4 Direct Functional Mapping Using Radiographic Methods (fMRI and DTI)
Abstract
Methods for noninvasively mapping neuronal activity (e.g., functional magnetic resonance imaging) and white matter connections (e.g., diffusion tensor imaging) have rapidly evolved. These techniques have greatly enhanced our understanding of normal brain function and anatomy. Their use in surgical planning has also had a positive impact on patient outcomes, operative times, and survival; however, these techniques remain subject to several potential pitfalls and limitations. In this chapter, we discuss the basics of these imaging methods and explore their current limitations.
4.1 Functional MRI
The principles underlying the use of magnetic resonance imaging (MRI) to detect intrinsic changes in blood oxygenation related to neuronal activity were first reported in humans in 1991 by Belliveau et al 1 using dynamic susceptibility contrast and followed by the first human report of blood oxygen level dependent (BOLD) imaging in 1992 by Ogawa et al. 2 These early studies highlighted the potential of dynamic MRI in assessing brain activity. Since then, substantial progress has been made in functional neuroimaging, and BOLD imaging has become a standard tool used in understanding brain function in vivo.
4.1.1 Principles
The signal change detected in BOLD functional MRI (fMRI) results from a change in relative concentrations of oxy- and deoxyhemoglobin. At the onset of neuronal activity, local vasodilation that exceeds the increased energy demand results in an effective increase in oxyhemoglobin and a resultant small, 1 to 5%, change in MRI intensity. The physiologic effects are not instantaneous. The time dependency of these effects is modeled by the hemodynamic response function (HRF). 3 In the normal adult brain, oxyhemoglobin change peaks approximately 6 seconds after the onset of neuronal activity and does not return to baseline until nearly 16 seconds after the initial neuronal onset (Fig. 4‑1). While the exact timing varies slightly across brain regions and subjects, this “double-gamma” function generally applies. Unfortunately, in the setting of brain pathology, this model may be inaccurate, or the HRF may not exist at all. This breakdown of a normal blood flow response to neuronal activity is termed “neurovascular uncoupling” (NVU) and is a major limitation of current clinical fMRI applications. 4

4.1.2 Task-Based Functional Magnetic Resonance Imaging
Currently, task-based fMRI is the most commonly applied method of performing functional imaging in the clinical setting. In task-based fMRI, the subject performs various tasks in the MRI scanner intended to elicit a specific brain function (e.g., tapping fingers to elicit hand motor activation). A block task design is most widely utilized and consists of short blocks of the active task (e.g., finger tapping) alternating with a control task (e.g., rest). Since BOLD signal is typically modeled as a linear time-invariant (LTI) system, rapid repetition of the task will scale the measured signal proportionally (e.g., back-to-back finger taps will produce twice the BOLD signal change as a single finger tap); however, the rate of signal rise and decay reaches a steady state at approximately 16 seconds. Therefore, individual blocks in task-based fMRI are typically short, on the order of 20 to 30 seconds. The block design remains a mainstay of clinical practice as it results in a maximum functional signal-to-noise ratio and the most robust activation given this summation of individual HRFs. The most common method of analysis of task-based fMRI is the general linear model (GLM) in which the expected variation in BOLD signal over time is predictable by a convolution of the block design (on and off block pattern) and the hemodynamic response curve (Fig. 4‑2).

4.1.3 Resting-State Functional Magnetic Resonance Imaging
In recent years, resting-state fMRI (rs-fMRI) has grown rapidly in popularity. Slow (< 0.1 Hz) spontaneous oscillations in BOLD signal had been initially considered noise in fMRI until these were first suggested to have a neural origin in a landmark study by Biswal et al in 1995. 5 In this study, Biswal et al were able to show that these seemingly erratic BOLD signal fluctuations in the motor cortex at rest were in close synchrony with the contralateral motor cortex. 5 Since this original paper, numerous resting brain networks have been shown including those in memory, language, executive function, and attention.
As opposed to task-based fMRI, no tasks are performed by the patient while in the scanner. Due to the absence of a task, the analysis of rs-fMRI data presents several major challenges. Recall that in the block design task-based fMRI, the expected BOLD signal change related to the task is already known by the convolution of the block design and the HRF allowing straightforward application of a GLM. In rs-fMRI, spontaneous BOLD signal changes are measured, and there is no preconceived knowledge of the expected time course of signal change (Fig. 4‑3). Thus, analysis of rs-fMRI requires a different mathematical approach. 6 Two of the most common approaches are seed-based analysis (SBA) and independent component analysis (ICA). SBA relies on choosing a reference region in the brain for which the BOLD signal time course can be extracted and used as the basis for a GLM in the remainder of the brain to find areas of similar spontaneous signal change. This is nicely illustrated by the original experiment of Biswal et al where the time course for one motor cortex is used as a model for eliciting the remainder of the motor network. 5 SBA, therefore, relies on an a priori assumption about the network of interest. The SBA approach is often able to be applied to preoperative planning when a normal network seed is readily identified, such as the anatomic reliability of the normal contralateral motor cortex, but it can be problematic when such reliability is absent. For instance, given the lateralization of language networks and variance of functional anatomy relative to anatomical landmarks, choosing an appropriate seed point can be challenging. The ICA approach uses no a priori assumption, but rather decomposes the time course throughout the whole brain into multiple component maps that explain the variance in the data. It is important to note that much of the variance in the time course is explained by phenomena of no interest, such as the cardiac and respiratory cycle, motion, and thermal noise. Therefore, each component identified must then be categorized as a potential brain network versus a signal of no interest. ICA has been shown as potentially reliable for use in preoperative identification of brain networks; however, questions remain to be answered including the ideal number of components and the ideal way of identifying relevant component maps. 6

Although seemingly easier to perform than task-based fMRI, rs-fMRI presents several additional challenges. First, the true origin of these resting BOLD signal fluctuations is not known. Recent evidence does support a neural origin; however, the lack of understanding of their origin means limited insight into the effects of brain pathology. Second, NVU has also been demonstrated in rs-fMRI, but the relationship of NVU with resting BOLD fluctuations is not entirely clear. 7 Last, in task-based fMRI, the extended experimental blocks of 20 to 30 seconds allow increased ability to remove sources of higher frequency noise (such as cardiac and respiratory signal) with the simple application on a bandpass filter. Unfortunately, the BOLD signal change of interest in rs-fMRI lies in the same frequency range as do these sources of noise and unwanted signal. Therefore, separation of true signal from background is more challenging in rs-fMRI. In summary, rs-fMRI provides a unique method of identifying brain networks and is likely applicable to a greater number of patients (including pediatric patients, patients with cognitive impairment, etc.); however, many unique challenges of rs-fMRI have prevented its widespread use in presurgical planning, to date.
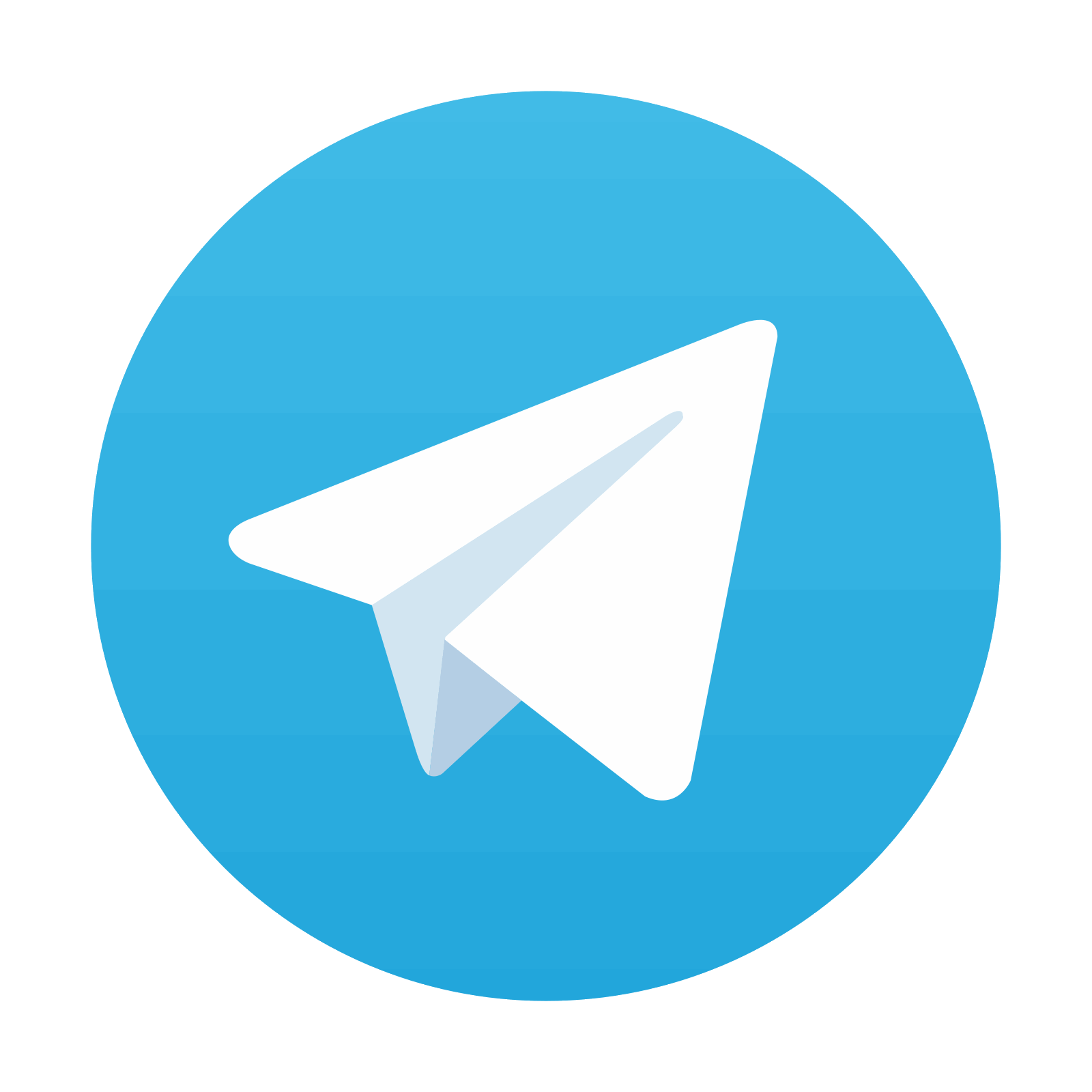
Stay updated, free articles. Join our Telegram channel

Full access? Get Clinical Tree
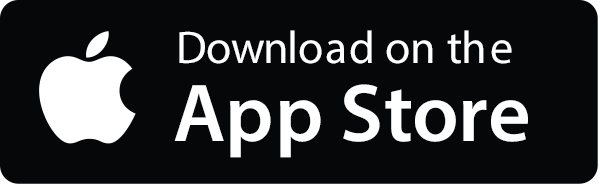

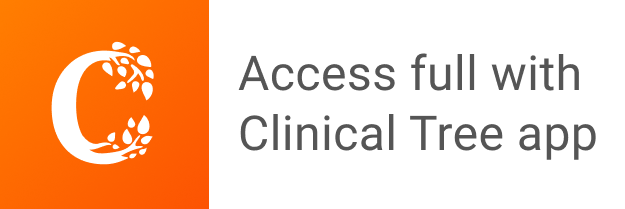