Abstract
Almost all efficacious stroke treatments confer moderate-to-large benefits, but not staggeringly huge benefits. However, moderate treatment effects can be clinically very worthwhile for the patient. To detect moderate-large treatment benefits, trials must avoid bias and random error. Studies with weak designs (personal experience, observational studies with historical controls, and observational studies with concurrent, non-randomized controls) will not sufficiently control bias and random error to enable reliable discrimination of a true moderate-to-large benefit from false positives and false negatives. Randomized clinical trials are required. ‘Ingredients’ for a good trial – Proper randomization and concealment of allocation (i.e. clinician cannot have foreknowledge of next treatment allocation)/Outcome evaluation blind to the allocated treatment/Analysis by allocated treatment (including all randomized patients: intention-to-treat)/Large numbers of major outcomes and correspondingly narrow CIs/Conclusion based on pre-specified primary hypothesis and outcome/Chief emphasis on findings in overall study population. Advantages of systematic reviews (over traditional unsystematic, narrative reviews) – Use explicit, well-developed methods to reduce bias/Summarize large amounts of data explicitly/Provide all available data/Increase statistical power and precision/Look for consistencies/inconsistencies/Improve generalizability. Cochrane Reviews – Generally higher quality than other systematic reviews/Periodically updated/Available over internet/Abstracts available free of charge/Full reviews available free of charge in over 100 low- and middle-income countries
One of the challenges in finding effective treatments for stroke is that stroke is not a single entity. Stroke has a broad spectrum of clinical features, pathologies, aetiologies, and prognoses. Consequently, there is wide variation in the types of treatments for stroke and in the response of patients to effective treatments. This means that ‘magic bullet’ therapies that treat all types of stroke are likely to be limited in number and effectiveness, confined to aspects of risk factor management to prevent first or recurrent stroke, acute supportive care to prevent early complications, and rehabilitation treatments to promote neuroplasticity and stroke recovery. This diversity is analogous to that seen with infectious diseases and cancers. They also have a broad spectrum of clinical features, pathologies, causes, and outcomes. As a result, there is a range of antibiotic and antineoplastic treatments targeting different aetiologies and mechanisms of cellular injury and, even in targeted patients, their effectiveness is variable. This is because the response of patients is also determined by other genetic and acquired factors.
Given that there are likely to be different treatments for different causes and sequelae of stroke, and different responses in different patients, stroke researchers need ideally to aim to evaluate the effects of treatments for particular pathological and aetiological subtypes and sequelae of stroke, and stroke clinicians need ideally to strive to target effective treatments to appropriate patients who are likely to respond favourably.
Stroke clinicians therefore need to know which treatments for patients with particular types and sequelae of stroke are effective (or ineffective), and their respective risks and costs. Theory alone is insufficient for guiding practice; treatments should have been tested appropriately and thoroughly in clinical practice (Doust and Del Mar, 2004; Ionnadis, 2005; Djulbegovic and Guyatt, 2017). Although appropriate evaluation usually requires enormous efforts and resources, this is several-fold less than the costs of misplaced scepticism, which leads to underuse of effective treatments, and of misplaced enthusiasm, which leads to the introduction of, and perseverance with, ineffective and dangerous treatments. Formal evaluations demonstrating the effectiveness of many therapeutic advances have led to their wide dissemination in practice, such as statins and carotid artery revascularization procedures for stroke prevention and intravenous thrombolytics and endovascular thrombectomy for acute ischaemic stroke (Sarpong and Zuvekas, 2014; Lichtman et al., 2017; Adeoye et al., 2011; George et al., 2019). Conversely, formal trials showing lack of benefit of many physiologically plausible treatments have led to reductions in use of many costly, and sometimes risky, ineffective therapies, such as extracranial–intracranial bypass surgery for atherosclerotic disease and intensive glucose control for acute ischaemic stroke (Johnston et al., 2006; Powers et al., 2011; Johnston et al., 2019). This experience confirms that it is critical to evaluate all potential therapies for stroke with formal controlled clinical trials, and to enrol eligible patients in available trials. Contrary to the commonly expressed notion that it is not ethical to enrol patients in controlled clinical trials in which they might not be allocated to a therapy in which a physician has a strong personal, but unvalidated, belief, moral imperatives support performance and offering enrolment in well-designed clinical trials as the best action that can be taken both for the individual patient being cared for and for all future patients (Ashcroft, 2000; Emanuel et al., 2000; van Gjin, 2005; Lyden et al., 2010).
Indeed, a primary reason for the wide variation in stroke management among different clinics, cities, regions, and countries, and use of ineffective and harmful treatments, is continuing uncertainty about the safety and effectiveness of many of the available treatments due to the lack of reliable evidence of efficacy and safety (Table 2.1) (Chalmers, 2004; Doust and Del Mar, 2004; Ionnadis, 2005). Fewer than 10–25% of practice guideline recommendations in cardiovascular care are supported by high-grade randomized trial evidence (McAlister et al., 2007; Schumacher et al., 2019).
Table 2.1 Reasons for using ineffective or harmful treatments
•Lack of reliable evidence of safety and effectiveness |
•Over-reliance on surrogate outcomes |
•Anecdotal clinical experience |
•Use of historical controls |
•Unsound theoretical/physiological reasoning (e.g. enthusiasm for a particular physiological model, which is incorrect) |
•Dismal natural history of the disease (poor prognosis so unwillingness not to offer some therapy) |
•Patients’ expectations (real or assumed) |
•A desire to ‘do something’ |
•Ritual |
•No questions asked or permitted (‘eminence-based’ rather than ‘evidence-based’ medicine) |
In the presence of systematic uncertainty about the relative intrinsic merits of different treatments, clinicians cannot be sure about their benefits in any particular instance – as in treating an individual patient. Therefore, it seems irrational and unethical to insist one way or another before the completion of a suitable evaluation/trial of the different treatments. Therefore, the best treatment for the patient is to participate in a relevant trial (Ashcroft, 2000; Emanuel et al., 2000; Lyden et al., 2010). Although this is experimentation, it is simply choice under uncertainty, coupled with data collection. The choice is made by random allocation, and constructive doubt is its practical counterpart, but this should not matter as there is no better mechanism for choice under uncertainty. When circumstances increase practitioner unease with random allocation, as when there is strong personal belief in a therapy even though there is community scientific equipoise or when the outcome of standard care is uniformly poor, enrolment into clinical trials can be made more appealing to clinicians and patients by use of unequal ratio randomization (e.g. 2 patients randomized to experimental therapy for every 1 to conventional care) (Broderick et al., 2013); response-adaptive randomization (dynamically changing the randomization ratio to assign more patients to more effective or safer treatment regimens based on interim data from an ongoing trial) (Hobbs et al., 2013); and incorporation of the ‘uncertainty principle’ as an entry criterion. The ‘uncertainty principle’ approach states that a patient can be entered if, and only if, the responsible clinician is, personally, substantially uncertain which of the trial treatments would be most appropriate for that particular patient (ECST Trialists, 1998; Sackett, 2000; IST-3 Collaborative Group et al., 2012). If clinicians are inaccurate in their personal judgements of treatment benefits, trials enrolling with the ‘uncertainty principle’ as an added entry criterion will yield the same results as trials enrolling without this addition; however, if clinicians are accurate in their understanding of treatment benefits, enrolling under the ‘uncertainty principle’ will tend to bias trials toward neutral results (Vyas and Saver, 2016).
In order to assess the effects of a treatment on outcome after stroke, the treatment must be evaluated in patients, and the outcomes it yields must be compared with those in patients who have not been exposed to the treatment, but who are ideally identical in all other ways such as in prevalence and level of prognostic factors that influence outcome (i.e. a control group). A control group is needed because the outcome after stroke is neither uniformly poor nor good (i.e. it is variable), and because it is difficult to accurately predict the outcome of any individual patient (see Chapter 1).
As stroke commonly causes substantial loss of brain tissue within minutes to hours, and there are many pathogenetic pathways mediating injury in acute stroke, it is likely that individual efficacious treatments for stroke will have small to moderate benefits, rather than massively favourable effects, on patient outcome. Should a dramatically beneficial treatment exist, it could in theory exert so large a treatment effect that it could be identified reliably from observational studies of the outcome of treated patients compared with the literature or with untreated historical or concurrent controls, without the need for large randomized trials. This is because any possible modest effects of systematic or random error, either in the opposite direction to the treatment effect (i.e. reducing the true treatment effect) or in the same direction as the treatment effect (i.e. inflating the true treatment effect), are not likely to be large enough to disguise the dramatic effect of the treatment. For example, the striking effectiveness of penicillin was realized from observational studies of treated patients with hitherto uniformly fatal or disabling diseases, such as pneumococcal meningitis, who subsequently recovered dramatically after penicillin. Randomized controlled trials (RCTs) were deemed not to be required.
However, the great preponderance of (if not all) treatments for stroke are likely to have mild or moderate effects. Even relatively small benefits of a treatment for stroke are clinically worthwhile, given the frequency of morbidity and mortality and expense from the disease, particularly if the treatment is safe, inexpensive, and widely applicable (Warlow 2004; Cranston et al., 2017). In order to reliably identify such moderate, yet important, treatment effects, it is necessary to ensure that they are not underestimated or even nullified (and therefore missed) by modest systematic or random errors (false negative, or type II error) (Table 2.2) (Collins and MacMahon, 2001; Rush et al., 2018). Similarly, for treatments with no effect, it is necessary that modest systematic and random errors are minimized, and not sufficiently large to produce an erroneous conclusion that the treatment is effective (false positive, or type I error).
Systematic errors (biases) in the assessment of treatment effects |
---|
•Selection bias (systematic pretreatment differences in comparator groups) |
•Performance bias (systematic differences in the care provided apart from the intervention being evaluated) |
•Attrition bias (systematic differences in withdrawals from the treatment groups) |
•Recording/detection bias (systematic differences in outcome assessment) |
•Outcome reporting bias (selective reporting of some, but not other, outcomes depending on direction of the results) |
Random errors in the assessment of treatment effects |
---|
•Relate to the impact of play of chance on comparisons of the outcome between those exposed and not exposed to the treatment of interest |
•Are determined by the number of relevant outcome events in the study |
•The potential error can be quantified by means of a confidence interval (CI) which indicates the range of effects statistically compatible with the observed results |
•Can prevent real effects of treatment being detected or their size being estimated reliably |
Strategies to Minimize Systematic and Random Errors
Reliable and accurate identification of treatment effects requires simultaneous minimization of systematic errors (bias) and random errors (Table 2.3).
Table 2.3 Strategies to minimize systematic and random errors
Minimization of systematic error |
---|
•Proper randomization |
•Analysis by allocated treatment (including all randomized patients: intention to treat) |
•Outcome evaluation blind to allocated treatment |
•Pre-specification of primary outcome (preventing data dredging or ‘p-hacking’) |
•Chief emphasis on results in overall population (without undue data-dependent emphasis on particular subgroups) |
•Systematic review of all relevant studies (without undue data-dependent emphasis on particular studies) |
Minimization of random error |
---|
•Large numbers of participants with major outcomes (with streamlined methods to facilitate recruitment) |
•Systematic search and review of all relevant studies (yielding the largest possible number of participants with major outcome events) |
Randomization is the most efficient method of minimizing systematic bias in treatment allocation; blinded outcome evaluation is the most efficient method of minimizing observer or recording/detection bias; pre-specification and pre-registration of the primary outcome and hypothesis is the most reliable method of minimizing outcome reporting bias; and registering and analysing large numbers of participants with primary outcome events (and therefore randomizing large numbers of patients) is the main method of minimizing random error (Collins and MacMahon, 2001; Kaplan et al., 2014; Gopal et al., 2018; Strauss et al., 2019).
Of these, random error is arguably the most important to minimize. Surprisingly large numbers of patients (often thousands or even tens of thousands) must be included in randomized trials of stroke treatments to provide really reliable estimates of effect. Trials of such size are uncommon in stroke medicine.
Different study types vary in the degree to which they restrain bias and overcome noise to provide reliable measurement of treatment effects. The best evidence about the effects of stroke treatments comes from large RCTs in which there are large numbers of outcome events, and in which outcome evaluation is undertaken by observers who are blinded to the treatment allocation. Evidence-based medicine grading classifications array granular study types in order from most to least reliable. Though the fine orderings differ modestly across different consensus groups and authors (Laika 2008; Shaneyfelt 2016), the shared overall framework is to value RCTs at the highest tier, followed by large observational studies that have made some attempts at adjusting for differences in patient groups, then physiological studies, and lastly expert opinion (Table 2.4).
Randomized trials |
---|
1. Multiple, congruent, very large mega-trials |
2. Single, very large mega-trial |
3. Multiple, congruent small- to moderate-size trials |
4. Multiple, mostly congruent small- to moderate-size trials |
5. Single small- to moderate-size trial |
Observational data |
---|
6. Large and propensity-weighted or multivariate-adjusted studies |
7. Small or unadjusted studies |
Physiological data |
---|
8. Studies in humans |
9. Studies in other species |
Authority |
---|
10. Expert opinion |
Grading Systems for Recommendations in Evidence-based Guidelines
Formal grading systems have been developed to characterize the strength of evidence for diagnostic and treatment recommendations advanced in guidelines. Two systems that are commonly applied in assessing stroke prevention and treatment strategies are (1) the Grading of Recommendations Assessment, Development and Evaluation (GRADE) working group system (Table 2.5) (Guyatt et al., 2011); and (2) the American College of Cardiology/American Heart Association (American Stroke Association) (ACC/AHA) clinical practice guideline recommendation classification system (Table 2.6) (Halperin et al., 2016).
Study design | Quality of evidence | Lower rating if … | Higher rating if … |
---|---|---|---|
Randomized trial | High |
|
|
Moderate | |||
Observational study | Low | ||
Very Low |
Table 2.6 ACC/AHA (ASA) system for rating strength of, and quality of evidence for, evidence-based guideline recommendations
Class (strength) of recommendations Reflects the magnitude of benefit over risk | |
---|---|
Class I (Strong) | Benefit>>>Risk |
Class IIa (Moderate) | Benefit>>Risk |
Class IIb (Weak) | Benefit>Risk |
Class III: No Benefit (Moderate) | Benefit=Risk |
Class III: Harm (Strong) | Risk>Benefit |
Level (quality) of evidence Reflects the certainty of the evidence supporting the recommendation |
---|
Level A
|
Level B-R (randomized)
|
Level B-NR (nonrandomized)
|
Level C-LD (limited data)
|
Level C-EO (expert opinion)
|
Key Elements to the Design of a Clinical Trial of a Treatment for Stroke
All clinical trials should be designed and reported using the CONSORT guidelines (Schulz et al., 2010). However, not all trials are reported in this way and not all journals insist on it (Blanco et al., 2018). Thus, some trials may have been carried out adequately but reported inadequately, and others may have been designed and carried out inadequately. When analysing a clinical trial, several important aspects of design, conduct, reporting, and interpretation should be considered. Several key aspects are covered in this section and, for further details of what to look for in a report of an RCT, we would recommend Lees et al. (2003), Lewis and Warlow (2004), Rothwell et al. (2005), Schulz et al. (2010), Saver (2011), Bath et al. (2012), Dahabreh et al. (2016), Hill (2018), and Higgins et al. (2018).
Hypothesis and Aim
Are the study primary hypothesis and aim clearly stated, in a testable (falsifiable) manner? In addition, was the primary analysis to test the study hypothesis pre-specified prior to data unblinding?
Were study secondary hypotheses and subgroup analyses clearly stated? In addition, were the analyses to test secondary hypotheses and subgroups pre-specified prior to data unblinding?
Design
What is the study design?
Is it a randomized trial?
Is the method of randomization described and was it an appropriate method? (Broglio, 2018)
Was the decision to enter each patient made irreversibly in ignorance of which trial treatment that patient would be allocated to receive? If not (e.g. if allocation was based on date of birth, date of admission, alternation [e.g. first patient receives treatment A, second receives treatment B, and alternating assignment continues thereafter]), foreknowledge of the next treatment allocation could affect the decision to enter the patient, and those allocated one treatment might then differ systematically from those allocated another.
Were adequate measures taken to conceal allocations, such as use of central internet or phone randomization assignment systems? Or were concealment methods vulnerable to potential breaching by site staff, such as use of sealed envelopes that could be held up to the light and made semi-transparent?
Patients
Were explicit and clearly operational inclusion and exclusion criteria employed?
Were most eligible patients enrolled? Or were many treated outside of the trial, rendering the trial population potentially not representative of the targeted patient population?
Follow-up
Were all patients followed up prospectively at pre-specified, regular intervals?
Was patient follow-up complete?
If not, this can lead to attrition bias (systematic differences in withdrawals from trials), because patients who are withdrawn from, or stop participating in, a trial tend to differ from those who remain in the study (e.g. they may have a higher rate of complications or adverse effects from the disease or treatment respectively). This type of bias can be minimized by performing an ‘intention-to-treat analysis’ where the analysis of results at the end of the study includes every patient who was assigned to the intervention or control group, regardless of whether they received the assigned treatment or subsequently dropped out of the trial (see below).
Was the trial stopped early? Truncated RCTs may be associated with greater effect sizes than RCTs not stopped early (Bassler et al., 2010).
Outcome measures
Is the primary measure of outcome:
relevant to the patient (e.g. death, functional dependency, serious vascular event)?
relevant to the intervention (i.e. potentially modifiable by the treatment, given its expected mechanism of action)?
valid (does it actually measure what it intends to measure)?
reliable (reproducible)?
simple?
communicable?
Avoid surrogate outcome measures in pivotal phase III studies:
Surrogate outcomes may reflect only one part of the disease process and beneficial effects on them may not be associated with worthwhile improvements in survival and functional outcome. Deciding whether a treatment is safe and efficacious just on the basis of its effects on a physiological measurement, a blood test, or an imaging biomarker may be misleading.
Basing treatment decisions on effects on surrogate outcomes may be hazardous (Fleming and Powers, 2012). Cardiac premature beats are associated with a poor prognosis and antidysrhythmic drugs can markedly reduce their frequency. However, various antidysrhythmic drugs, though they reduce the surrogate outcome (ventricular premature beats), actually increase mortality.
Outcome Evaluation
Statistical Analysis
Is the primary analysis by intention-to-treat (i.e. is the final analysis based on the groups to which all randomized patients were originally allocated)?
Even in a properly randomized trial, bias can be inadvertently introduced by the post-randomization exclusion of certain patients (e.g. those who are non-compliant with treatment), particularly if the outcome of those excluded from one treatment group differs from that of those excluded from another. ‘On-treatment’ comparisons, among only those who were compliant, are therefore potentially biased (DeMets and Cook, 2019). However, because there is always some non-compliance with allocated treatments in clinical trials, an intention-to-treat analysis tends to underestimate the effects produced by full compliance with study treatments. In order to estimate the treatment effect with full compliance, it is more appropriate to avoid using the potentially biased ‘on-treatment’ comparisons, and to apply the approximately level of compliance seen in the trial (e.g. 80%) to the estimate of the treatment effect provided by the intention-to-treat comparison, to yield a less-biased estimate of therapeutic effect with full compliance (e.g. a 10% absolute risk reduction with 80% compliance would suggest a 12% absolute risk reduction with 100% compliance).
Is the point estimate for the treatment effect, and its confidence interval (CI), stated, not just p-values?
It is important that the results are expressed to indicate the clinical magnitude of effect (treatment effect point estimate) and the uncertainty around that magnitude (CI), not just statistical significance or non-significance (p-value) (Sullivan and Feinn, 2012). Trials optimally should give a precise estimate of treatment effect and therefore have narrow CIs (e.g. absolute reduction of 25%, 95% CI: 22–28%).
Is the result stated as an absolute effect rather than only as a relative effect?
Large relative treatment effects can look impressive (e.g. ‘50% reduction’), but if event rates are low, the absolute benefit may be small (e.g. reduction from 2% to 1% of patients having an event over 10 years).
Is the result clinically significant as well as statistically significant?
Outcome differences that do not exceed the minimal clinically important difference (MCID), as determined by patients and clinicians, are of no important consequence. As has been said, ‘A difference, to be a difference, must make a difference.’
Do pre-specified secondary outcomes show the same general direction and degree of effect as the primary outcome?
Consistent evidence of a treatment effect across all pre-specified endpoints that are expected to have some co-variation provides supportive evidence that the benefit or non-benefit on the primary outcome reflects a genuine biological effect and not play of chance.
Are there safety (on-treatment) population and per-protocol population analyses, and are they appropriate?
In addition to the primary analysis in the intention-to-treat population, it can be helpful to analyse select outcomes in predefined safety and per-protocol populations. The safety/on-treatment population will include all patients as actually treated, rather than as randomized (e.g. a patient who was randomized to treatment A but actually received treatment B will be included in the treatment A group in the intent-to-treat analysis, but in the treatment B group in the safety analysis). Analysis of the safety/on-treatment population may be of value in describing the frequency of specific adverse effects among only those who actually received the treatment. The ‘per-protocol’ population will include all patients in both treatment groups deemed to have been handled throughout the study in a protocol-adherent manner, including adjudicated as meeting all study entry criteria even when additional information about the patient emerges after trial enrolment and complying with the allocated treatment. Evidence that treatment benefits or harms are magnified in the per-protocol population, compared with the intention-to-treat population, provides supportive evidence that the effects are due to genuine biological activity rather than play of chance.
Is the intervention cost-effective compared with (an)other intervention(s)?
Given that finite societal resources are available for healthcare, it is important to quantify the relative health benefits, harms, and costs associated with alternative interventions by means of a cost-effectiveness analysis. This is an analytic approach in which the incremental financial costs and incremental changes in health outcome states of an intervention (intervention A) and at least one alternative (intervention B) are calculated. The incremental costs are the additional resources (e.g. medical care costs, costs from productivity changes) incurred from the use of intervention A over intervention B. The health outcome changes are typically the number of cases of a disease prevented or the number of quality-adjusted life-years (QALYs) gained through the use of intervention A over intervention B. The result is expressed as the difference in cost between the two interventions divided by the difference in their effect – the incremental cost effectiveness ratio (ICER) (Sanders et al., 2019). The World Health Organization has suggested that a reasonable threshold for considering an intervention cost-effective is 1–3 times the annual per capita gross domestic product (GDP) of a country per additional QALY, which for the developed nations is approximately $50,000 to $175,000 per QALY gained (WHO Commission on Macroeconomics and Health, 2001).
If there are there any subgroup analyses:
Were they pre-specified and adjusted for multiple data looks?
As individual patients differ from each other and treatments can have disparate effects in individuals with different clinical features, there is an understandable temptation to examine treatment effects in subgroups of interest, particularly if the overall trial is negative. However, the more subgroups that are examined the more likely that an effect will be identified due to chance (e.g. analysing 20 subgroups will lead to one being statistically significant at the p = 0.05 level, 1 out of 20, just due to play of chance) (Counsell et al., 1994; Munroe, 2011). Post hoc subgroup analyses cannot, therefore, be regarded as anything more than hypothesis-generating. Even pre-specified subgroup analyses, if many analytic groups were planned, must be considered hypothesis-generating unless the p-value threshold for considering a finding statistically significant has been lowered to account for the multiplicity of analyses. Any apparent treatment effect must be confirmed in a further trial in which there is an a priori hypothesis that a particular subgroup of patients will benefit while other subgroups will not.
Are claims advanced regarding them based on evidence of differences in response between the groups?
Individual population segments in subgroup analyses have fewer patients than in the overall trial. Consequently, analysis of an individual subgroup segment alone (e.g. only older patients) is almost always underpowered to determine the presence or absence of treatment effects, and is vulnerable to showing chance associations or non-associations. In contrast, analyses of differences across subgroup segments (e.g. older versus younger patients) use the full size and power of the whole sample of randomized patients. Evidence of heterogeneity of response across subgroups (e.g. an interaction between age and treatment response) provides more reliable evidence of a genuine qualitative difference in treatment effect (Table 2.7) (Sun et al., 2010; Wallach et al., 2017).
Table 2.7 Criteria to assess the credibility of subgroup analyses
Design |
---|
1.Is the subgroup variable a characteristic measured at baseline or after randomization? |
2.Is the effect suggested by comparisons within rather than between studies? |
3.Was the hypothesis specified a priori? |
4.Was the direction of the subgroup effect specified a priori? |
5.Was the subgroup effect one of a small number of hypothesized effects tested? |
Analysis |
---|
6.Does the interaction test suggest a low likelihood that chance explains the apparent subgroup effect? |
7.Is the significant subgroup effect independent? |
Context |
---|
8.Is the size of the subgroup effect large? |
9.Is the interaction consistent across studies? |
10.Is the interaction consistent across closely related outcomes within the study? |
11.Is there indirect evidence that supports the hypothesized interaction (biological rationale)? |
Conclusions
Is the study conclusion supported by the trial’s findings?
Claims that a trial has shown a treatment benefit or harm should only be advanced if findings for the pre-specified primary outcome show an effect that is both statistically significant (exceeds the pre-specified p-value threshold for the trial) and clinically significant (exceeds the MCID) (Pocock and Stone, 2016b). Conversely, if a trial has not shown superiority of one treatment over another, that does not mean it has shown the two treatments are equivalent (Pocock and Stone, 2016a; Mauri and D’Agostino, 2017). Failure to demonstrate that treatment yields a large difference in outcomes does not rule out the possibility that the treatment yields a more modest, but clinically meaningful, difference in outcomes (Pocock and Stone, 2016a, 2016b). Many trials are underpowered to rule out small to moderate, but clinically meaningful, effects on outcome. Only if the trial results have demonstrated that any differences between the treatments must be less than the MCID (as is the goal of equivalence and non-inferiority trials) can a claim be advanced that there are no differences in outcome with the study intervention.
Trial Sponsorship and Competing Interests
The development of new medical interventions requires funding, and elements of a market economy are indispensable. However, capitalism should be subject to restraining forces to ensure an appropriate balance with any other endeavour in society, and especially so when individuals’ lives and health are directly affected. Sponsors of trials of interventions have a right to use their knowledge regarding an intervention’s effect and general principles of study design to inform trial protocols, but they also have a responsibility to study participants and future patients and prescribers of an intervention to include, in study design, conduct, analysis, and reporting, clinicians with expertise in the disease being treated and in disease-specific aspects of trial design (Donnan et al., 2003; van Gijn, 2005; Harman et al., 2015; Rasmussen et al., 2018).
Due to the potential for the best interests of patients and society to be compromised by the financial interests of the sponsors and prescribing/procedure-performing clinicians, it is essential that the highest form of honesty and integrity prevails (Shaw, 1911; Lo and Field, 2009).
To foster ethical conduct and reporting of clinical trials, several regulations and guidelines have been developed. ‘Good Clinical Practice (GCP)’ guidelines provide an ethical and scientific quality standard for investigators, sponsors, monitors, and institutional review boards throughout each stage of drug trials (International Council for Harmonisation, 2016). The GCP recommendations focus on diverse study aspects, including the relations between the site clinical investigator and both the patient and the sponsor, how often every value in the trial records of individual patients should be checked against source medical records, and which disciplines and stakeholders should be represented on institutional review boards/research ethics committees. In a complementary effort, the DAMOCLES consensus group has delineated the types and roles of data safety and monitoring boards tasked to perform constant oversight of the well-being and interests of trial participants during study conduct (DAMOCLES Study Group, 2005). The International Committee of Medical Journal Editors (ICMJE) have established requirements for transparent reporting of who was responsible for data storage, management, and analysis among study sponsors and academic steering committees (ICMJE, 2018). Best practices in transparent declaration and management of financial conflicts of interest among clinical investigators have been promulgated (AAMC-AAU Advisory Committee on Financial Conflicts of Interest in Human Subjects Research, 2008; Lo and Field, 2009; Stead, 2017). To prevent non-publication of unfavourable trial results (publication bias), the ICMJE, consensus groups, and governmental legislation and regulations have indicated that trials should be publicly registered before initiation, report key results in a publicly accessible manner, and work to make de-identified individual patient-level data available for external, independent analysis (Laine et al., 2007; Ali et al., 2012; Zarin et al., 2016; Taichman et al., 2018). Preliminary guidance has also been developed regarding the composition, roles, and responsibilities of academic steering committees of clinical trials (Donnan et al., 2003; van Gijn, 2005; Harman et al., 2015; Rasmussen et al., 2018).
There is evidence that research funded by pharmaceutical companies is more likely to have outcomes favouring the sponsor than research funded from other sources (Bekelman et al., 2003; Falk Delgado and Falk Delgado, 2017; Lundh et al., 2017), including for neurovascular and cardiovascular trials (Liebeskind et al., 2006; Ridker and Torres, 2006). As industry-funded trials, compared with academic sponsored trials, are on average designed with higher-quality features to reduce risk of bias (Lundh et al., 2017), the higher rate of positive findings seems likely to be due to non-reporting of nonpositive trials (publication bias), more frequent use of surrogate endpoints more likely to show treatment effect, and more favourable interpretation of study mathematical results (‘spin’). Recent initiatives to mandate trial registration and reporting and to require pre-specification of primary analyses may mitigate this discrepancy, and represent appropriate regulatory supervision to ensure transparent and complete reporting of trial results regardless of sponsor type.
It is crucial that trial sponsorship and all potential competing interests are presented in any report of a study.
Limitations of RCTs
Sample Size (Random Error and Imprecise Estimates of Treatment Effects)
Although RCTs provide the least biased and hence most reliable evaluation of whether a treatment is effective and safe, they are commonly limited by suboptimal sample size. As a result, there is potential for some random error and therefore imprecision in the estimated treatment effect.
Generalizability/External Validity
Another weakness of RCTs is limited generalizability. The results of trials conducted in a single centre, region, or country and in a single racial or ethnic group or type of patients cannot necessarily be generalized (applied) to other centres, regions, or countries and other racial or ethnic groups or types of patients. Often, patients enrolled in clinical trials are on average younger and healthier than patients encountered in clinical practice (Flather et al., 2006; Sheth et al., 2016). Even well-executed trials with sound internal validity may not necessarily inform us about the effect of a treatment among patients who were not entered into the trial (i.e. its external validity) (Rothwell, 2005; Dekkers et al., 2010).
One of the solutions to the geographical and race–ethnic limitations of RCTs conducted in individual countries is to conduct multicentre trials in multiple countries, but the disadvantages include practical difficulties, time, and cost (Senn and Lewis, 2019). Another solution, particularly if a single, large multi-national RCT is impractical, is to perform parallel trials in different regions contemporaneously, using shared methodology and data definitions to facilitate pooled analysis upon completion (Mead et al., 2015). Failing that, a further approach is to perform a systematic review and meta-analysis of independently designed and conducted trials from different localities.
Systematic Reviews and Meta-analyses of RCTs
A systematic review and meta-analysis seeks to reduce systematic error (bias) and random error by applying scientific methods to the review of all of the published and unpublished research evidence; in this case RCTs. The PRISMA statement provides consensus recommendations of best practices when reporting systematic reviews and meta-analyses (Liberati et al., 2009).
Systematic Reviews
The scientific methods or strategies of a systematic review include the following:
Defining the research question to ensure the review will be relevant and reliable and guide the development of the review protocol. Most reviews define a broad question (e.g. Does thrombolysis in acute ischaemic stroke improve outcome?) which includes several pre-specified subquestions (e.g. Does thrombolysis with alteplase within 3 hours of stroke onset reduce death and dependency at 3–6 months after stroke?).
Developing a review protocol based on the research question. The protocol contains specific explicit and reproducible inclusion and exclusion criteria for selecting trials for the review in order to minimize bias in trial selection. The protocol also contains explicit methods of data extraction and synthesis to minimize bias during data collection and the analysis of results.
Undertaking a systematic and comprehensive search for all potentially relevant trials.
Applying the pre-specified eligibility criteria to select relevant trials.
Performing a critical appraisal of quality (research designs and characteristics) of the trials to ensure that most emphasis is given to the most methodologically sound trials.
Extracting and analysing data using predefined, explicit methods. The statistical synthesis of analysis of the results is called a meta-analysis (see Meta-analyses section later in this chapter).
Interpreting the results and drawing conclusions based on the totality of the available evidence (not a biased subset).
Therefore, a systematic review provides a method of reviewing the available evidence using explicit scientific strategies to reduce any bias (e.g. in trial selection and data extraction) in the estimate of the direction of the treatment effect from using only selected trials, and to increase the precision of the estimate of the treatment effect by examining a larger amount of data and thereby reducing random error (Higgins and Green, 2011; Murad et al., 2014; Pollock and Berge, 2018).
Sources of Bias in Systematic Reviews
There are three main sources of bias in systematic reviews: publication bias, study quality bias, and outcome recording bias.
Publication Bias
Systematic reviews aim to identify and include all trials that are relevant to the research question. However, some studies are difficult to find, and these may tend to differ from trials which are easy to find. For example, studies which have reported a ‘positive’ or interesting result are more likely to be published, and therefore easier to locate, than studies which have produced ‘negative’ (harmful or neutral) results (Liebeskind et al., 2006; Hopewell et al., 2009). The conduct of a systematic review therefore needs to engage multiple overlapping sources of study ascertainment. The search should ideally cover multiple electronic databases of published trials (e.g. Medline, Embase), mixed published and unpublished trials (e.g. the Cochrane Central Register of Controlled Trials [CENTRAL]), and trial registries (e.g. clinicaltrials.gov in the USA, the Australian New Zealand Clinical Trials Registry [ANZCTR] in Australasia, and the Chinese Clinical Trial Registry [ChiCTR] in China), as each individual database has some restrictions in scope, e.g. to a journal in certain languages. In addition, hand searching of additional sources, including additional journals, conference abstracts, theses, and unpublished trials, should be undertaken, as well as review of studies cited in initially retrieved articles (Higgins and Green, 2011; Chan, 2012; NICE, 2014).
Study Quality Bias
There is sound empirical evidence that more methodologically robust trials tend to indicate that new treatments are less effective than do less reliable trials (Savovic et al., 2018). It is therefore important that the conduct of a systematic review includes a measure of the methodological quality of the trials included, and if possible a sensitivity analysis of the results according to the methodological quality of the trials (Higgins and Green, 2011; Higgins et al., 2018).
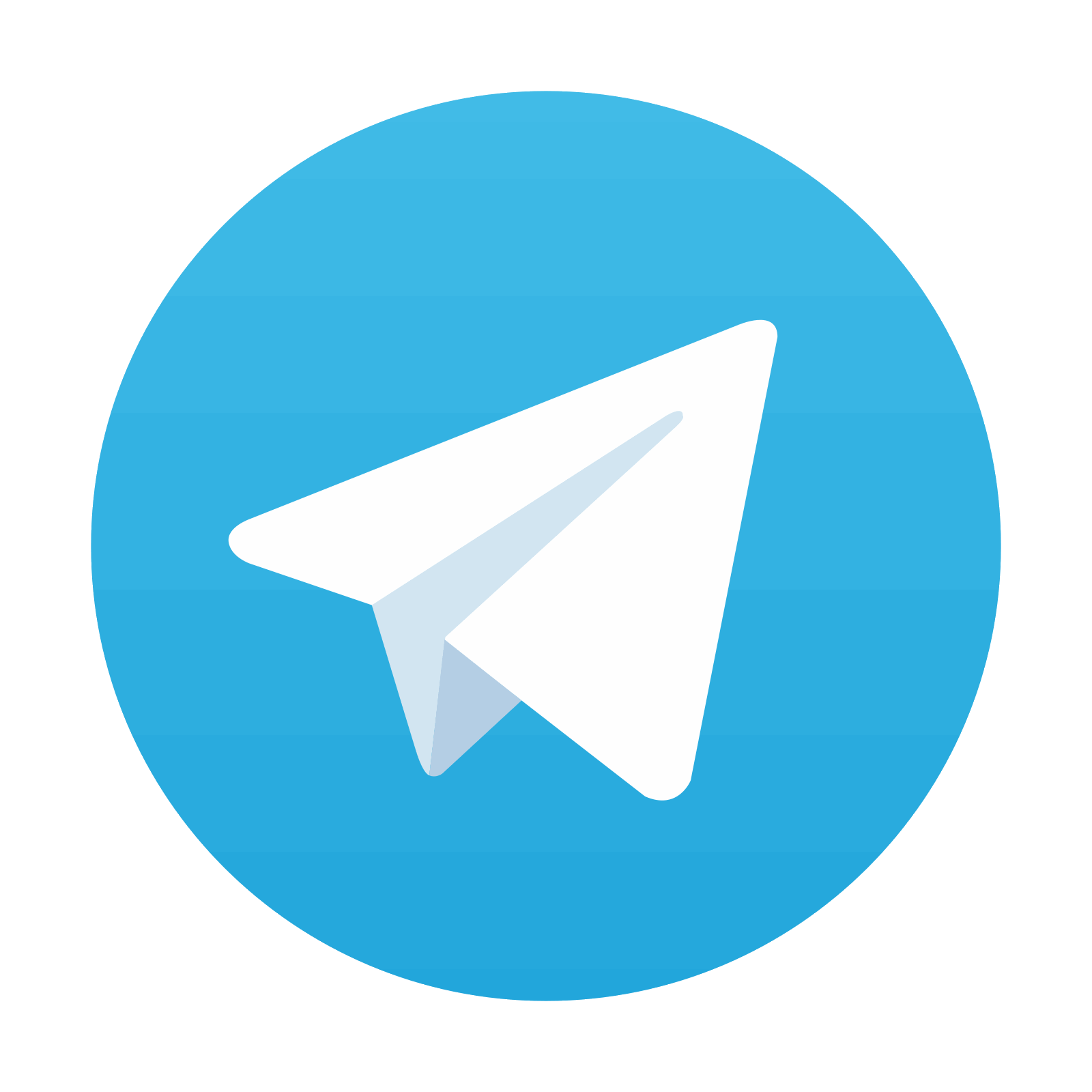
Stay updated, free articles. Join our Telegram channel

Full access? Get Clinical Tree
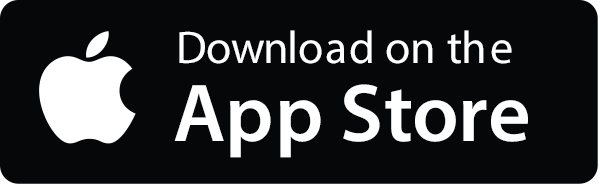

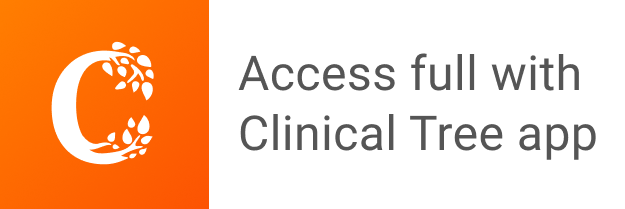