5
Trial Designs to Establish Efficacy and Safety in Multimodal Therapies
Evaluating multimodal therapies for brain disorders is a complex problem and likely to be slow, costly, and inefficient unless innovative strategies are employed that directly address this complexity, said Roger Lewis, senior medical scientist at Berry Consultants, LLC, and professor and chair in the Department of Emergency Medicine at Harbor-UCLA Medical Center. With the goal of delivering more effective treatments to patients with complex diseases that require multimodal interventions, challenges arise with regard to scaling research efforts to incentivize the involvement of multiple vendors or stakeholders so that questions can be asked about the interactions of multiple modes of interventions, such as whether effects are additive or synergistic. As with other clinical trials, failing early and efficiently is important so that resources are not wasted on approaches that are unlikely to be successful, said Lewis. Therefore, he proposed two intersecting strategies: platform trials and adaptive trials.
PLATFORM TRIALS AND ADAPTIVE TRIALS
Lewis defined a platform trial as an experimental infrastructure built to efficiently evaluate multiple treatments or combinations of treatments, either in a disease or group of diseases, that is intended to survive the evaluation of any individual treatment. Efficiency derives from eliminating the need to repeatedly create and disable the clinical trial infrastructure as new treatments become available for testing (Berry et al., 2015). It provides incentives for participation, teamwork, and the achievement of scale, said Lewis, because costs—including the cost of the control group—are shared across multiple stakeholders.
Fundamental to achieving efficiency in a platform trial is the concept of adaptation as knowledge is gained, Lewis noted. Adaptive trials leverage the fact that partial information gained from patients enrolled early in the trial can be used to make changes in the trial design in order to optimize the search for treatments with the greatest promise for improving outcomes. Lewis added that the technique of response-adaptive randomization enables modification of randomization proportions so that later patients are preferentially shunted toward the arms, or combinations of arms, that appear most effective. Randomization rules are prespecified to minimize bias and errors, including false-positive and false-negative results.

SOURCE: Presentation by Lewis, June 15, 2016.
Lewis illustrated the evolution of a platform trial over time, as shown in Figure 5-1. In this illustration, three treatments are tested. In the first group of patients, interventions are randomized equally to six arms: A, B, C, AB, BC, and AC. Outcomes from the first cohort of patients are used to unbalance the randomization in the next stage of testing, emphasizing assignment to arms that appear most promising. In subsequent stages of the trial, an arm may be dropped if it appears ineffective (treatments A, AC, and AB in the illustration). Other treatment arms could also be added (not shown in the illustration). The model also illustrates the concept of population enrichment, another adaptation that enables subgroups of individuals to be dropped from the study if they do not appear to benefit from any of the treatments under investigation. Together, adaptive randomization and enrichment increase efficiency by shifting trial resources to the most effective treatments and the populations most likely to benefit.
Adaptive randomization may also be based on intermediate endpoints, which is particularly important for neurological diseases, where traditional endpoints such as survival or time to an event may require long-duration trials. Lewis cited the example of the rare neuromuscular disease GNE myopathy, which is characterized by slow, progressive muscle weakness. Patients demonstrate a characteristic pattern of progression (Nishino et al., 2015) that, according to Lewis, could be translated into a quantitative endpoint, which might be used for adaptive randomization.
Large factorial designs for multimodal therapies that involve different therapeutic approaches such as behavioral therapies introduce additional complexity. For example, Figure 5-2 shows schematically the number of arms (boxes) that could be required for a trial combining three different drugs with three different therapies in three different population subgroups. Spreading trial participants across so many arms could require huge sample sizes. However, Lewis described restricted factorial designs that use external information to restrict the number of arms required. For example, if it is already known that certain drugs do not work with certain therapies; those boxes could be eliminated. There are also statistical approaches that incorporate clinical knowledge about the patient population and the effectiveness of therapies that can be used to optimize the trial parameters.
Planning a complicated adaptive trial requires the use of simulations to demonstrate the effects of adjusting trial parameters, including sample size, effect size, dropouts, etc. These additional evaluations through simulation before a trial begins can be extensive, said Lewis, but will save substantial time overall. More importantly, he said, these trials and the simulations that are used in their design increase the likelihood of obtaining a clear answer at the end. The worst thing that can happen in a large clinical trial is to have made a substantial investment without learning anything, such as whether the trial failed because the dose was wrong, the duration too short, or the patient population inappropriate, noted Lewis. He maintained that adaptive trials can be thought of as “rightsized trials” that can also increase efficiency because failures from lack of efficacy would be seen earlier, thus enabling sponsors to redirect resources to other projects.

SOURCE: Presentation by Lewis, June 15, 2016.
Adaptive trials are particularly valuable in disease areas where there is a large degree of uncertainty with regard to defining the parameters of the trial, Lewis said. The adaptive approach specifically addresses uncertainty by utilizing simulations over the full range of uncertainty to determine how well the trial will perform under certain conditions, and under which conditions the trial is less likely to perform well.
Although adaptive randomization1 has been endorsed by regulators, because of the novelty of these approaches the analysis and interpretation of data emerging from these studies will be considered carefully on a case-by-case basis, according to Dunn. The high-level issue, he said, is whether the submitter has tested its hypothesis in a rigorous manner that results in interpretable data.
QUANTIFYING DOSE WITH NON-PHARMACOLOGIC INTERVENTIONS
With any intervention, controlling the dose is essential to determining what does and does not work; however, this can be particularly challenging for behavioral and neuromodulation interventions because of variability in how practitioners use scales and how devices are deployed, according to Marom Bikson of the City University of New York.
Quantifying dose and determining the correct dose are two different things, added Bikson. For both pharmaceuticals and neurostimulation, the body transforms the dose into some level of activity. The response to the dose can thus be used to adjust the dose to the appropriate level. Dosing is further complicated by the fact that for some diseases, such as epilepsy, doses are not generalizable, but must be individualized, said Martha Morrell. Moreover, there is not a dose for everybody, and the appropriate dose for a single individual changes depending on environmental conditions, time of day, stress, stage of disease, etc. What is needed, said Morrell, are dynamic markers that can be quantitated in real time.
Quantifying Dose with Devices
With neuromodulation, the dose is defined by all parameters of the stimulation device that affect the electric and current density fields generated in the body (Peterchev et al., 2012), that is, the position and electrical properties of the electrode/coil (waveform), repetition frequency, train duration, interval, and number of sessions, said Bikson. The result is current flow in the body, which produces physiologic changes that can be measured as clinical outcomes; however, the response itself is not the dose, but can be used to adjust the dose, for example, by moving the placement of the coil or dialing back on the intensity. Thus, because placement of the coil or electrode is one of the parameters that determines dose, two people getting DBS with the same delivery platform, for example, may be getting very different doses. Bikson compared this to changing the chemical composition of a drug, such that two tablets can provide very different doses and have different effects. Moreover, because of individual variations in the body, as with pharmaceuticals, what shows up at the target tissue may be very different despite delivery of the same dose, said Bikson. The dose is defined by parameters that can be controlled by the operator, but outcomes relate to what actually shows up at the target.
Quantifying Dose with Psychosocial Interventions
Standardizing and quantifying how psychosocial interventions are delivered and measured is particularly challenging, said Karl Kieburtz. In psychotherapy, for example, dose is usually defined by the number of sessions and the duration of treatment, said Wolfgang Lutz from the University of Trier in Germany. Based on a meta-analysis of 15 studies comprising more than 2,400 patients, Howard and colleagues (1986) proposed a dose−response model in 1986 that linked the number of sessions to improvement in symptoms. This and subsequent studies have indicated a log-linear dose−response relationship over the course of treatment; that is, a dose−response curve that shows diminishing effectiveness of treatment over time (Stulz et al., 2013). Another model, called the “good enough model,” proposes that clients stay in treatment until they believe they have a good enough level of improvement (Bark-ham et al., 2006). All of these studies define dose as number of sessions, said Lutz.
Lutz added that within the field, there is debate about whether psychometric feedback over the course of treatment might provide additional information about treatment response that could lead to more optimized and personalized dosing strategies. This has led to the development of treatment prediction, selection, and adaptation tools (DeRubeis et al., 2014; Lutz et al., 2014; Rubel et al., 2015). However, he has also shown that the receptiveness of the therapist to feedback determines, in part, the likelihood of a positive response to the therapy (Lutz et al., 2015). Furthermore, Lutz said most treatment decisions are determined by how many sessions an insurer will cover rather than by empirical methods, and he suggested that dose is a neglected area of research in psychosocial interventions.
Payers are especially interested in determining the number of sessions needed for psychotherapy. However, according to Rhonda Robinson-Beale, studies are typically based on a protocol rather than on the natural trajectory of the illness. Another problem related to dose in psychotherapy, said Robinson-Beale, is that training varies from discipline to discipline and from one therapist to the next, and rarely involves the use of feedback and validated tools. Providing feedback to the practitioner and patient, such as by assessing and reporting global distress, may provide a feedback loop to better identify the appropriate number of sessions, she said.
ASSESSING RESPONSE TO INTERVENTIONS
Regardless of how the dose of neuromodulation is measured, the effect of the intervention is determined not just by what is administered, but by the brain’s response to it, and this requires measurement of both the neurophysiological effects of the intervention (e.g., through EEG or functional magnetic resonance imaging), the effects on the function of the neural circuit that was targeted (e.g., working memory performance), and the patient’s subjectively reported symptoms (e.g., depression rating scales), said Lisanby. She added that while there are limitations to self-report scales, subjective assessments of symptoms are also used to make a diagnosis. For neurostimulation, the most valuable markers, particularly for interventions that are delivered over a long period of time, are those that precede a clinical signal, said Bikson, such as intracranial epileptiform activity in epilepsy. Lisanby cited the need to identify short-term markers that are objective and quantifiable, and that could predict long-term change in a complex behavioral disorder such as depression or pain. Bikson concurred, noting that there is a strong precedent in the neuromodulation field for using adaptation and monitoring. For example, a single-session measure that indicates how responsive a patient might be to TMS could dramatically increase the ability to adjust dose.
In the psychotherapy area, what is actually administered in the therapist’s office is not typically measured, said Lisanby. There is potential to rectify this by using sensors that assess social, auditory, and nonverbal interactions between therapist and patient, she said. Lutz’s group is investigating using video recording to capture movements and interactions between therapist and patient at a micro level. Kieburtz added that it might also be helpful to measure objectively the subject’s internal state at the time a therapy is administered by measuring galvanic skin response or EEG, for example. Although these measures might interfere with psychosocial interventions, Lisanby noted that sensors are available to measure facial expression, facial intonation, the verbal content of speech, and other responses that reflect the social context and psychological state might be measured less intrusively. This is analogous, she said, to the measures used during multimodal therapy involving cognitive training in combination with brain stimulation, described earlier in the workshop by Luber, where reaction time, accuracy, and other variables are quantified and these data are used in an iterative feedback fashion to adjust the training. These measures represent a behavioral readout of neural circuits, and may also provide some information about the biological origins of the psychological state, said Lisanby. The complexities associated with obtaining reliable and objective measures of treatment response further highlight the challenge in studying and assessing the efficacy of multimodal therapies that include a psychosocial intervention component.
__________________
1 For additional information and examples of adaptive randomization see Kuehn (2006) and Meurer et al. (2012).
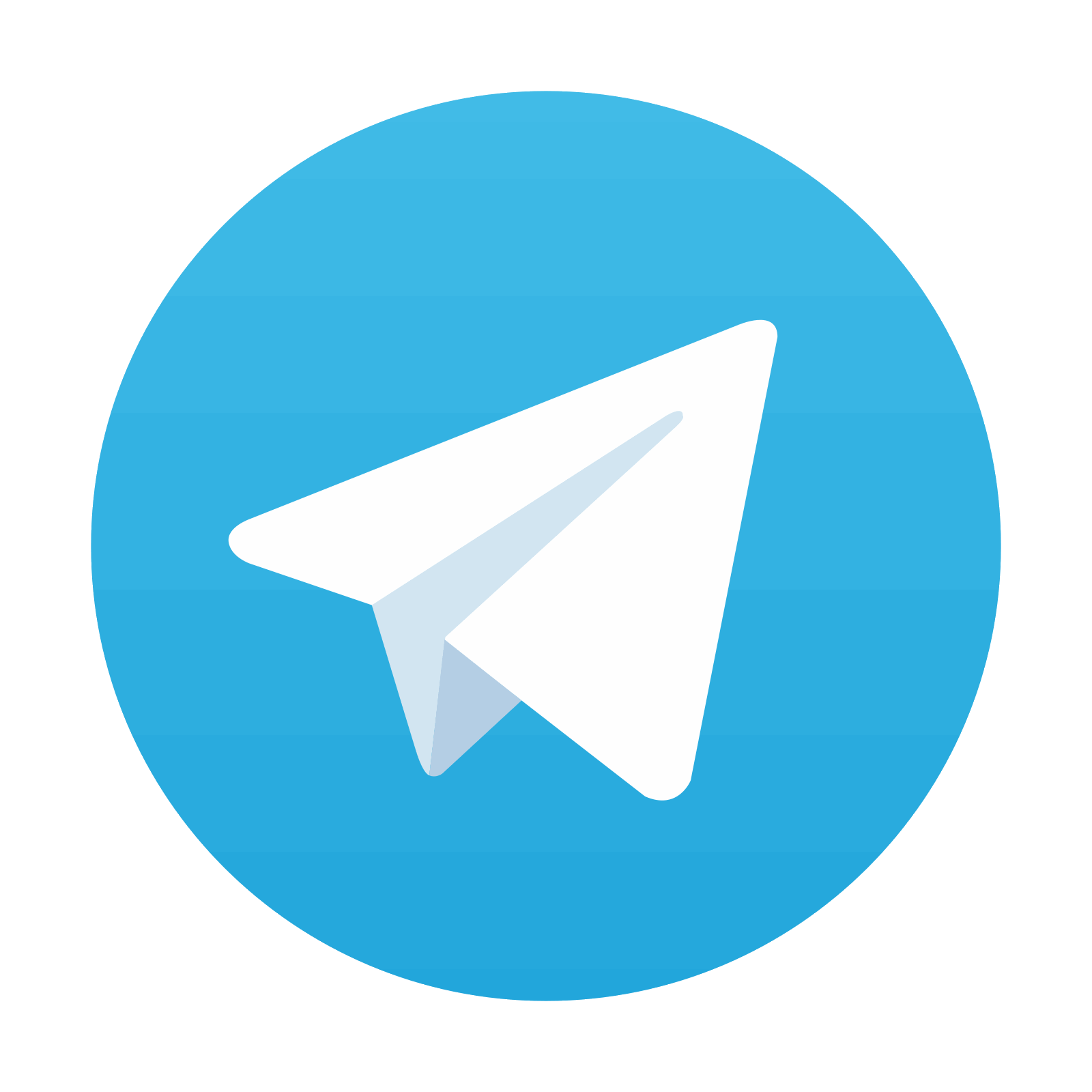
Stay updated, free articles. Join our Telegram channel

Full access? Get Clinical Tree
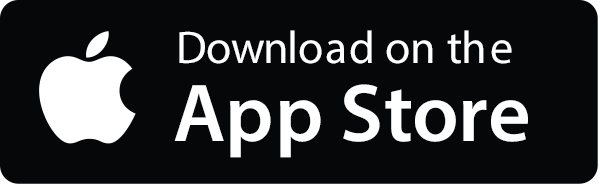
