Fig. 1
Complex interactions of the master circadian oscillator in the suprachiasmatic nuclei (SCN), neuromodulators (e.g. orexin/hypocretin Ox/Hcrt; monoamines MA), sleep and psychiatric disorders. Circle: master circadian oscillator; Oval: neuromodulators; Snip single corner rectangle: psychiatric disorders; Rectangle: sleep; Solid arrows: known interactions; Dashed arrows: tentative interactions
The organization of the chapter is as follows. The role of monoamines and orexin in psychiatric disorders are first discussed, and then the role of orexin along with other neuromodulators in the regulation of the sleep-wake cycle will be discussed. This is followed by a discussion of the regulatory functions of the main circadian pacemaker in the suprachiasmatic nuclei (SCN) which coordinates and drives daily activity in other semi-autonomous oscillators in the brain (such as lateral habenula, LHb) and controls circadian timing in some neuromodulatory systems (orexin, monoamines) and sleep. Then a computational modelling perspective will be presented. Finally, there will be a discussion on key unanswered questions and identification of future research directions.
2 Neuromodulators and Psychiatric Disorders
Results from many studies have indicated that altered circadian rhythms, disrupted sleep and abnormal basal levels of neuromodulators are associated with the clinical status of patients experiencing depression (Wehr et al. 1983; Ruhe et al. 2007; Wirz-Justice 2008; Germain and Kupfer 2008). Many of these characteristics are captured by the monoamine imbalance hypothesis which posits that perturbation in the basal levels of monoamines such as serotonin (5-HT), norepinephrine/noradrenaline (NE/NA), and dopamine (DA) can trigger depression (Nemeroff 1998). These monoamines are mainly synthesized at different brain regions. For example, 5-HT is synthesized in the raphe nuclei, NE in the locus coeruleus (LC), and DA in the substantia nigra and ventral tegmental area (VTA) (Grzanna and Molliver 1980; Park et al. 1999; Anzalone et al. 2012). These monoamine producing neurons project to many parts of the brain and are involved in the regulation of a wide range of behaviours (Leibowitz and Shor-Posner 1986; Cools et al. 2008; Aston-Jones et al. 2000; Bromberg-Martin et al. 2010). To understand how alteration in monoaminergic levels influences depression, many studies have focused on the expression and functionality of the monoaminergic receptors and transporters at the source and projection sites (Klimek et al. 1997; Rajkowska 2000; Arango et al. 2001; Boldrini et al. 2005). For example, depressed subjects have decreased 5-HT transporter, altered 5-HT1A/2A binding sites, and lower level of plasma tryptophan and metabolites (Quintana 1992; Owens and Nemeroff 1994; Malison et al. 1998; Drevets et al. 1999; Mintun et al. 2004). In the case of NE, altered adrenoreceptor (α2) densities and lower NE levels have been observed in brain sites innervated by NE neurons, including the cortex (Ordway et al. 2003; Valdizán et al. 2010; Lanni et al. 2009; Moret and Briley 2011). Such changes may arise from reduced NE innervation, reduction in NE transporter activity in NE-containing terminals, or by a combination of both (Klimek et al. 1997). For DA, lower transporter binding potential (a correlate of receptor density) in striatum is reported in depressed patients (Meyer et al. 2001). Similar findings were also observed in depressed patients with anhedonia symptoms (Sarchiapone et al. 2006). However, there are inconsistencies between different studies (Laasonen-Balk et al. 1999). Similarly, investigations have also measured DA metabolites in the CSF, but these results are not necessarily conclusive (Dunlop and Nemeroff 2007).
Despite the inconsistencies, such findings have led to the development of pharmaceutical treatments of unipolar depression. Many antidepressant compounds are designed to increase monoamine levels in the central nervous system (CNS). The strategy for drug treatments for other disorders can vary since the symptomatology can be diverse and dynamic (Bowden 2005; Walderhaug et al. 2011). Current research focuses on other novel treatment options include the exploration of peptidergic ligands. Clinical and preclinical studies are suggestive of the roles of neuropeptide mechanisms (e.g. orexin, vasopressin, galanin, corticotropin-releasing hormone, neuropeptide Y, relaxin-3 and substance P) in the pathophysiology of psychiatric disorders, including depression (Den Boer 2006; Madaan and Wilson 2009; Nollet and Leman 2013). Indeed, emerging drug targets for the treatment of psychiatric disorders are compounds that signal via neuropeptide receptors (Fang et al. 2014; Smith et al. 2014).
Among the neuropeptides potentially involved in the symptomatology of psychiatric disorders are the orexins (Ox; also called the hypocretins or hcrt). These neuropeptides occur in two forms, Ox-A and Ox-B, and are produced by neurons in the lateral, perifornical and dorsomedial areas of the hypothalamus (Sakurai et al. 1998; de Lecea et al. 1998). These Ox-containing neurons send projections to many brain regions (Peyron et al. 1998), including key regions that regulate arousal and motivational states such as the dorsal raphe nucleus (DRN), LC, arcuate nucleus, VTA, tuberomammillary nucleus (TMN), basal forebrain (BF) and laterodorsal and pedunculopontine tegmental nucleus (LDT/PPT) (Tsujino and Sakurai 2009). Through these and other neural connections, Ox play key roles in the regulation of important behaviours and physiological functions such as sleep-wake cycle, energy homeostasis, addiction, endocrine function, reward seeking, and emotional behaviour (de Lecea et al. 2006; Sakurai 2006, 2007, 2010, 2014; Tsujino and Sakurai 2009; Aston-Jones et al. 2009; López et al. 2010). Interestingly, many of these are disrupted in depression (Drevets 2001), suggesting that insights into Ox signaling may lead to a better understanding of the biological basis of depression. Indeed, in the last few years, there have been many studies in humans and animals investigating the role of Ox in depression as well as in other psychiatric disorders.
For example, it has been known that Ox levels can exhibit circadian variation and depressed patients manifest lower amplitude circadian variation in Ox levels in the cerebrospinal fluid (CSF) than do control individuals. Treatment with sertraline (a selective serotonin reuptake inhibitor (SSRI) anti-depressant) decreased mean Ox levels, suggesting that elevated Ox signaling contributes to the depressive symptoms (Salomon et al. 2003). Such a putative interaction appears complex since other studies have reported that following attempted suicide the CSF Ox levels are decreased (Brundin et al. 2007a, b). In a later follow-up study (6–12 months after the attempt), CSF Ox levels among suicide survivors were relatively high compared to those measured immediately following suicide attempt (Brundin et al. 2009). In another investigation, Ox levels were reportedly unaltered in manic, control and depressed patients (Schmidt et al. 2010). Thus the effects of Ox in depression in humans remain inconclusive.
The relationship between Ox and depression has been also explored in animal models. For example, in the Flinders Sensitive Line (FSL), a rat genetic model of depression in which the animals are more susceptible to cholinergic agonists, the number of Ox neurons are higher than in control rats (Overstreet 1986; Overstreet et al. 2005; Mikrouli et al. 2011). Similarly, a 20 % increase in the number of detectable Ox neurons in lateral hypothalamus area was recently reported in a rodent model where high dosage of corticosterone treatment results in depressive like symptoms (Jalewa et al. 2014b). These findings suggest that during depression, either the number of Ox neurons increases or Ox synthesis increases, such that Ox-containing neurons are more readily available in the hypothalamus. In support for this interpretation, central infusion of Ox stimulates the hypothalamic–pituitary–adrenal (HPA) axis (Kuru et al. 2000), and hyperactivity of the HPA axis is often associated with depression (Vreeburg et al. 2009). Interestingly, attenuating Ox signaling in rodents through treatment with the Ox receptor antagonist almorexant improves HPA functioning and decreases behavioural measures of depression (Nollet et al. 2012). In contrast, exogenous treatments with Ox-A can have anti-depressant properties (Ito et al. 2008), suggesting that reduced Ox levels are associated with depression.
Ox is also potentially involved in other psychiatric disorders. For example, Ox signaling influences attentional and cognitive activities and has been linked with schizophrenia (Deutch et al. 2005; Lambe et al. 2007; Fukunaka et al. 2007; Poirier et al. 2010). Interestingly, higher plasma Ox levels are associated with improved symptoms in schizophrenia (Chien et al. 2015). There is also robust evidence indicating that a reduction in Ox neurons can lead to narcolepsy (Lin et al. 1999; Chemelli et al. 1999; Peyron et al. 2000). Since psychiatric patients frequently develop sleep disorders, this raises the possibility that changes in Ox signaling contributes to such arousal and wake-rest disruptions. This is explored in the next section.
3 Neuromodulators and Sleep
In mice, direct injection of Ox-A into the brain promotes wakefulness and suppresses non-rapid eye movement (NREM) and rapid eye movement (REM) sleep (Mieda et al. 2011), while similar treatment with a dual Ox1/Ox2 receptor antagonist promotes sleep and reduces locomotion (Mang et al. 2012). Thus, the activity of Ox neurons and Ox release varies across the sleep-wake cycle. Indeed, during the wake state, Ox neurons in rodents are very active (Lee et al. 2005b; Marston et al. 2008; Estabrooke et al. 2001), coinciding with the time when Ox release is maximal (Deboer et al. 2004). As noted earlier, Ox neurons innervate arousal-promoting nuclei in the pons, midbrain, posterior hypothalamus and forebrain, and since the main action of Ox is excitatory, it is interpreted that Ox activates neurons in these nuclei to drive wakefulness. Such neurons are major sources of acetylcholine, histamine, serotonin, and norepinephrine, all of which are known to contribute to arousal states. Indeed, the activity of these neurochemically identified neurons is higher during wakefulness than in sleep. Thus, during wakefulness, the outputs of arousal-promoting neurons act to inhibit the activity of NREM and REM promoting neurons in ventrolateral preoptic nucleus (VLPO) and LDT/PPT, respectively, and relay excitatory signals to the thalamus and cortical neurons (Saper et al. 2001; España and Scammell 2011).
During NREM sleep, neurons of the preoptic areas are activated, and these neurons act to inhibit the wake and REM-promoting neurons elsewhere in the brain. Thus, during this time, the firing in monoaminergic neurons decreases significantly and ceases completely in the cholinergic and Ox neurons (Saper et al. 2001; España and Scammell 2011). In addition to the preoptic neurons, interneurons (mainly GABAergic) in some of the arousal areas (LHA and BF) are also active during NREM phase and contribute in conveying inhibitory signals to the cortex to promote slow wave sleep (Manns et al. 2000; Hassani et al. 2009, 2010).
REM sleep occurs during the transition from NREM to waking states (Saper et al. 2001). Hallmark features of REM sleep include cortical activation and loss of muscle tone. Interestingly, during REM sleep, Ox neurons are not completely silenced but instead contribute to the phasic components of this brain state (Torterolo and Chase 2014). By contrast, during REM, cholinergic and VLPO neurons show high firing rate, while monoaminergic neurons cease firing (España and Scammell 2011). Thus an imbalance between cholinergic and monoaminergic discharge activity may contribute to the disruption between REM and NREM sleep which is very common among depression sufferers. Moreover, an imbalance between Ox and monoaminergic activity is believed to be a key factor in narcolepsy and depression (Brown et al. 2001; Feng et al. 2008).
These interpretations can be better understood by considering the two-process model of the dynamic regulation of sleep-wake cycle (Borbély 1982; Daan et al. 1984). It is widely believed that this regulation is achieved via two separate biological processes where process S is responsible for sleep homeostasis, and process C controls the circadian timing (via the circadian clock) (Fig. 2). Accordingly, the increase in sleep pressure that occurs during wakefulness subsequently declines during sleep. Studies have linked this pressure with extracellular adenosine levels (Sims et al. 2013; Huang et al. 2014). Adenosine forms from the degradation of adenine nucleotides, and during wakefulness its levels are believed to be increased in the BF and cortical regions (Huang et al. 2014). Thus, depending upon the adenosine concentration, process S sends timing signals to process C and regulates the sleep-wake cycle. So, the circadian clock not just controls the timing of process S but also interacts with various sleep stages, which may well decide the recovery pattern in instances of sleep deprivation. Interestingly, a study shows that activity of neurons in the brain’s master circadian clock in the suprachiasmatic nuclei (SCN) is higher during the REM and wake states, and relatively lower during the NREM state (Deboer et al. 2003). There is further evidence that suggests that mutation, polymorphism or deletion of some of the clock genes disrupt sleep homeostasis and response to the sleep deprivation (Naylor et al. 2000; Toh et al. 2001; Laposky et al. 2005; Dijk and Lockley 2002). However, how changes in sleep-wake patterns affect the molecular mechanism of the clock still remains unknown (Deboer et al. 2003).
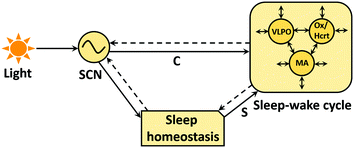
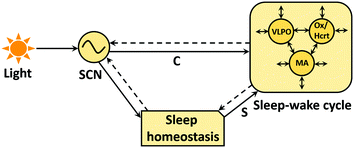
Fig. 2
A two-process model of sleep. The circadian clock in the SCN receives light inputs and sends the timing signals (C) to regions of the brain involved in sleep-wake (S-W) cycle and to a conceptual sleep homeostasis process which further regulates the balance between sleep and wake state. The S-W cycle is controlled by the complex interactions between sleep (ventrolateral preoptic nucleus VLPO) and wake promoting neurons (MA, Ox). Circle: circadian oscillator, sleep and wake promoting areas; Snip single corner rectangle: sleep homeostasis; Solid and dashed arrows as in Fig. 1 (Borbély 1982; Daan et al. 1984; Dijk and Lockley 2002)
In the next section, we shall discuss how the circadian pacemaker in the SCN drives daily timing in different brain areas and regulates neuromodulator levels and sleep. We will also discuss how disruption of circadian clock timing is linked to specific symptoms commonly observed in psychiatric disorders.
4 Circadian Rhythms
Circadian rhythms are intrinsic near 24 h oscillations and pervade all aspects of physiology and behaviour. Neurons of the SCN contain the intracellular circadian clock of which the Period (Per1–2), Cryptochrome (Cry1–2), Circadian Locomotor Output Cycles Kaput (CLOCK) and Brain and Muscle ARNT-like protein 1 (Bmal1) genes and their protein products play important roles (Mohawk and Takahashi 2011). The synchronized activity of these autonomous cellular clocks enables the SCN as a whole to function as the master clock. In turn, the SCN receives information about environmental lighting directly from the retina, with daily variation in light entraining the SCN to the external world (Fig. 2). The SCN output then communicates these integrated timekeeping signals to the rest of the brain and body to control daily rhythms in sleeping and waking, metabolism, cognition, and mood (Piggins and Guilding 2011).
Intriguingly, as noted earlier, in many psychiatric conditions, alterations in sleeping and waking as well as mood are frequently present as part of the patients’ symptoms, suggesting that SCN or SCN-regulated processes are disrupted in such disorders (Wulff et al. 2010; Menet and Rosbash 2011; McCarthy and Welsh 2012; Schnell et al. 2014; Gonzalez 2014). Indeed, in mice, genetic alterations that affect components of the intracellular molecular clock are implicated as murine models of human psychiatric conditions (Landgraf et al. 2014). For example, the Δ19 mutation of the clock gene, results in a dysfunctional CLOCK protein and the mouse manifests >24 h rhythms or lacks circadian rhythms as well as hyperactivity and other hallmarks of mania (Vitaterna et al. 1994; McClung et al. 2005; Roybal et al. 2007; Sidor et al. 2015). Such a mutation directly affects the SCN clock, but since circadian clock genes are also expressed in other brain areas as well as peripheral tissues, local physiology can be affected in such regions and organs. In the case of the Δ19 clock mutant mouse, mania-like behaviours are attributable to dysfunction of dopamine neurons in the VTA. This appears to be due to changes in gene regulation normally under the control of CLOCK (Mukherjee et al. 2010). Another mutation that influences the stability of CRY proteins also lengthens the period of circadian rhythms, which is accompanied by changes in anxiety (Keers et al. 2012).
In humans, some mutations in clock genes are associated with the sleep-wake cycle. Of note, treatment of bipolar disorder can include lithium which exerts some of its actions via GSK-3beta, a key regulator of the intracellular molecular clock (Iitaka et al. 2005). Indeed, in animal studies, lithium lengthens the period of the molecular clock rhythms and locomotor behavior (Welsh and Moore-Ede 1990; Li et al. 2012).
In rodents the activity of Ox neurons is under SCN circadian control (Zhang et al. 2004), with higher activity occurring during the behaviourally-active circadian night (Estabrooke et al. 2001; Marston et al. 2008). At this phase, Ox release in the brain is also at maximal (Deboer et al. 2004). Experimental destruction of the SCN or exposure to constant light, which attenuates SCN output, abolishes circadian variation in CSF levels of Ox, indicating that the day-night activity profile of this key arousal-promoting neurochemical is under SCN output control. Interestingly, the phase of the SCN circadian clockwork is also sensitive to feedback actions of arousal-promoting stimuli, particularly during the behaviourally-inactive circadian day (Hughes and Piggins 2012). During the day, arousal-promoting stimuli activate Ox neurons (Estabrooke et al. 2001; Marston et al. 2008; Webb et al. 2008) and suppress the SCN’s electrical activity (van Oosterhout et al. 2012). Indeed, in SCN brain slice preparations Ox not only suppresses the electrical activity of SCN neurons, but also potentiates the phase-shifting capacity of another neurochemical correlate of arousal, neuropeptide Y (Belle et al. 2014).
Hence, alterations in the SCN circadian clock as well as circadian regulation of the brain and behavioural states can result in symptoms seen in psychiatric conditions. Since Ox-containing neurons project widely across the brain (Peyron et al. 1998; Nambu et al. 1999), including structures of the neural circadian system (McGranaghan and Piggins 2001; Backberg et al. 2002), Ox released during states of arousal can influence circadian timing in SCN and extra-SCN brain sites (Fig. 3). This may be particularly important in psychiatric conditions when the pattern of sleeping and waking is severely disturbed. Incorporating such knowledge when considering the treatments and the timing of treatments, therefore, has considerable therapeutic potential.
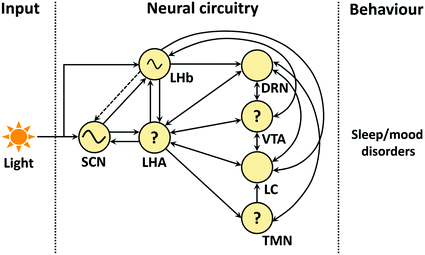
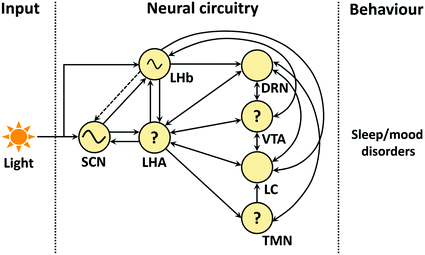
Fig. 3
Circadian clock, neuromodulators and their possible role in the etiology of sleep and mood disorders. Suprachiasmatic nuclei (SCN) with lateral habenula (LHb) receive the light inputs and regulate the activity of other brain areas that are important in S-W and mood regulation. SCN, LHb: master and semi-autonomous clocks. Lateral hypothalamus area (LHA), dorsal raphe nucleus (DRN), ventral tegmental area (VTA), locus coeruleus (LC), and tuberomamillary nucleus (TMN): major source of orexin/hypocretin, serotonin, dopamine, norepinephrine and histamine, respectively. ‘?’: Either clock gene(s) are present in these areas (existence of intrinsic activity not yet confirmed) or their daily activity is indirectly regulated by the circadian clocks present in other brain regions (e.g. SCN and/or LHb). Circle: circadian oscillators (SCN, LHb), LHA and monoaminergic areas; Note: non-photic inputs are not shown (Jones and Moore 1977; Herkenham and Nauta 1979; Segal 1979; Deutch et al. 1986; Kalen et al. 1988; Ericson et al. 1989; Herkenham and Nauta 1993; Peyron et al. 1998; Nambu et al. 1999; Abrahamson et al. 2001; Yoshida et al. 2001; Kim et al. 2004; Mileykovskiy et al. 2005; Lee et al. 2005a; McClung et al. 2005; Hattar et al. 2006; Guilding and Piggins 2007; Vertes and Linley 2008; Omelchenko et al. 2009; Tsujino and Sakurai, 2009; Schwartz et al. 2011; Goncalves et al. 2012; Watabe-Uchida et al. 2012; Stamatakis et al. 2013; Belle et al. 2014; Dorocic et al. 2014; Proulx et al. 2014; Root et al. 2014; Sakhi et al. 2014; Yu et al. 2014; Ogawa et al. 2014)
Thus, mutual interactions between circadian clock, neuromodulators and sleep are likely to be important in understanding the biological mechanisms of some aspects of psychiatric disorders. However, the behaviours due to these interactions are not intuitive. For example, 5-HT neurons in the DRN receive inputs from other monoaminergic neurons including the NE-containing neurons of the LC, histamine-containing neurons from TMN, as do DA neurons of the VTA (Kalen et al. 1988; Lee et al. 2005a; Dorocic et al. 2014; Ogawa et al. 2014). Additionally, the activities of these neuromodulators are also regulated by their auto-receptors. Thus, it is very difficult to assess the causal relationship (between activities or firing rate) among the different brain areas to establish precisely how these changes can cause or affect the severity of a symptom.
A promising approach towards assessing and understanding such complex interacting systems is to develop, simulate and analyze computational models. Some initial cognitive computational models have focused on improving the diagnosis of psychiatric disorders (Siegle 1999; Siegle and Hasselmo 2002). More recent cognitive computational models have focused on decision-making, reward and reinforcement learning aspects, e.g. learned helplessness and anhedonia, which are the core symptoms in depression (Dayan and Huys 2008; Gradin et al. 2011; Huys et al. 2013). However, these models are based on high-level abstractions and hence do not readily illuminate the underlying biological mechanisms underpinning the disorders. Moreover, there is a lack of modelling on the interactions between Ox and other neuromodulators, and their effects on sleep and depression. This is not surprising as substantial modelling and analysis work was more focused on associating Ox with the sleep-wake cycle, and in particular, its role in narcolepsy [e.g. (Behn et al. 2010)]. In the next section, we will briefly review some biologically based computational modelling studies on Ox, monoamines, circadian rhythms, and sleep-wake dynamics.
5 Neural Computational Models
The mathematical modelling and analysis of sleep-wake dynamics and circadian rhythms have a relatively long history, primarily due to the availability of behavioural and physiological (e.g. temperature and neuroendocrine) data (Czeisler 1978; Czeisler et al. 1980; Daan et al. 1984; Kawato et al. 1982; Kronauer et al. 1982, 1983; Strogatz and Carpenter 1986; Strogatz 1987; Winfree 1983). All these models address the sleep-wake dynamics and various autonomic circadian rhythms. There is a considerable similarity among these models (Kronauer et al. 1982; Kronauer et al. 1983), which typically consist of homogeneous neuronal populations, or coarse-grained population-averaged (firing-rate) type models (Wilson and Cowan 1972). The simplicity of the models allows tractability in the mathematical analyses and provides conceptual insights.
As various types of biological data become available, models of circadian rhythms become more physiologically detailed. At the molecular, genetic and protein levels, biochemical reactions and feedback loops are modelled to understand their regulations on the intrinsic circadian rhythms and their perturbations (Goldbeter 1995; Leloup and Goldbeter 2003; Forger and Peskin 2003). Physiologically, more realistic neuronal models of sleep-wake regulation have also begun, building on previously more abstract mathematical models (Behn et al. 2007; Phillips and Robinson 2007; Booth and Behn 2014). These models are typically considered at a single level of biological details.
At the neuronal level, a biophysical conductance-based model of the SCN neurons has successfully incorporated the available neuronal and synaptic properties in the SCN and can mimic the neuronal firing patterns, which are consistent with experiments (Sim and Forger 2007). Similar biophysical models for Ox neurons has also been developed with the minimal set of currents (Postnova et al. 2009; Carter et al. 2012) and model parameters were based on other neuronal types (Williams and Behn 2011). Despite the assumptions, such models could account for some aspects of sleep-wake transitions (Postnova et al. 2009), and the dynamical effects of Ox and dynorphin, a colocalized neuropeptide, on the Ox neurons (Williams and Behn 2011). In Carter et al. (2012), a more biophysical two-compartmental model of Ox neuron was also developed.
Neurocomputational models at the microcircuit level may offer a way to investigate heterogeneity effects and emergent properties, which are not feasible at the single neuronal modelling level. For example, Postnova et al. (2009) incorporated local glutamatergic neurons to provide local feedback to the Ox neurons to understand homeostasis in sleep-wake transitions. Then, Patriarca et al. (2012) extended this model into a multi-neuron model and demonstrated that sufficient diversity in the synapses is needed to provide efficient functioning of the homeostasis regulation of the sleep-wake cycle. In Gonze et al. (2005) the model incorporated coupling among 10,000 circadian oscillators and found that spontaneous synchronization is achieved through the “mean-field” coupling and the population can be entrained with light pulses. In Wong-Lin et al. (2012), a microcircuit model of the DRN with non-5-HT GABAergic neurons was proposed to link from single neuronal spiking behaviours of 5-HT and local non-5-HT neurons in the DRN to phasic neuronal activity observed in behaving animals. The microcircuit DRN model suggested inputs via inhibitory neurons, and predicted low frequency oscillations in the network, which is clearly emergent network behaviour.
To understand the overall behaviour of a system as complex as that shown in Fig. 3, one would need to extend beyond microcircuit modelling levels, and towards larger circuit models that include the interactions of multiple brain regions (Sorooshyari et al. 2015). For example, Behn et al. (2007) modelled the interactions among the sleep active (VLPO), wake active (LC, DR, TMN) and REM active (LDT/PPT) neural populations. The model could capture some of the features of mouse the sleep-wake behaviour (such as short-term awakening), and also predicts the mechanism for state transitions. Subsequent modelling studies showed that Ox could play an important part in the sustenance and stabilization of prolonged episodes of wake and sleep (Behn et al. 2008; Fulcher et al. 2014). Similarly, the role of Ox is also analyzed for other large-scale modelling work that reasonably reproduce sleep-wake timings (under normal and sleep deprived conditions), circadian influence on total sleep time, and rapid transition between sleep and wake states with the loss of Ox (Rempe et al. 2010).
Recently, there has been considerable interest in more focused modelling of Ox system’s interactions with that of the monoamines (Joshi et al. 2011; Carter et al. 2012; Jalewa et al. 2014a). A common aim of these studies is to understand the possible roles of such interactions in sleep and depression (Joshi et al. 2011; Carter et al. 2012; Jalewa et al. 2014a; Mosqueiro et al. 2014). In one of these studies, the excitability of LC neurons is shown to be important for Ox-mediated transition in sleep-to-wake transition (Carter et al. 2012). In a different study, a mathematical neural circuit model with direct interactions between the DRN and LHA is developed (Joshi et al. 2011). The model’s novel input-output functions for the DRN and LHA areas are derived from the relationship between the neural firing rate in that area and neuromodulator concentration level, which can be obtained directly from experiments. In addition, the dynamics of the release and uptake of the neuromodulators can, in principle, also be measured from experiments, e.g. voltammetry.
Even more complex models have been studied, where the models include not just across-region interactions, but also interactions with other local interneurons. For example, in Mosqueiro et al. (2014), inhibitory GABAergic interneurons are used to investigate the regulation of NE-producing LC neurons by Ox, in which the GABAergic neurons provide an indirect inhibitory connection from Ox to LC. This modelling work shows that the relatively fast GABAA-mediated synapses are not sufficient to regulate LC activity. Similarly, the model in Jalewa et al. (2014a) has included more explicit interneurons, and for the first time, autoreceptors were included for the LHA-DRN interaction. More importantly, indirect connections are also considered in this model. The model demonstrates that LHA-DRN interactions are more stable if the indirect connections from 5-HT to GABAergic neurons in the LHA are strongly excitatory. Furthermore, it is found that faster 5-HT (e.g. via the 3A) receptor mediated timescales can quickly reset the Ox neuronal activities to baseline firing rate right after phasic 5-HT activation. Further, Kumar et al. (2012) have developed a mathematical model of REM-NREM dynamics that includes local GABAergic neurons, revealing the sensitive control of Ox on REM dynamics.
Taken together, these modeling studies have furthered our understanding of the relationship between Ox and the monoamines, and their roles in sleep-wake dynamics, providing mechanistic links to mood, cognition and psychiatric disorder. Despite the progress, integrated computational models that can link from cellular to behavioural levels have yet to be developed. Having such models would be of tremendous help in guiding future experiments across various levels.
6 Discussion
Many people worldwide suffer from psychiatric disorders. Depression is one psychiatric disorder with complex etiology and heterogeneous symptoms (Krishnan and Nestler 2008). Sleep anomalies and altered circadian rhythms are prevalent in depression (Germain and Kupfer 2008; Pandi-Perumal et al. 2009). Monoamine oxidase inhibitors (MAOI) are used for the clinical treatment of this atypical disorder (Thase et al. 1995) and are now augmented by drugs that target neuropeptide receptors, thought to be important in the etiology of depression (Saar et al. 2013; Nollet and Leman 2013; Yeoh et al. 2014).
In this chapter, we have discussed how Ox can interact with monoamines, circadian oscillators (e.g. SCN), and sleep wake-cycle. We raised some key issues, identified some of the unknown connections and attempted to understand how anomaly in one of the system can influence their complex interactions, which may contribute to certain behaviours that are common in psychiatric disorders.
Ox is a key neuropeptide which plays important roles in the regulation of a wide range of behaviours. Interestingly, Ox levels were inconsistent in different preclinical and clinical studies. There can be many reasons for such inconsistencies. For example, Ox levels are regulated by the circadian clock and if not sampled at the same time in each study, considerable interstudy differences could emerge. Indeed, one investigation showed that depressed patients have dampened CSF Ox rhythms (Salomon et al. 2003). Similarly, circadian variation in serum serotonin and metabolites is also reported in depression (Pietraszek et al. 1991). Not surprisingly, there are other factors that may also contribute to inconsistency in measured brain neuromodulator levels. Some of them include heterogeneity among the animals (e.g. mice, rats), experimental protocols, small sample size and methods for measuring the neuromodulator levels (e.g. voltammetry, high-performance liquid chromatography or radioimmunoassay).
Another important issue is to conside whether Ox and other neuromodulator levels are regulated reciprocally. The last few years have witnessed a surge of interest in understanding Ox interactions with monoamines as these interactions can play important roles in the regulation of cognitive functions altered in many psychiatric disorders. Ox interaction with DA neurons may contribute to the better understanding of sleep related problems reported in schizophrenia, as anti-dopaminergic drugs actively lower the Ox CSF levels and promotes the NREM sleep in schizophrenics (Dalal et al. 2003). Also co-release of Ox with dynorphin in the VTA is important in motivational and reward-related behaviours (Muschamp et al. 2014) which are known to be disrupted in depression and schizophrenia. Similarly, knowledge of the interaction among Ox and serotonergic neurons is necessary for the better understanding of depression, as higher Ox levels in the hypothalamus are reported in a clomipramine (SSRI) induced rat model of depression (Feng et al. 2008). In some respects, there is much in common in these Ox-monoamine interactions. All these neuromodulators are active during arousal, and are also involved in some of the cognitive functions. The other similarity between Ox and other neuromodulators is the way Ox connects with them; interestingly Ox shares an excitatory-inhibitory feedback loop circuitry with these neuromodulators (e.g. serotonin, norepinephrine and dopamine). For example, Ox depolarizes these monoamine- containing neurons and conversely these neuromodulators can hyperpolarize Ox neurons in the LHA. Recently, experimental and modeling studies have contributed towards the better understanding of such interactions (Carter et al. 2012; Schone et al. 2014; Jalewa et al. 2014a). However, mutual interaction of all these arousal areas is neglected in the literature. This is because mutual interactions among the monoaminergic neurons add another layer of complexity, and practical implementation of these connections remains a considerable challenge.
Other than these complex interactions, Ox neurons also receive daily timing information from the SCN, and play a key role in the regulation of REM sleep (Kantor et al. 2009). However, the exact pathway via which SCN regulates Ox neurons and REM sleep is not known. One obvious possibility is that SCN and sleep homeostasis processes interact with sleep-wake states in accordance with the two-process model (Fig. 2). The other possibility is that the SCN directly regulates the sleep-wake cycle, and Ox plays a dual role where it stabilizes the sleep-wake switch and also regulates the sleep homeostasis process (Postnova et al. 2009). The excitatory drive of Ox neurons during wakefulness is due in part to excitatory feedback connections between Ox and glutamatergic neurons within the LHA. It is possible that synaptic efficacy of Ox neurons decreases due to their prolonged activity during wakefulness which is recovered during sleep.
Apart from Ox, the SCN clock can also influence the process of sleep homeostasis as this is disrupted in clock mutant mice (Naylor et al. 2000). However the exact relationship between Ox and the sleep homeostasis process remains elusive. Further, recent evidence indicating that clock genes influence the activity of DA neurons adds another level of complexity (McClung et al. 2005). This brings us to a question, whether a disrupted clock can influence the level of Ox or monoamines, and if so then what is the neural pathway of this dysregulation. One possibility is that the master clock in SCN first desynchronizes other local clocks (LHb, paraventricular nucleus) or the areas with clock genes. These areas then indirectly communicates with the other brain areas, affecting the electrical activities of their neurons (Fig. 3). On the other side, a change in the level of Ox or monoamines can alter circadian rhythms in other physiological activity, such as the profile in stress hormones and the HPA axis (Mazzocchi et al. 2001; Spinazzi et al. 2006; Ziolkowska et al. 2005).
Thus the role of Ox is not just limited to the regulation of the sleep-wake states but it is involved in the regulation of a wide range of behaviours and cognitive functions (Mahler et al. 2014). Most of these behaviours are determined by the complex interaction between subcortical and cortical areas (Jankowski and Sesack 2004; Onge et al. 2012; Pujara and Koenigs 2014). However, linking the neuronal activity with the observed behaviour is one of the greatest challenges in neuroscience.
To accomplish this difficult task, one important approach is to collate the experimental data and build computational models across multiple levels, from intracellular processes in individual neurons to network properties and behavioural states. For example, a concerted effort to systematically collect neurobiological data, such as electrophysiological properties of neurons, release-and-reuptake dynamics of the neuromodulators, and receptor-mediated currents at the target sites, would be vital towards developing biologically faithful computational models of neuromodulation. With such data available, an integrated model endowed with multiscales can be developed. The multiscale modelling approach would extract the essence at each scale or level and integrate this with processes happening at other scales or levels (Yamada and Forger 2010; Vasalou and Henson 2010; Qu et al. 2011; Dada and Mendes 2011). Such effort in developing a multiscale framework for neuromodulator systems, from intracellular biochemical reactions to behaviour, is currently undertaken by the authors—(Eckhoff et al. 2009, 2011; Wong-Lin et al. 2012; Wang and Wong-Lin 2013; Laviale et al. 2013; Nakamura and Wong-Lin 2014; Flower and Wong-Lin 2014; Cullen and Wong-Lin 2014). With the availability of such multiscale models, one can rapidly test hypothesis, and can make model predictions that can be verified by future experiments. Thus, computational models with pharmacological, imaging and optogenetic approaches can improve our understanding of the underlying neural mechanism(s) responsible for specific symptoms that are common in psychiatric disorders.
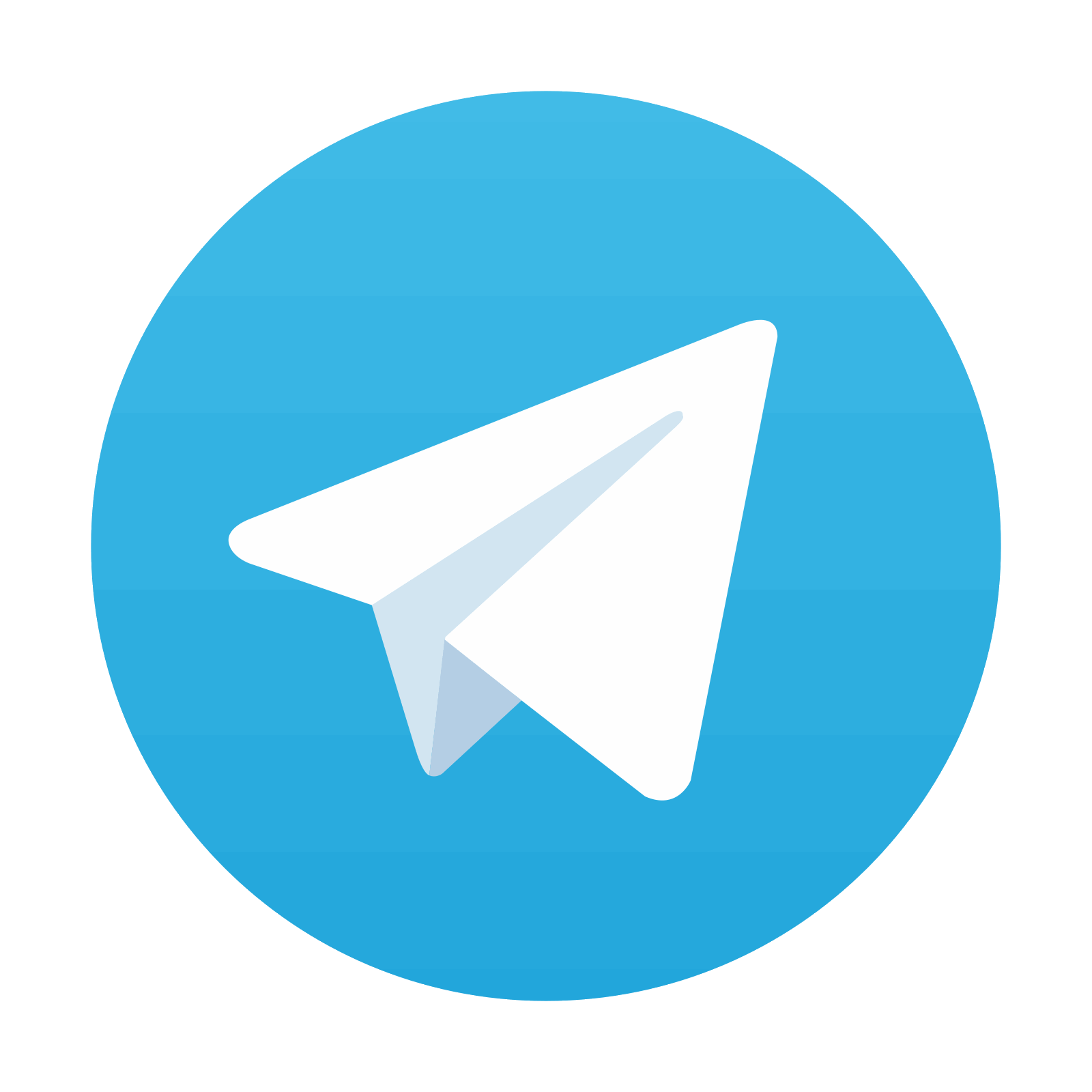
Stay updated, free articles. Join our Telegram channel

Full access? Get Clinical Tree
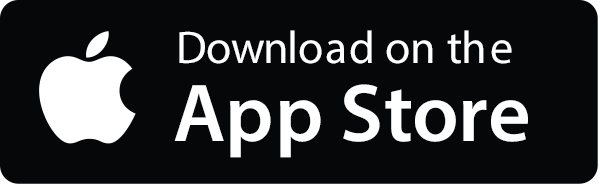
