Fig. 1
A typical setup for a study involving the measurements of the mental workload during the drive of a simulated car. The passive BCI return real-time information about the “perceived” mental effort of the subject performing the driving task
2.2 On-Line Mental Workload Estimation and Monitoring
The aim of this area of research is to design, implement and evaluate an online, passive, EEG-based BCI system to quantify the mental workload of the subject during tasks performed at three different difficulty levels, using machine learning techniques. The system was tested by subjects performing the Multi Attribute Task Battery (MATB; [7]). We tested the system during the online run performed in each session. In particular, for each session, we trained the classifier using the data acquired during the first three runs of the session (easy and hard), and we applied the parameters to the online run in order to allow the operator to visualize directly on the visual interface the output of the classifier (mental workload index), for all the subtasks (easy, medium and hard) provided by the online run (Fig. 2).
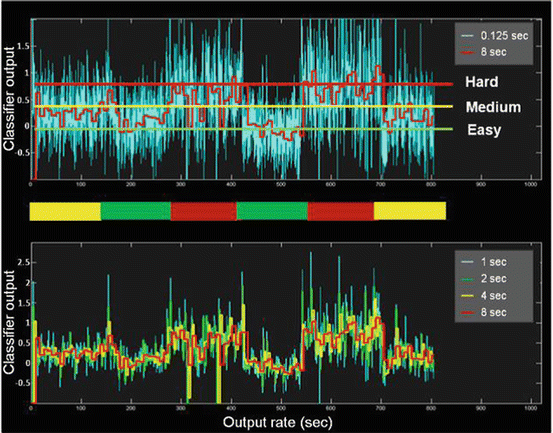
Fig. 2
Screenshot of the visual interface provided to the operator that allows to visualize the workload index (classifier output) over time. The red line is the average workload index, estimated in real time by the EEG recordings of the human operator. Note as during the different difficulty of tasks proposed to the subject (indicated by the different colors of the bar at the center of the figure, being red the most difficult task, and the green the easiest one) the workload index shows a different value. The higher the index, the higher the estimated workload
2.3 Neuromarketing
The final goal of the study is to link significant variations of electroencephalographic and autonomic variables with the cognitive and emotional reactions during the observation of TV advertisements. In an interview after the recording, subjects were asked to recall commercial clips they remembered and to score them according to the degree of pleasantness they perceived. Hence, the dataset has been divided into several subgroups in order to highlight differences between the cerebral activity related to the observation of the remembered and forgotten ads, and those between the liked against the disliked commercials. Both cognitive and emotional processing have been described by the Memorization (MI), Attention (AI) and Pleasantness Index (PI), which have been presented a previous work [8]. The percentage of spontaneous recall is linearly correlated with the MI values (R2 = 0.68, p < 0.01). In particular, when both MI and AI are under their average values the percentage of spontaneous recall (18 %) is under average as well. This percentage is slightly increased (20 %) when the AI exceeds the average threshold. The highest values of spontaneous recall correspond to MI values over average. In fact, in this case the percentage reaches the value of 33 % when the AI is under average and the value of 41 % when both MI and AI are over average (Fig. 3).

Full access? Get Clinical Tree
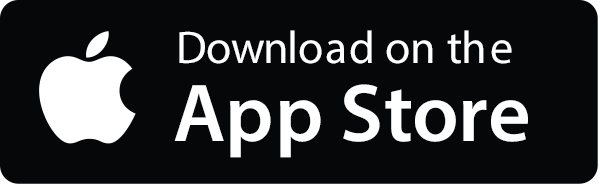
