Disclosures
LCB is a stockholder and former employee of Myriad Genetics, former employee and current consultant to Tempus Labs, Principal Consultant for Great Scott! Consulting, founding member of Pharmacogenetics Research Network (PGRN), and member of the Clinical Pharmacogenetics Implementation Consortium (CPIC). CAB is the founder and shareholder of Sequence2Script Inc. and a member of the CPIC and Pharmacogene Variation Consortium (PharmVar). HAE is a former employee of CNSdose.
Introduction
One aspirational approach to improve treatment response in major depressive disorder (MDD) and treatment-resistant depression (TRD) is to utilize biomarkers to aid accuracy of diagnosis and selection of more precise treatments ( ). One must also appreciate the role of correct diagnosis in utilizing biomarkers to improve treatment response. Biomarkers for diagnosis, such as polygenic risk scores, may also improve treatment decisions and therefore outcomes for individuals with depression and comorbid illnesses. Areas such as biomarkers in pharmacogenomics, epigenomics, proteomics, metabolomics, and microbiomics as well as algorithms such as machine learning and artificial intelligence may hold insights into matching a more effective treatment to each individual patient ( ). In this chapter, we will focus on the roles of pharmacogenomics (PGx) and algorithms to aid in treatment decisions to improve patient outcomes in MDD and TRD. Specifically, we will review the gradually improving performances of PGx tests and briefly describe progress in strategies to improve them further using artificial intelligence (AI) and machine learning (ML). We will also discuss relevant considerations for use of such tests such as optimal clinical times for administering PGx tests; recent trends in insurance coverage and federal programs for biomarker tests; and updates of FDA- drug labels for these new biomarkers. The “PGx” abbreviation in this chapter will be inclusive of both pharmacogenomic and pharmacogenetic approaches. The term “algorithm” will be inclusive of algorithmic approaches such as machine learning (ML) and artificial intelligence (AI).
Brief history of pharmacogenomic tests and algorithms for clinical use
The link between genes and mood disorders have been recognized since the Hippocratic era, but clinical approaches designed to leverage an individual’s genetic information to personalize therapy have taken centuries to develop ( ; ).
For most major medical disorders, biomarkers are demonstrated to be pivotal—even indispensable—for progress. Examples abound in oncology, cardiovascular medicine, pulmonary medicine, neurology, and other arenas ( ). The potential value of biomarker measures when treating cancers and other medical disorders gained credibility during the 1950s and helped fuel interest in PGx tests for brain-behavior illnesses. During cancer treatments, individual differences in genes and enzymes were shown to alter metabolism, clinical effectiveness and toxicity of antineoplastic medications ( ). Genetic outliers were found to have poorer clinical outcomes, sometimes because of ultrarapid metabolism requiring greater dosing, sometimes because of genetic patterns that led to poor metabolism, side effects, poor adherence, and the need for lower doses. The value of such information for treatment selections became evident, and clinical desires for biomarkers became more widespread.
Despite a growing desire for biomarkers, progress has been particularly slow in developing precision-based biomarkers to aid antidepressant selection and adjustments for the treatment of MDD, and for resolving TRD should it occur. Consequently, physicians are forced to make important clinical treatment decisions based primarily upon a collection of subjective and often erroneous clinical determinations for antidepressants such as: (1) “MDD depressions generally are the ‘same illness’ so can be treated using the same treatments”; (2) “One-size treatment tends to fit all so I will use my favorite”; and (3) “that didn’t work, so let’s try this.” These factors are common launching pads for the development of TRD. Fortunately, research advances are accelerating, and a variety of PGx tests and algorithms are beginning to show promise ( ; ).
Facilitating this acceleration is a current estimate that suggests genomic variation explains approximately 80% of the variability in drug efficacy and adverse effects for most medical illnesses ( ). Likewise, the past decade has been witness to a growing recognition that an individual’s genes play an important role in psychotropic treatment response, in part attributed to Dr. David Mrazek’s pivotal book on Pharmacogenomics ( , India slides, p. 3). He defined psychiatric pharmacogenomics as “the study of how gene variations influence the responses of a patient to treatment with psychotropic medications.” Mrazek emphasized that gene actions stemming from variations in pharmaco kinetic (PK) genes helped determine how one’s body affects the drug such as metabolizing rapidly or poorly, whereas variations in pharmacodynamic (PD) genes predominantly changed how the drug affects the body, potentially altering clinical response. Importantly, Mrazek and other investigators clarified that pharmacodynamic genes and pharmacokinetic genes interactively assert their composite actions, that medication effects are regulated by a multitude of genes and enzymes, and that most clinical decisions could be improved only by integrating and considering these composite considerations. However, most evidence in the field of pharmacogenomics relies on single gene-drug pairs and this composite or combinatorial approach is limited (PMID: 31004441) ( Fig. 7.1 ).

Evidence for pharmacogenomic testing in clinical practice
Methodological shortcomings used in nascent PGx tests and early publications were quite recognizable. Many were understandable because of absent or minimal sustained funding support, and studies were often plagued by small samples, lack of standardized clinical evaluations, limited focus upon single genes or enzymes, short durations of follow-up, no blinded ratings, and an array of other reasons. Commercial or parochial origins of emerging PGx tests for mood disorders also generated concerns ( ; ).
Efforts to minimize or overcome research shortcomings and accelerate PGx tests focus on both studies from academic/healthcare institutions and commercial PGx companies. The majority of evidence for prospective clinical utility of PGx testing has come from commercially sponsored clinical randomized controlled trials. Multiple meta-analyses have analyzed the overall effect of these studies and PGx testing efficacy in depression (PMID: 30520364).
Of note, multiple studies did show improved outcomes with PGx testing when decisions were congruent (or aligned) with the PGx test report. This indicates that individuals with gene-drug interactions may benefit the most from PGx testing ( ; ; ; ). “Congruence” generally is used to mean “in agreement,” “in harmony” or “compatible.” “Incongruence” implies its absence. While few clinicians traditionally use the terms “congruency” or “incongruency” with regard to medications, many have known about the consequences of prescribing genomically incongruent medications, i.e., those that may not be compatible with the recipient’s genomic composition. An example would be a medication for which FDA approval was based on average rates of metabolism, but when given to an individual with “incongruent” genetic composition, the results could be ineffective or undesirable. Incongruent medications for an individual commonly result in more adverse events and side effects, lower adherence, more medication discontinuations, less likelihood to achieve improved responses by failure to alter underlying, more achieving lower remission rates, and poor, disappointing outcomes. These all contribute to TRD and enable its devastating consequences.
Importantly, some experts have argued that RCTs should not be the gold standard in evaluating PGx testing because it does not mirror real clinical practice ( ; ). While open-label studies risk bias without a “placebo” standard of care arm, implementation mirrors real clinical practice. One of the largest, prospective clinical trials, Individualized Medicine: Pharmacogenetic Assessment & Clinical Treatment (IMPACT), evaluated the implementation of PGx testing in both primary care provider (PCP) settings and specialist psychiatry settings in almost 2000 patients and found that patient outcomes were actually better in individuals under the care of a PCP compared to a psychiatrist, indicating that PGx testing can be used in both specialist and nonspecialist settings ( ).
For individuals with MDD, the gold standard algorithm was when MDD treatments were compared in the Sequenced Treatment Alternatives to Relieve Depression (STAR*D) study. This study showed that with each medication trial, response and remission decreases while side effects increase. Interestingly, Fan et al. performed a PGx analysis simulating the STAR*D algorithm to determine potential gene–drug interactions ( ). The authors found that in the first step of STAR*D, which includes the prescription of citalopram, ~ 30% of patients had genetic implications that would change how the medication was administered. In the second step, ~ 21% had actionable gene-drug interactions. These data beg the question, that if PGx had been used to guide treatment decisions, would outcomes in STAR*D have been improved?
PGx testing to help guide medication selection appears to meaningfully help eliminate or reduce exposure to incongruent medications and to guide clinicians into avoiding their use. When making determinations about congruity vs. incongruity, PGx tests appear to more objectively integrate contributing factors, whether those be pharmacokinetic or pharmacodynamic. Consequently, should combinatorial PGx testing identify medications with greater likelihood for incongruency, that medication ideally should be avoided if possible, even should it be a “favorite” treatment for the clinician. Incongruency matters. Switching to achieve congruency matters.
PGx economic studies
Expenses for biomarker tests are areas of concern for many. Multiple Health Economic Outcomes Research (HEOR) studies have been performed in order to determine the economic validity and utility of PGx testing. Prospective, retrospective, and modeling studies have been performed. The largest prospective study to date, evaluated medication cost savings in individuals with psychiatric disorders receiving psychotropic medication prescriptions with and without guidance from PGx testing ( ). The study included over 13,000 patients and found that PGx testing resulted in savings of $1035.60 USD per year compared to standard of care. Interestingly, prescribing that was congruent with the test resulted in even greater savings of $2774.53 compared to standard of care. Equally interesting, a subanalysis of this study also found that individuals receiving psychotropic prescriptions from PCPs congruent with the PGx test saved $3988 per year compared to $1308 per year under the care of psychiatrists ( ). These data support the results of the IMPACT study that PCPs can not only implement PGx testing effectively, but implementation is also cost saving. A large, retrospective study of health insurance claims found that in over 3500 individuals, those whose treatment was guided by PGx testing had significantly less healthcare utilization, including fewer emergency room visits and in-patient hospitalizations ( ).
Some systems such as Medicare and private health insurance companies have now begun to reimburse portions or total PGx testing expenses for specific patients. Most often, this reimbursement is for individuals with depression who have failed at least one medication and whose clinician is considering a medication with a known gene-drug interaction ( ). Cost and insurance coverage variables may turn out to be among the most influential in determining future refinements, more widespread use, and accessibility of PGx testing.
Algorithms for medication guidance
One limitation to PGx testing is that it tends to be diagnostic and clinically agnostic, meaning it only takes into account specific gene-drug interactions alone, without considering other features of the individual such as diagnosis, symptoms, concomitant medications, etc. Other approaches to try to identify markers of disease and treatment response have also taken a singular approach. Algorithmic tools that take multiple features into account may improve the use of PGx testing or be valuable tools on their own. These tools can also incorporate patient reported features such as measurement-based symptom scales. Three algorithmic pathways with great promise for guiding medication treatment (and potentially even diagnosis) are machine learning (ML), artificial intelligence (AI), and polygenic risk scores (PRS). Here we discuss the current landscape for ML, AI, and PRS in guiding medication decisions. In 2021, the FDA released an action plan for AI/ML-based software as a medical device for regulating AI and ML algorithms in healthcare ( ).
Machine learning for medication guidance
Machine Learning is the ability to create or train a model and then apply that model to a new set of data. For example, Chekroud et al. took patient reported data from the STAR*D study to identify features predictive of medication response ( ). The authors trained the model on these data then applied the output to the results of the Combining Medications to Enhance Depression Outcomes (CO-MED) trial to determine if the model could also predict outcomes in this study. Overall, the model was able to predict outcomes with 59.6% accuracy. Another study sought to use ML to predict TRD ( ). The group trained the ML algorithm on STAR*D and applied the algorithm to the Canadian Biomarker Integration Network in Depression (CAN-BIND1) dataset. The ML algorithm predicted the risk for TRD with 70%–73% accuracy.
Even more valuable tools are those that integrate genomic data and ML. Athreya et al. trained a model on baseline depression data from Mayo Clinic Pharmacogenomics Research Network Antidepressant Medication Pharmacogenomic Study (PGRN-AMPS) and in conjunction with genome-wide association date, applied the model to STAR*D and the International SSRI Pharmacogenomics Consortium (ISPC) ( ). The study showed that when using mutations and baseline depression score, trained ML predicted remission and response in PGRN-AMPs with an AUC > 0.7 and in STAR*D/ISPC with an AUC > 69%. This study showed that ML can be used to combine clinical features and PGx.
AI for medication guidance
In order to understand if AI is acceptable and can be implemented in care, a recent group evaluated the acceptability and impact of an AI-based clinical decision support tool ( ). The investigators reported that the AI tool would increase patient understanding and was valuable in guiding treatment decisions.
Genome-wide association studies and polygenic risk scores (PRS) for diagnosis and medication guidance
With the surge in genomic medicine and technologies, polygenic risk scores (PRS) have become the next big biomarker in psychiatry for both diagnostics and medication response ( ; ). PRS is the use of genome-wide sequencing to associate multiple variants in multiple genes with a specific trait. One of the first large-scale PRS studies was published in 2017 for schizophrenia and identified 108 loci associated with the disease ( ). Since then, PRSs have been evaluated for other diseases such as postpartum depression, MDD, Bipolar Disorder, attention deficit hyperactivity disorder, and autism spectrum disorders ( ; ; ; ; ; ). Interestingly, investigators have also sought to apply disease PRS for medication response. One study showed that individuals with higher PRS for schizophrenia had more schizophrenia symptoms post-antipsychotic treatment, indicating a genetic load against treatment response ( ). Another study showed that individuals with MDD and a high PRS for schizophrenia were less likely to respond to treatment ( ). Finally, another study showed that genetic liability for depression increased risk for suicide ( ). Impute. Me is an open-source program that allows individuals to upload their genomic information and retrieve PRS for various traits such as anxiety, athleticism, asthma, etc. ( ).
A note on black box algorithms
Dr. David Mrazek was the first to utilize the fact that multiple genes affect multiple medications at once, and therefore many commercial companies have created proprietary PGx algorithms to implement this information into clinical practice ( ). However, much controversy over the secrecy of these algorithms exists ( ). While companies may publish clinical data supporting these algorithms, the lack of transparency means that others cannot replicate or validate the algorithms ( ). Clinicians and healthcare decison-makers can argue that they want to know what is in the “black box” if it’s going to be used in patient care. Proponents, however, have argued that the black box approach is aligned with the idiosyncratic manner by which most clinicians treat patients, the unknown mechanisms of many medications, and the conventional commercial roles in developing new treatments or tests ( ; ). Efforts must be made to increase the transparency, explainability, and value of black-box algorithms. We recently provided recommendations to encourage this ( ).
Future directions for PGx and algorithm testing
While the evidence for PGx testing efficacy to guide medication decisions in individuals with depression who have failed one medication is strong, efficacy in other populations and varying clinical features is unknown. Many questions remain for the implementation of ML and AI algorithms. Future questions that need to be addressed with PGx testing and algorithms are outlined below:
Should PGx/algorithm testing be done proactively or retroactively, before first treatment with medications or only after failure to respond?
Will PGx/algorithm results help predict vulnerability for and/or aid in reducing suicides?
Will PGx/algorithm testing improve identification of individuals whose antidepressant or mood-stabilizing medications could be removed safely following resolution of depressive episode?
Will PGx/algorithms be useful in guiding treatment selections for individuals with other diagnoses than MDD such as Bipolar Spectrum Disorders, schizophrenia, anxiety, etc.?
Can PGx/algorithm testing help predict manic switches in MDD and bipolar disorders?
Will PGx/algorithm testing help identify individuals who may benefit from placebo?
Will PGx/algorithm testing results remain consistent over time among individuals who continue to suffer additional episodes? Might such a clinical pattern suggest a different etiology cause and the need for different precise treatments?
Will ethnicity differences alter interpretation of PGx/algorithm test results? Data from other pharmacogenomic assessments such as coagulation studies suggest this will be the case.
Will PGx/algorithm test results be significantly altered or made invalid by cooccurring medical illnesses and/or medications?
Can the PGx/algorithm fields adhere to responsible innovation in neurotechnology principles?
References
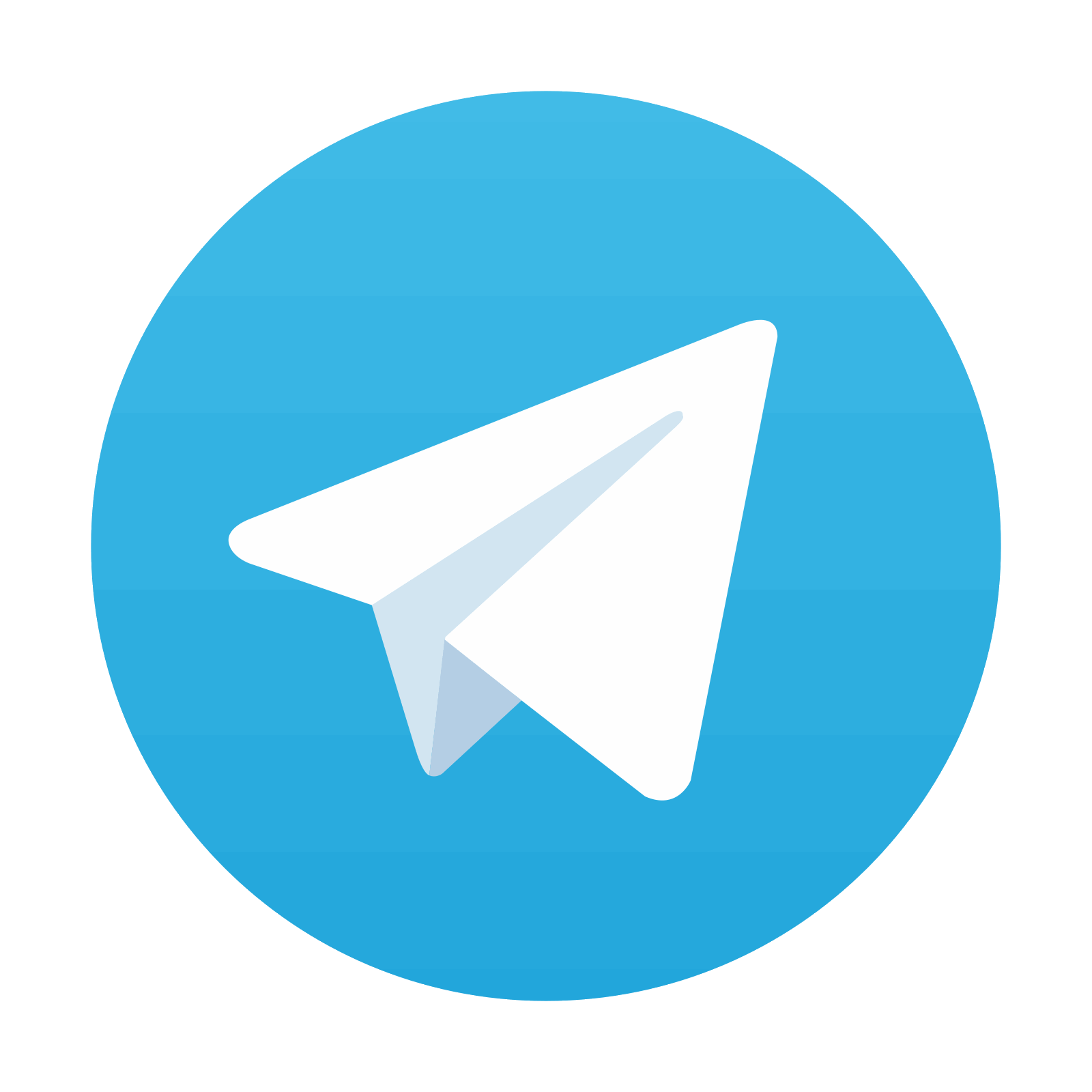
Stay updated, free articles. Join our Telegram channel

Full access? Get Clinical Tree
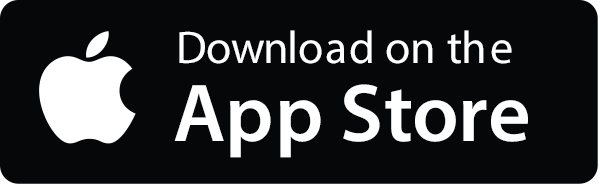

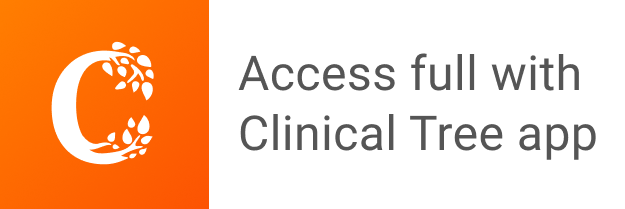