Understanding Research Methods and Statistics: A Primer for Clinicians
George A. Morgan
Jeffrey A. Gliner
Robert J. Harmon
Introduction
Purposes of Research
Research has two general purposes: a) increasing knowledge within the discipline and b) increasing knowledge as a professional consumer of research in order to understand and evaluate research developments within the discipline. For many clinicians, the ability to understand research in one’s discipline may be even more important than making research contributions. Dissemination occurs through an exceptionally large number of professional journals, workshops, and continuing education courses, as well as popular literature. Today’s professional cannot simply rely on the statements of a workshop instructor to determine what should be included in an intervention. Even journal articles need to be scrutinized for weak designs, inappropriate data analyses, or incorrect interpretation of these analyses. Current professionals must have the research and reasoning skills to be able to make sound decisions and support them.
Research Dimensions and Dichotomies
Self-Report versus Researcher Observation
In some studies the participants report to the researcher about their attitudes, intentions, or behavior. In other studies the researcher directly observes and records the behavior of the participant. Sometimes instruments such as heart rate monitors are used by researchers to “observe” the participants’ physiological functioning. Self-reports may be influenced by biases such as the halo effect, or participants may have forgotten or not thought about the topic. Many researchers prefer observed behavioral data. However, sensitive, well trained interviewers may be able to alleviate some of the biases inherent in self-reports.
Quantitative versus Qualitative Research
We believe that this topic is more appropriately thought of as two related dimensions. The first dimension deals with philosophical or paradigm differences between the quantitative (positivist) approach and the qualitative (constructivist) approach to research (1). The second dimension, which is often what people mean when referring to this dichotomy, deals with the type of data, data collection, and data analysis. We think that, in distinguishing between qualitative and quantitative research, the first dimension is the most important.
Positivist Versus Constructivist Paradigms
Although there is disagreement about the appropriateness of these labels, they help us separate the philosophical or paradigm distinction from the data collection and analysis issues. A study could be theoretically positivistic, but the data could be subjective or qualitative. In fact, this combination is quite common. However, qualitative data, methods, and analyses often go with the constructivist paradigm, and quantitative data, methods, and analyses are usually used with the positivist paradigm. This chapter is within the framework of the positivist paradigm, but the constructivist paradigm provides us with useful reminders that human participants are complex and different from other animals and inanimate objects.
Quantitative Versus Qualitative Data, Data Collection, and Analysis
Quantitative data are said to be “objective,” which
indicates that the behaviors are easily and reliably classified or quantified by the researcher. Qualitative data are more difficult to describe. They are said to be “subjective,” which indicates that they are hard to classify or score. Some examples are perceptions of pain and attitudes toward therapy. Usually these data are gathered from interviews, observations, or documents. Quantitative/positivist researchers also gather these types of data but usually translate such perceptions and attitudes into numbers. Qualitative/constructivist researchers, on the other hand, usually do not try to quantify such perceptions; instead, they categorize them.
indicates that the behaviors are easily and reliably classified or quantified by the researcher. Qualitative data are more difficult to describe. They are said to be “subjective,” which indicates that they are hard to classify or score. Some examples are perceptions of pain and attitudes toward therapy. Usually these data are gathered from interviews, observations, or documents. Quantitative/positivist researchers also gather these types of data but usually translate such perceptions and attitudes into numbers. Qualitative/constructivist researchers, on the other hand, usually do not try to quantify such perceptions; instead, they categorize them.
Data analysis for quantitative researchers usually involves well defined statistical methods, most often providing a test of the null hypothesis. Qualitative researchers are more interested in examining their data for similarities or themes which might occur among all of the participants on a particular topic.
Variables and Their Measurement
Research Problems and Variables
Research Problems
The research process begins with a problem. A research problem is an interrogative sentence about the relationship between two or more variables. Prior to the problem statement, the scientist usually perceives an obstacle to understanding.
Variables
A variable must be able to vary or have different values. For example, gender is a variable because it has two values, female or male. Age is a variable that has a large number of potential values. Type of treatment/intervention is a variable if there is more than one treatment or a treatment and a control group. However, if one studies only girls or only 12-month-olds, gender and age are not variables; they are constants. Thus, we can define variable as a characteristic of the participants or situation that has different values in the study.
Operational Definitions of Variables
An operational definition describes or defines a variable in terms of the operations used to produce it or techniques used to measure it. Demographic variables like age or ethnic group are usually measured by checking official records or simply by asking the participant to choose the appropriate category from among those listed. Treatments are described in some detail. Likewise, abstract concepts like mastery motivation need to be defined operationally by spelling out how they were measured.
Independent Variables
We do not restrict the term independent variable to interventions or treatments. We define an independent variable broadly to include any predictors, antecedents, or presumed causes or influences under investigation in the study.
Active Independent Variables
An active independent variable such as an intervention or treatment is given to a group of participants (experimental) but not to another (control group), within a specified period of time during the study. Thus, a pretest and posttest should be possible.
Attribute Independent Variables
A variable that could not be manipulated is called an attribute independent variable because it is an attribute of the person (e.g., gender, age, and ethnic group) or the person’s usual environment (e.g., child abuse). For ethical and practical reasons, many aspects of the environment (child abuse) cannot be manipulated or given and are thus attribute variables. This distinction between active and attribute independent variables is important for determining what can be said about cause and effect. Research where an apparent intervention is studied after the fact is sometimes called ex post facto. We consider the independent variables in such studies to be attributes.
Dependent Variables
The dependent variable is the outcome or criterion. It is assumed to measure or assess the effect of the independent variable. Dependent variables are scores from a test, ratings on questionnaires, or readings from instruments (electrocardiogram). It is common for a study to have several dependent variables (performance and satisfaction).
Extraneous Variables
These are variables that are not of interest in a particular study but could influence the dependent variable and need to be controlled. Environmental factors, other attributes of the participants, and characteristics of the investigator are possible extraneous variables.
Levels of a Variable
The word level is commonly used to describe the values of an independent variable. This does not necessarily imply that the values are ordered. If an investigator was interested in comparing two different treatments and a no-treatment control group, the study has one independent variable, treatment type, with three levels, the two treatment conditions and the control condition.
We have tried to be consistent and clear about the terms we use; unfortunately, there is not one agreed-upon term for many research and statistical concepts. At the end of most sections, we have included a table similar to Table 2.1.1.1 that lists a number of key terms used in this chapter alongside other terms for essentially the same concept used by some other researchers. In addition to these tables of different terms for the same concept, we have appended a list of partially similar terms or phrases (such as independent variable vs. independent samples) which do not have the same meaning and should be differentiated.
TABLE 2.1.1.1 SIMILAR RELATED TERMS ABOUT VARIABLES | ||
---|---|---|
|
Measurement and Descriptive Statistics
Measurement
Measurement is introduced when variables are translated into labels (categories) or numbers. For statistical purposes, we
and many statisticians (2) do not find the traditional scales of measurement (nominal, ordinal, interval, or ratio) useful. We prefer the following: a) dichotomous or binary (a variable having only two values or levels), b) nominal (a categorical variable with three or more values that are not ordered), c) ordinal (a variable with three or more values that are ordered, but not normally distributed), and d) normally distributed (an ordered variable with a distribution that is approximately normal [bell-shaped] in the population sampled). This measurement classification is similar to one proposed by Helena Chmura Kraemer (personal communication, March 16, 1999).
and many statisticians (2) do not find the traditional scales of measurement (nominal, ordinal, interval, or ratio) useful. We prefer the following: a) dichotomous or binary (a variable having only two values or levels), b) nominal (a categorical variable with three or more values that are not ordered), c) ordinal (a variable with three or more values that are ordered, but not normally distributed), and d) normally distributed (an ordered variable with a distribution that is approximately normal [bell-shaped] in the population sampled). This measurement classification is similar to one proposed by Helena Chmura Kraemer (personal communication, March 16, 1999).
Descriptive Statistics and Plots
Researchers use descriptive statistics to summarize the data from their samples in terms of frequency, central tendency, and variability. Inferential statistics, on the other hand, are used to make inferences from the sample to the population.
Central Tendency
The three main measures of the center of a distribution are mean, median, and mode (the most frequent score). The mean or arithmetic average takes into account all of the available information in computing the central tendency of a frequency distribution; thus, it is the statistic of choice if the data are normally distributed. The median or middle score is the appropriate measure of central tendency for ordinal-level data.
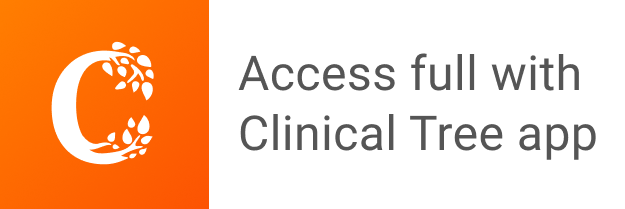