic eye movement from a fixation spot at the center of a screen to a light spot (the target) that appeared somewhere else on the screen (and quickly disappeared). On any given trial, the target could have appeared in any one of eight locations,
arranged in a circle around the central fixation point. On some trials, a masking stimulus appeared 50 ms after the target. At this critical interval, the monkey responded on some trials (called “hits”), but not on others (called “misses”), despite the fact that the sensory evidence was identical in both. As Figure 23.4 shows, the investigators found several classes of neurons in the FEF. Two of these classes showed rapid increases in activity after the target appeared. One of these classes, called visual neurons, showed little difference in activity during hits versus misses, and the activity decayed rather quickly in either case. In another class, called selection neurons, the activity increased at the same rate as in visual neurons, but decayed much less overall, and decayed less for hits than misses. These neurons seemed to accumulate information about a stimulus in the location that they monitored. A third class, called movement neurons, showed activity increases that began later (after the dashed vertical line in all parts of Fig. 23.4). These cells seemed to accumulate information about whether to make a movement to the region of space they monitored. Note that qualitatively similar neural processing occurred for hits and misses; the activity was similar in many ways when the monkey made a movement and when it did not. Accumulator-racetrack models assume that when the network’s activity fails to reach threshold, no movement occurs. In the “misses,” the evidence for making the movement was present and identical to that in “hit” trials, but for some reason the activity of the movement neurons did not reach the threshold. In looking at these cell classes, one can imagine that the influence of selection neurons on movement neurons depended on the maintenance of activity seen for “hit” trials, and without it, the activation level of the movement neurons remained subthreshold. Unfortunately, no direct evidence exists for this idea. But subthreshold decisions clearly exist. For example, stimulation of cells in the FEF evokes eye movements that deviate systematically in the direction of a subthreshold decision (19,22). A failure of such subthreshold decisions-in-the-making to reach threshold could have some relevance to negative psychomotor disorders.
arranged in a circle around the central fixation point. On some trials, a masking stimulus appeared 50 ms after the target. At this critical interval, the monkey responded on some trials (called “hits”), but not on others (called “misses”), despite the fact that the sensory evidence was identical in both. As Figure 23.4 shows, the investigators found several classes of neurons in the FEF. Two of these classes showed rapid increases in activity after the target appeared. One of these classes, called visual neurons, showed little difference in activity during hits versus misses, and the activity decayed rather quickly in either case. In another class, called selection neurons, the activity increased at the same rate as in visual neurons, but decayed much less overall, and decayed less for hits than misses. These neurons seemed to accumulate information about a stimulus in the location that they monitored. A third class, called movement neurons, showed activity increases that began later (after the dashed vertical line in all parts of Fig. 23.4). These cells seemed to accumulate information about whether to make a movement to the region of space they monitored. Note that qualitatively similar neural processing occurred for hits and misses; the activity was similar in many ways when the monkey made a movement and when it did not. Accumulator-racetrack models assume that when the network’s activity fails to reach threshold, no movement occurs. In the “misses,” the evidence for making the movement was present and identical to that in “hit” trials, but for some reason the activity of the movement neurons did not reach the threshold. In looking at these cell classes, one can imagine that the influence of selection neurons on movement neurons depended on the maintenance of activity seen for “hit” trials, and without it, the activation level of the movement neurons remained subthreshold. Unfortunately, no direct evidence exists for this idea. But subthreshold decisions clearly exist. For example, stimulation of cells in the FEF evokes eye movements that deviate systematically in the direction of a subthreshold decision (19,22). A failure of such subthreshold decisions-in-the-making to reach threshold could have some relevance to negative psychomotor disorders.
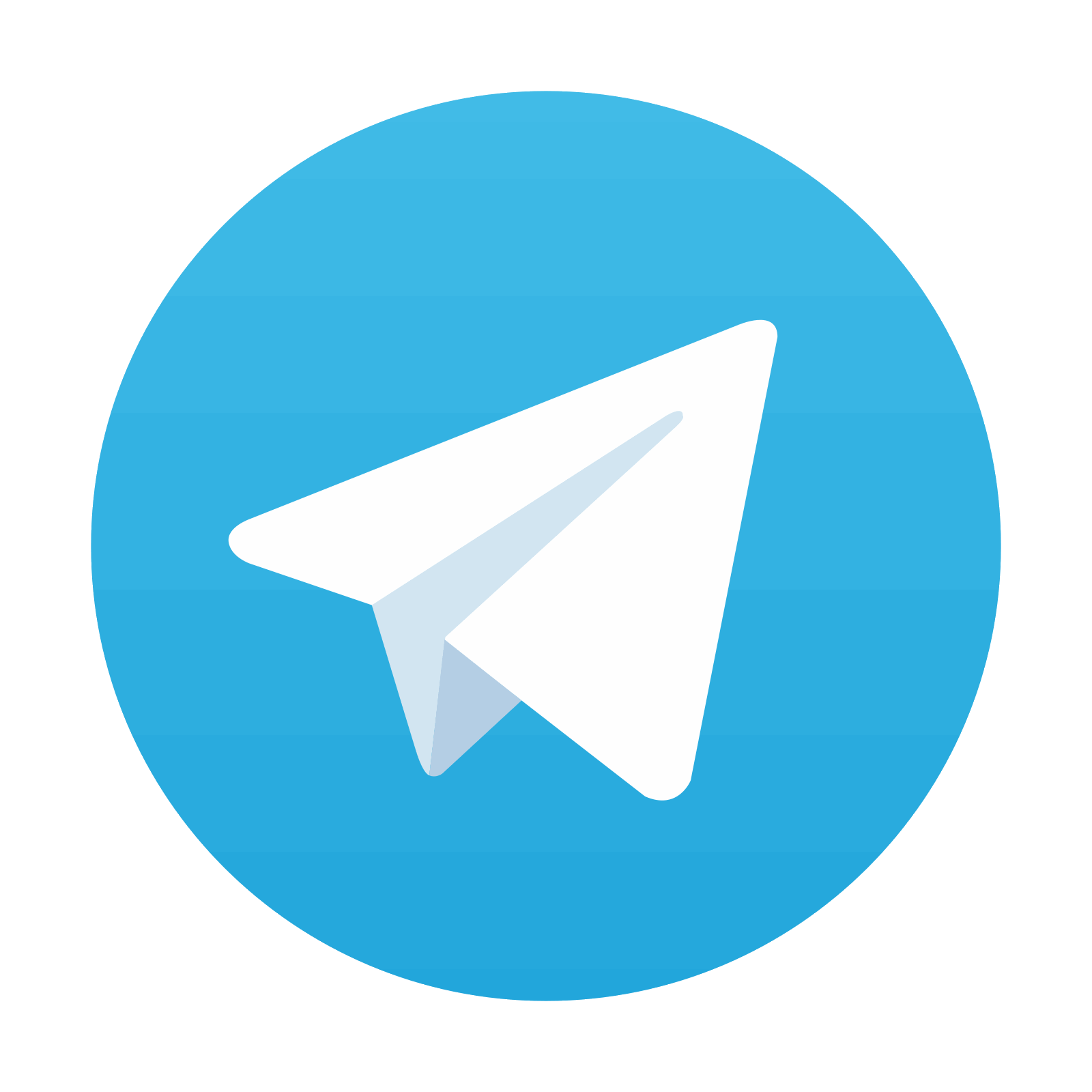
Stay updated, free articles. Join our Telegram channel

Full access? Get Clinical Tree
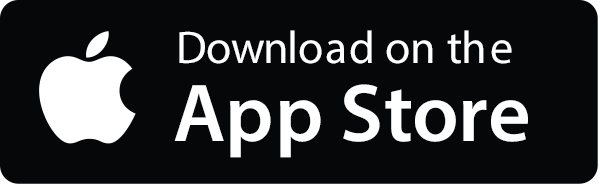
