Fig. 1
Genetic approaches used in sleep homeostasis research. For each of these approaches, different techniques can be employed in different animal species to bring information about the involvement of genes in sleep and its homeostasis. The three approaches are complementary and should be combined to decipher the molecular pathways of sleep homeostasis
In humans, traits of interest are generally examined by linkage analyses. Linkage studies investigate shared chromosomal fragments among members of a family who manifest the trait of interest or the disease. By analyzing the co-segregation of the trait and chromosomal markers, it may be possible to statistically identify chromosomal loci “linked” to the trait. However, linkage studies are limited by the availability of family-based samples, and rely on the observation of heritability of the trait of interest. In comparison, association studies of genetically complex traits require larger samples and involve comparisons between cases and controls with respect to selected polymorphisms (Altshuler et al. 2008; Chiang et al. 2009). In genome-wide association studies (GWAS) the concept of association study is extended to the whole genome, with the idea to observe the segregation of many polymorphisms with the disease or the trait. Thus, the relative impact of different genomic regions can be assessed simultaneously in the same sample. Despite initial excitement the loci identified in GWAS studies have, in general, weak additive power, explaining only a small portion of the narrow-sense heritability reported for a given phenotype. This suggests that rare rather than the common variants interrogated in a GWAS, underlie the phenotype of interest. Moreover, most reported loci are noncoding and thus not immediately informative and GWAS results not always replicate across studies and populations leading to false positives (Ward and Kellis 2012).
The GWAS can also be applied to outbred mouse populations (Yalcin et al. 2010). Increased recombination in outbred populations is expected to provide greater mapping resolution than traditional inbred line crosses, improving prospects for identifying the causal genes for a trait. However, outbred populations are not a GRP and thus each individual is genetically unique and has to be genotyped individually. This also limits the number of phenotypes that can be obtained for a given genotype which is another advantage of using GRPs.
3.2 Reverse Genetics
In contrast to forward genetics, in which the strategy is to go from the observed phenotype to the underlying genotype, reverse genetics starts with a disrupted or altered gene of interest and examine its effect on a phenotype or phenotypes of interest. In humans, reverse genetic studies most often concern natural occurring functional polymorphisms while the use of animal models allows for targeting specific genes. Transgenic animals can be used to study the consequences of overexpression, ectopic expression, time- and tissue-specific expression, and gain or loss of function of a specific gene. To date, a large number of studies in sleep genetics have utilized reverse genetic approaches by knocking-out a gene of interest (see Sect. 5.1). The delineation between reverse and forward genetic approaches is not a formal one and depends on the scale at which they are used. Transgenic strategies can also been used in forward genetic studies, in which hundreds of mutated or knockout (KO) lines of mice (Knight and Abbott 2002), or flies (Cirelli 2003) are screened. The International Knockout Mouse Consortium is currently creating a collection of mouse in which all protein-coding genes are mutated and will therefore provide a population of animals for genome-wide screens (Ringwald et al. 2011).
3.3 Molecular Genetics
Molecular genetics is an approach that evaluates changes in gene expression related to the trait of interest; it is an unbiased method that makes use of several techniques such as DNA microarrays, proteomics, and RNA sequencing. Molecular approaches in sleep studies are based on the assumption that the expression of genes change as a function of time spent awake or asleep (Cirelli et al. 2004; Mackiewicz et al. 2009; Thompson et al. 2010). Rhyner et al. were the first to use a molecular approach to identify genes that change their expression after SD in the rat, and identified the protein neurogranin to be decreased in the rat forebrain after 24 h of SD (Rhyner et al. 1990). These methods have been helpful in identifying the brain expression of genes involved in sleep or modulated by sleep (see Sect. 8). However, these approaches cannot reveal a causal relationship between a gene or a set of genes and a particular behavior or phenotype. For example, a gene that does not show transcriptional modification may nonetheless play an important role in the process under investigation. On the other hand, a transcript that does increase with increased sleep need might merely be driven by the sleep–wake distribution while not playing a role in the homeostatic sleep process itself.
It is clear that the above approaches are complementary; e.g., the function of candidate genes identified with QTL or molecular genetics approaches have to be confirmed in KO animals (an example is provided in Sect. 8.1). Future efforts should therefore combine genetics approaches in animal models and GWAS in humans to facilitate uncovering the molecular pathways that underlie sleep homeostasis. However, other approaches and techniques will remain important in making progress in elucidating the complex physiology of sleep; genetic dissection of sleep can be used in conjunction with state-of-the-art electrophysiological, neuroanatomical and pharmacological techniques already used with great success in sleep research.
4 In Search for Sleep Homeostatic Genes: Defining the Phenotype
A sleep homeostatic gene could be defined as a gene that modifies the sleep–wake dependent dynamics of EEG delta power or a gene that affects the (compensatory) increase in NREM or REM sleep duration after SD . Establishing whether a gene variant affects a sleep homeostasis process is, however, not always straightforward and several considerations have to be taken into account in the analysis. Here we illustrate some of the general problems that can occur with interpreting the results of SD studies that should be considered before claiming a homeostatic phenotype is observed.
Although, sleep homeostatic processes could, in principle, be assessed and quantified under baseline conditions, usually sleep homeostatic responses are studied after experimentally challenging sleep need through; e.g., keeping subjects awake for a certain duration at a time-of-day sleep is present under undisturbed baseline conditions. In most studies, sleep variables measured during recovery sleep after enforced waking are then contrasted to the individual levels reached in baseline. Subsequently, these relative changes are compared between genotypes to assess the effect of a disrupted or mutated gene of interest on sleep homeostasis (Fig. 2a). A slightly more elaborate variant of such analysis are the so-called “gain-loss” time course analyses in which the sleep loss incurred over the course of a SD is analyzed by accumulating the differences of sleep observed during the SD minus the sleep duration observed during the same time-of-day during baseline (Fig. 2b′). This accumulated sleep deficit then serves as the starting point of the sleep gained during the recovery period following a SD and is assessed in the same way (i.e., as relative differences from matching times during baseline). Genotype differences in ‘gain-loss’ dynamics are then taken as evidence of an altered homeostatic regulation of sleep. An important short-coming of such analyses is that they implicitly assume that the sleep obtained during baseline is the amount a subject “needs” or, in other words, the duration of sleep that is homeostatically defended. For instance, the deficit in sleep duration in the gain-loss analyses introduced above exclusively reflects the sleep duration obtained during the baseline period the SD took place (given that no sleep is obtained during the SD protocol). Also the recovery dynamics are obtained by contrasting recovery values to corresponding baseline values. In the hypothetical example shown in Figs. 2b and 2b′, the recovery dynamics of sleep is exactly the same; i.e., each hour the same duration of extra sleep is obtained making the recovery curves run in parallel. Nevertheless, a researcher can claim the discovery of a homeostatic phenotype because of statistical genotype differences that, in this case, are due only to a difference of sleep expressed during baseline at the time SD was performed. In another example, recovery was made to be exactly proportional to the sleep duration the animal was deprived of and this amount of extra sleep was linearly distributed of the 18 h depicted (example in Fig. 2b′). As a result, in this exercise, recovery is “complete” by hour 24 (i.e., after 18 h of recovery) reaching the zero deficit level in both genotypes. Nevertheless, a researcher can again claim that homeostatic regulation is different between genotypes because the SD resulted in different relative deficits and the slopes of the gain process differed, while it could equally be claimed that recovery of sleep time lost is perfect and not different in both because in one case more sleep was deprived of and thus a higher pressure for sleep was accrued leading to more extra sleep. Many other scenarios could be construed pointing out these or related problems such as the so-called “ceiling effect.” This effect is sometimes alluded to account for a smaller increase attained during recovery at times-of-day when during baseline the subject already sleeps a lot. It is clear, and all will agree, that the level of a sleep variable expressed during baseline cannot be attributed to homeostatic need alone. For example, in a study examining sleep need in short and long sleepers that differed in habitual sleep duration by more than 3 h, it was concluded that the dynamics of the sleep homeostat did not differ and that short sleepers somehow could resist higher levels of sleep pressure (Aeschbach et al. 1997, 2001).
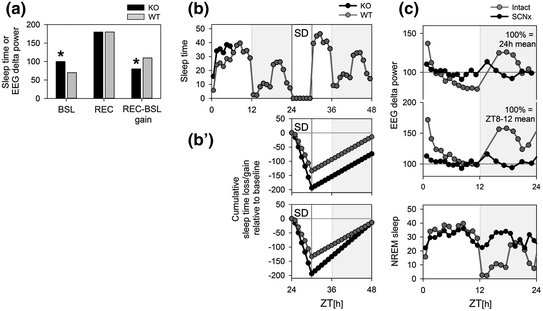
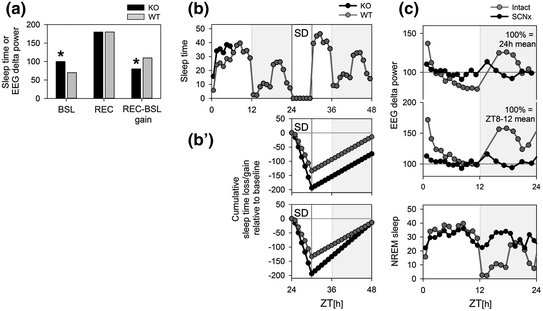
Fig. 2
Conceptual issues when assessing a sleep homeostatic phenotype. (Panel a) Sleep regulation in mice carrying a targeted deletion of a gene (i.e., knockout or KO) is compared to that in wild-type (WT) controls by submitting mice of both lines to a sleep deprivation (SD). Time spent asleep during the recovery period (REC) or the level of EEG delta power reached after the onset of recovery sleep was higher than in baseline (BSL) in both genotypes but levels reached did not differ between them. Nevertheless, by contrasting the values obtained during recovery to those obtained during baseline (REC-BSL gain), a significant (*) difference in gain was observed. The gain difference in this hypothetical example must, however, be ascribed to the differences in baseline. (Panels b) In another example, KO mice differed only from WT mice in the first 6 h of the baseline (ZT0-6; top panel) during which KO mice slept 1 h more. During the remainder of the experiment the two genotypes had identical amounts of sleep. The effect of SD was analyzed with a loss/gain analysis by accumulating the sleep time lost during the SD (−140 min in WT) and the extra sleep obtained during recovery from a 6 h SD (+200 min in both genotypes). Because of the baseline difference in sleep amount at the time the SD was performed the following day, KO mice start with a 60 min higher sleep deficit at the start of recovery sleep. Although the extra sleep obtained during recovery sleep did not differ (parallel gain curves) KO maintain a deficit. In (Panel b′) a scenario is depicted where both genotypes fully compensate for their differing sleep time lost during SD; i.e., reaching 0 levels within the 18 h of recovery. Does sleep homeostasis differ (steeper gain curve) or not (same end point)? Baseline and recovery data for b′ not included in upper panel. (Panels c) The sleep–wake distribution (lower panel) drives changes in EEG delta power such that during periods when NREM sleep is prevalent (light period ZT0-12) EEG delta power decreases in an exponential fashion while during period of wakefulness (first half of the dark period; ZT12-18) EEG delta power in subsequent NREM sleep is elevated. In SCN-lesioned (SCNx) animals that sleep more and in which the distribution of NREM sleep over the day is strongly reduced, EEG delta power can be expected to be low and its time course flat over a 24 h baseline recording. Taking the 24 h mean value of EEG delta power as a reference (100 % upper panel), as is often done, results in overall higher relative values of EEG delta power in SCNx mice such as in baseline (e.g., ZT6-12) and also after SD (not illustrated) compared to intact mice that can lead to erroneous conclusions concerning homeostatic sleep need. We propose to take the EEG delta power values reached during the last 4 h of the main rest phase (ZT8-12) as a reference (100 % middle panel)
The problem becomes even more complex when the variable cannot be directly analyzed in absolute terms (i.e., in the units they are measured) because, due to a range of confounds (e.g., electrode placement, impedance) variability among individuals is large thereby decreasing statistical power. Quantitative EEG variables are therefore often expressed relative to an individually calculated reference value to reduce variability among subjects. Differences in absolute levels should also be corrected for variables for which only the sleep–wake dependent changes are considered informative. Our genetic analyses of the sleep–wake dependent relative changes in EEG delta power and of the contribution of EEG delta power to NREM sleep EEG revealed that these two aspects are under the control of different genetic factors (Franken et al. 2001; Maret et al. 2005). Similarly, EEG delta power contributes more prominently to the NREM sleep EEG in female compared to male mice; nevertheless, the sleep–wake dependent dynamics of the changes in EEG delta power differ with sex such that the build-up in females is slower than in males, and therefore higher absolute EEG delta power not necessarily reflect higher homeostatic sleep need (Franken et al. 2006). Studies in humans arrive at similar conclusions. EEG analyses in subjects carrying specific polymorphisms has demonstrated that large genotype effects on the prevalence and amplitude of EEG delta waves (absolute values) do not necessarily translate in differences in the sleep–wake dependent relative changes in EEG delta power (i.e., homeostasis; reviewed in Franken 2012). As a last example illustrating this duality between these two aspects of EEG delta power , the effect of benzodiazepines can be mentioned. These sleep-promoting drugs are known to reduce EEG delta power while leaving the sleep–wake dependent dynamics unaffected (Borbely and Achermann 1991). Therefore, one should be cautious with making claims on sleep homeostasis and sleep depth based on absolute EEG values. Assessing other, non-EEG indexes of NREM sleep depth or quality such as the fragmentation of NREM sleep (Franken et al. 1991a, 1999) or arousal thresholds (Neckelman and Ursin 1993; Wimmer et al. 2012) could be used to strengthen such claims.
When investigating the homeostatic regulation of sleep, the relative changes in EEG delta power related to the sleep–wake distribution are analyzed. The individual reference value for EEG delta power should be chosen such that effects of eventual differences in the sleep–wake distribution and sleep duration do not affect the result. As the sleep–wake distribution is the main determinant of the changes in EEG delta power , genotype differences in sleep duration as well as sleep distribution can greatly impact average levels of EEG delta power while not (necessarily) impacting the dynamics of the underlying homeostatic process. This can be illustrated in SCN-lesioned animals (Trachsel et al. 1992). In our hypothetical example (Fig. 2c), removing the SCN results in an increase in sleep and in a “flat” distribution of sleep over the 24 h day mainly due the lack of the consolidated period of wakefulness after dark onset. The bout of spontaneous wakefulness results in high levels of EEG delta power in subsequent NREM sleep in the intact animals, levels that are never reached in SCN-lesioned animals. Therefore, when taking the 24 h mean value of EEG delta power as a reference, as is often done, this reference value will be higher in the intact animals compared to the same reference in the arrhythmic animals. As a result, the relative values calculated using this reference, will be lower in the intact animals and when compared to the lesioned animals could lead to erroneous statements concerning sleep homeostasis because the difference in the level in EEG delta power reached after SD are an artifact of selecting the reference. As an alternative, we have proposed to use the mean EEG delta power reached in the last portion (4 h) of the rest phase (or light phase in nocturnal species) as a reference (Franken et al. 1999). Levels reached at this time are less dependent on eventual differences in the sleep–wake distribution because EEG delta power decreases exponentially and EEG delta power differences at sleep onset gradually disappear over the course of the rest phase. In our hypothetical case of the SCN-lesioned animal, EEG delta power does not appreciatively deviate from this low level (Fig. 2c).
Another issue concerning the interpretation of EEG delta power seen in the literature is to contrast EEG delta power measured after SD to its value at corresponding clock time during baseline. Already minor genotype differences in the sleep–wake distribution during baseline can lead to significant differences in EEG delta power . Therefore, by expressing values reached after SD as a percentage of values reached during particular times during baseline can lead to wrong conclusions. To circumvent the problem of having to contrast values obtained after a SD to a baseline reference, “dose–response” experiments could be considered. In such studies, SD s of different durations could be used to quantify directly the dynamics of the relationship between the time animals were kept awake and the response variable (sleep duration, EEG delta power etc.; e.g., Tobler and Borbely 1986; Franken et al. 2001; Seugnet et al. 2006). To quantify the relationship between the sleep–wake distribution and EEG delta power , computer simulations can be used as an alternative approach (Franken et al. 2001).
In conclusion, the definition of an altered sleep homeostasis is not trivial. Besides some of the conceptual issues outlined above, differences in methods to perform SD such as the sleep time allowed during the SD , recording and analyzing the EEG further add to the problem of unambiguously establishing whether a genotype difference in the response to sleep loss qualifies as a homeostatic sleep phenotype.
Many different cells, molecules, and signaling pathways have been investigated through reverse genetic studies for their possible role in the control of sleep homeostasis (Table 1). In the Sect. 5 through 8, we give an overview of the pathways that have been linked to sleep homeostasis , with an emphasis on clock genes and adenosine . The list provided is, however, non-exhaustive and many other systems are currently under investigation. Even though these studies brought essential information in the understanding of sleep homeostasis, a unifying picture on its genetic control has yet to emerge.
Table 1
Genes investigated through reverse genetic studies for their involvement in sleep homeostasis
Family | Gene | Species | Homeostatic phenotype | References |
---|---|---|---|---|
Clock genes | Clock | Mouse | Yes | Naylor et al. 2000 |
Fly | Yes | Shaw et al. 2002 | ||
Bmal1 | Mouse | Yes | Laposky et al. 2005 | |
Cycle | Fly | Yes | Shaw et al. 2002 | |
Npas2 | Mouse | Yes | Franken et al. 2006 | |
Cry1, Cry2 | Mouse | Yes | Wisor et al. 2002 | |
Dbp | Mouse | No | Franken et al. 2000 | |
Per1, Per2 | Mouse | No | Kopp et al. 2002 | |
Mouse | No | Shiromani et al. 2004 | ||
Fly | Yes | Shaw et al. 2002 | ||
Per3 | Mouse | No | Shiromani et al. 2004 | |
Mouse | Yes | Hasan et al. 2011 | ||
Mouse | Yes | Hasan et al. 2012 | ||
Human | Yes | Viola et al. 2007 | ||
2–Dec | Mouse | Yes | He et al. 2009 | |
Adenosine | A 1 R | Mouse | No | Stenberg et al. 2003 |
Mouse | Yes | Bjorness et al. 2009 | ||
Mouse | Yes | Halassa et al. 2009 | ||
A 2 R | Mouse | Yes | Urade et al. 2003 | |
dAdoR | Fly | No | Wu et al. 2009 | |
Adk | Mouse | Yes | Palchykova et al. 2010 | |
CD73 | Mouse | Yes | Zielinski et al. 2012 | |
Neurotransmitters | COMT | Human | No | Bodenmann et al. 2009 |
Human | Yes | Goel et al. 2011 | ||
GABAR | Mouse | No | Winsky-Somerer et al. 2009 | |
Mouse | No | Vienne et al. 2010 | ||
Ion channels | Sh | Fly | No | Cirelli et al. 2005 |
Sss | Fly | Yes | Koh et al. 2008 | |
Hk | Fly | No | Bushey et al. 2007 | |
Kcna2 | Mouse | No | Douglas et al. 2007 | |
Signaling pathways | BDNF | Human | Yes | Bachman et al. 2012 |
Stress and immunity | BiP | Fly | Yes | Naidoo et al. 2005 |
Hsp83 | Fly | Yes | Shaw et al. 2002 | |
Synaptic plasticity | Homer1 | Mouse | No | Maret et al. 2007 |
Homer | Fly | Yes | Naidoo et al. 2012 | |
Homer1a | Mouse | No | Naidoo et al. 2012 |
5 Circadian Clock Genes
Although the genes referred to as clock genes are involved in many pathways they are best known (and named) for their role in circadian rhythm generation. Clock genes are transcriptional regulators engaged in negative feedback loops that underlie the molecular circuitry of the circadian clock machinery (Ko and Takahashi 2006). Positive elements of this feedback loop are three factors: CLOCK, NPAS2, and BMAL1. NPAS2::BMAL1 and CLOCK::BMAL1 heterodimers can drive transcription of many genes including the Period (Per1 and –2) and Cryptochrome (Cry1, and –2) genes. PER::CRY protein complexes suppress CLOCK/NPAS2::BMAL1-mediated transcription and thus their own transcription thereby constituting the negative elements in the feedback loop. Additional interactions between these core clock genes and other actors (e.g., REV-ERBα, RORα) at the level of transcription, translocation back into the nucleus, post-translational modifications add further complexity and stability. In Drosophila, the molecular oscillator relies on very similar timing systems using orthologs to the mammalian clock genes (Benito et al. 2007). The central autoregulatory feedback loop is composed of the two factors CLOCK and CYCLE, which are the homologues of the mammalian CLOCK and BMAL1, respectively. The CLOCK::CYCLE heterodimers activates the transcription factors PERIOD and TIMELESS which act as negative regulators like the mammalian PER and CRY proteins, respectively (Tomioka and Matsumoto 2010).
The circadian process and the homeostatic process appear independent (for review see Franken 2013). Rendering animals arrhythmic through lesioning the SCN (Trachsel et al. 1992; Easton et al. 2004) or a light pulse (Larkin et al. 2004), did not affect the increase in EEG delta power after SD . Moreover, studies in humans showed that the sleep–wake dependent dynamics of EEG delta power are little affected by circadian factors (Dijk and Czeisler 1995). Analyses of sleep homeostasis in humans, mice, and flies carrying polymorphism or targeted disruptions of clock genes demonstrate, however, that at least at the molecular level, circadian rhythms and sleep homeostasis are difficult to dissociate (Shaw and Franken 2003; Franken and Dijk 2009). In the following, we will discuss the different clock gene mutants/polymorphisms that have been studied and suggesting a non-circadian role of clock genes in sleep homeostasis .
5.1 Reverse Genetic Studies for Clock Genes
Clock is the first circadian gene identified in a mammal (Vitaterna et al. 1994) and subsequently cloned (Antoch et al. 1997; King et al. 1997). The Clock mutation (Clock Δ/Δ) affects numerous aspects of circadian rhythmicity, including a lengthened circadian period. Clock was also one of the first clock genes for which a role in sleep homeostasis has been claimed (Naylor et al. 2000). Under baseline conditions, Clock Δ/Δ mice showed decreased NREM sleep, associated with a reduction in NREM sleep episode length. When challenged with a 6 h SD, Clock Δ/Δ mice showed a normal NREM sleep rebound but a reduced rebound in REM sleep. The authors suggested that NREM sleep homeostasis was affected by the Clock mutation, because NREM sleep delta energy was reduced both during baseline and during recovery. Because this measure is a function of the total time spent in NREM sleep, the difference has, however, to be largely attributed to the fact that these mice slept less. Moreover, when sleep homeostasis was evaluated according to the time course of EEG delta power , no genotype differences could be observed. Therefore, it remains unclear whether the Clock mutation affects NREM sleep homeostasis . The altered relative increase in REM sleep, which could not be explained by differences in REM sleep amount in baseline, points to an altered homeostatic regulation of REM sleep. In flies, the mutation of the Drosophila Clock homolog mildly affects baseline sleep as well as the response to SD (Shaw et al. 2002). It remains to be determined whether Clock KO mice have a sleep homeostatic phenotype.
In addition to abolishing circadian rhythms in overt behaviors, targeted disruption of Bmal1 leads to profound differences in sleep (Laposky et al. 2005). Under baseline conditions, Bmal1 –/– mice showed attenuated amplitude of the distribution of sleep and wakefulness across the 24 h day as well as elevated NREM and REM sleep amounts. Moreover, this increase in sleep time was accompanied by a more fragmented sleep. The authors found that sleep intensity, determined as the average of absolute levels of EEG delta power in baseline, was increased in Bmal1 –/– mice, a surprising finding given that sleep fragmentation and intensity are usually inversely correlated (Franken et al. 2001). To assess genotype effects on the homeostatic regulation the relative, sleep–wake dependent changes in EEG delta power were analyzed in baseline and after a 6 h SD . As normalization the averaged 24 h baseline level was used. With this normalization higher than wild-type (WT), EEG delta power levels were reached in the light period and lower values in the dark period of baseline. This time course can serve as an example of the problem that exists with the use of this reference value (Fig. 2c), especially because Bmal1 –/– mice sleep overall more and lack the sustained period of wakefulness in the first half of the dark period. As a result, levels of homeostatic sleep pressure can be expected to be constitutively low in Bmal1 –/– mice, consistent with the lower levels of sleep consolidation observed. Nevertheless, the relative increase in EEG delta power was reduced pointing to slower build-up of homeostatic sleep need .
An extreme sleep homeostatic phenotype was observed in Cycle mutants, the fly homolog of Bmal1. Cycle mutant flies showed an exaggerated sleep rebound after SD and died after 10 h of SD (Shaw et al. 2002). This was the first study to show a vital role for sleep in flies. The combination of circadian disruption and alterations in the response to SD in Bmal1 –/– mice and in Cycle mutant flies supports the notion that this clock gene plays a role in both circadian and sleep homeostatic processes.
In mammals, CLOCK is the main component of the circadian machinery in the SCN and peripheral organs, whereas in peripheral brain regions (i.e., peripheral to the SCN), it is substituted by its paralog NPAS2. CLOCK and NPAS2 are similar in amino acid sequence, share BMAL1 as an obligate partner, bind to the same DNA recognition element, are suppressed by CRY proteins, and commonly depend on favorably reducing ratio of NAD factors (Rutter et al. 2001). Because NPAS2 acts both as a sensor and an effector of intracellular energy balance, and because sleep is thought to correct energy imbalance incurred during waking, Npas2 might be a candidate for a sleep homeostatic gene. In contrast to Clock Δ/Δ mice, which showed a lengthened circadian period (Vitaterna et al. 1994), Npas2 –/– mice (Garcia et al. 2000) have a shorter period of activity (Dudley et al. 2003) whereas Clock –/– /Npas2 –/– double KO are completely arrhythmic (DeBruyne et al. 2007). This suggested that CLOCK and NPAS2 function as redundant regulators of circadian behavior. With regard to sleep, Npas2 –/– mice were found to sleep less in the latter half of the baseline dark period, a time of day at which sleep need is high and WT mice showed a consolidated period of sleep (i.e., a nap) conceivably to discharge accumulated sleep pressure (Franken et al. 2006). After SD , these mice were incapable in initiating the appropriate compensatory behavior during the circadian phase in which mice are usually awake, i.e., the dark period (Franken et al. 2006). They regained less NREM sleep in the following hours after SD , and the EEG delta power after SD was smaller. Based on simulation analysis, the estimated rate at which EEG delta power increases during wakefulness tended to be slower in Npas2 –/– mice. In conclusion, NPAS2 affects the homeostatic regulation of NREM sleep and, in contrast to Clock Δ/Δ mice, homeostatic regulation of REM sleep was not affected.
Mice lacking both Cry1 and Cry2 genes lack a functioning circadian clock and are behaviorally arrhythmic when kept under constant conditions (van der Horst et al. 1999; Vitaterna et al. 1999). Under baseline light–dark conditions, Cry1,2 –/– mice spent more time in NREM sleep and sleep was more consolidated (i.e., longer uninterrupted episodes of NREM sleep). This increased consolidation of sleep was accompanied by a higher level of EEG delta power . In contrast to Npas2 –/– mice, simulation analysis revealed that these higher levels of EEG delta power were due to a faster rate at which EEG delta power increases during wakefulness in Cry1,2 –/– mice, compared to control mice (Wisor et al. 2002). The apparent higher sleep drive during baseline could also explain that after SD, Cry1,2 –/– mice did not exhibit significant increases in NREM and REM sleep time, and only a brief and smaller increase in EEG delta power . These results were not observed in the Cry1 –/– and Cry2 –/– single KO mice (Wisor et al. 2008), consistent with the functional redundancy between the two CRY proteins observed for circadian rhythms (van der Horst et al. 1999; Vitaterna et al. 1999).
Albumin D-binding protein (DBP) is a PAR leucine zipper transcription factor that is expressed according to a robust circadian rhythm in the SCN. Mice lacking DBP display a shorter circadian period in locomotor activity and are less active (Lopez-Molina et al. 1997). Although DBP is not essential for circadian rhythm generation, it does modulate the expression of core clock components as well as important clock outputs (Bozek et al. 2009). In particular, in vitro and in vivo studies have shown that the expression of the Per and Cry1 genes is modulated through activation of the D-box in their promoter, an element that is bound by DBP (Vatine et al. 2009; Yamajuku et al. 2010, 2011; Ukai-Tadenuma et al. 2011; Mracek et al. 2012). Mice lacking the Dbp gene showed an altered sleep–wake distribution in baseline with reduced amplitude of the daily changes (Franken et al. 2000). These findings suggest that DBP, in addition to changing the period of the circadian clock, modifies the strength of the SCN output signal, which governs the distribution and consolidation of sleep and wakefulness over the day (Dijk and Czeisler 1995). In addition, Dbp –/– mice showed a decreased NREM sleep consolidation and EEG delta power amplitude, suggesting an overall lower sleep propensity . Computer simulations predicting the time course of EEG delta power demonstrated that the difference in EEG delta power was, to a large extent, due to a reduction in the circadian amplitude of the distribution of sleep and wakefulness and not to an altered dynamics of the homeostatic regulation of EEG delta power (Franken et al. 2000). This study demonstrated that DBP mostly affects those aspects of sleep that are known to be under direct circadian control but leaves the homeostatic regulation of NREM sleep unaffected. Nevertheless, similar to Clock Δ/Δ mice, Dbp –/– mice showed a reduced compensatory rebound in REM sleep pointing to an altered homeostatic regulation of REM sleep.
Per1 and Per2 represent key element of the mammalian molecular clock in the SCN, and their disruption leads to gradual loss of rhythmicity under constant conditions. Two studies have investigated a possible role of Per genes in sleep homeostasis using mutant lines. In a first study, Kopp and colleagues observed that the main differences between genotypes occurred in the distribution of sleep and wakefulness over the day under baseline conditions (Kopp et al. 2002). Per1 mutants slept less than WT in the dark period, whereas Per2 mutants slept less before dark onset. This earlier decrease of sleep in Per2 mutants is consistent with the earlier onset of the active phase in these mice. Although the authors concluded that both Per1 and Per2 mutants mice had intact sleep homeostasis , SD led to a larger increase in total sleep time during recovery in Per2 mutants. Moreover, both mutant lines showed earlier onset of NREM and REM sleep rebound after the SD and Per2 mutant mice showed a larger relative increase in sleep time in the recovery dark period. Finally, lower levels of EEG delta power were reached during recovery sleep immediately following the SD . Together these observations would argue for altered dynamics of the sleep homeostat. In a second study, Shiromani and colleagues recorded sleep in Per1, Per2, and Per1,2 double mutant animals (Shiromani et al. 2004). Similar to the previous findings, they observed an altered sleep–wake distribution especially in Per2 mutant and double mutant mice. More importantly, the authors observed that after 6 h SD, the rebound in EEG delta power was longer lasting in Per1 and Per1,2 mutant mice. Although the authors did not comment on the differences in the magnitude of the response, the relative increase in EEG delta power after SD seemed also larger in Per1 and Per1, 2 mutant mice. Thus in contrast to the authors’ conclusion these clock genes do seem to alter the dynamics of the sleep homeostatic process albeit the genotype effects on the increase in EEG delta power seem opposite to those reported by Kopp et al. (Kopp et al. 2002). Also Drosophila Per mutant flies exhibit a homeostatic phenotype, with an increased sleep rebound compared to WT flies (Shaw et al. 2002). Together, these data support a role for Per1 and Per2 signaling in sleep homeostasis although results in mice deserve further investigation.
While, Per1 and Per2 are widely considered to be integral part of the core circadian clock machinery, the role of the third Per homologue, Per3, in maintaining circadian rhythmicity is controversial. This controversy comes from observations that in mice, the absence of Per3 has only a subtle effect on circadian rhythm phenotype (Shearman et al. 2000; reviewed in van der Veen and Archer 2010). Disruption of Per3 in mice seems to alter (non-circadian) light-sensitivity which in turn could result in some of the circadian phenotypes reported such as a shortening of the free-running period under constant dark conditions (Van der Veen and Archer 2010). In the aforementioned study Shiromani and colleagues also recorded sleep in Per3 mutant mice but did not note any differences in the increase of time spent asleep or EEG delta power during recovery from SD (Shiromani et al. 2004). However, in a more recent paper homeostatic sleep phenotypes were reported for Per3 –/– mice (Hasan et al. 2011). Differences in the rebound in REM sleep were found during recovery from SD . These differences must, however, be attributed to baseline differences at the time the SD was performed because the recovery dynamics in REM sleep time as well as the levels reached in recovery did not differ. The resulting gain–loss curve resembles the hypothetical example presented in Fig. 2b′. Also the levels of EEG delta power reached after SD did not differ between Per3 –/– and wild-type mice suggesting that the rate of increase of homeostatic sleep pressure during the SD was similar. Nevertheless, EEG delta power during the dark periods of baseline and recovery was significantly higher in Per3 –/– mice, a difference that could not be explained by alterations in the amount or distribution of EEG delta power between the two genotypes. Thus like its role in circadian rhythms, a critical role for Per3 in the homeostatic regulation of sleep in the mouse remains questionable.
In humans, a primate-specific variable number tandem repeat (VNTR) polymorphism in the Per3 gene was investigated a few years ago for its role in circadian rhythmicity (Jenkins et al. 2005). A 54-nucleotide coding-region segment of the gene is repeated either 4 or 5 times, leading to different alleles. Initially, an association study revealed a higher frequency of people homozygous for the 5-repeat in morning types than in evening types suggesting possible functional role for Per3 in sleep and circadian behavior (Archer et al. 2003). In follow-up studies investigating sleep phenotypes in Per3 4/4 and 5/5 carriers, it was found that this polymorphism affected electrophysiological and behavioral markers of sleep homeostasis such as sleep latency, EEG delta power , and the decrement in waking performance (Viola et al. 2007, 2012), executive function (Groeger et al. 2008), and neurobehavioral performance after sleep restriction (Rupp et al. 2012). This was the first evidence in human of a non-circadian role of clock genes in sleep regulation (Dijk and Archer 2009). While the human Per3 VNTR polymorphism has been linked with differences in sleep homeostasis , cognitive vulnerability to sleep loss, and differences in functional MRI-assessed brain activity in response to sleep loss (Dijk and Archer 2009), none of these studies has shown any association between the Per3 VNTR and any circadian phenotype. Thus, while other core clock proteins may have overlapping roles in both the circadian and sleep systems, PER3 phenotypes from human and animal studies point toward a more prominent role for PER3 in the regulation of sleep homeostasis . A new transgenic mouse line carrying the human Per3 polymorphism in the mouse Per3 gene is currently under investigation, and preliminary analyses seem to highlight similarities with the human sleep homeostatic phenotype (Hasan et al. 2012).
DEC2, a member of the basic helix-loop protein family of transcription factors, by repressing CLOCK::BMAL1 acts as a negative component of the circadian clock. In a family-based candidate gene resequencing study a point mutation in the Dec2 gene (P385R) was found to be associated with extremely early wake-up times and reduced sleep time (He et al. 2009). To examine the effect of DEC2 on sleep, several animal models were constructed. In transgenic mice carrying the human P385R Dec2 gene, both NREM sleep and REM sleep were reduced and sleep was more fragmented in baseline compared to mice carrying the wild-type human Dec2 thus recapitulating the human short sleep phenotype. A 6 h SD in P385R Dec2 mice resulted in a smaller rebound in both NREM sleep and REM sleep, and a smaller relative increase in EEG delta power , compared to the control mice. The loss–gain analyses of the effect of SD on REM sleep duration revealed, however, a dynamics resembling our example depicted in Fig. 2b, indicating that the apparent difference in REM sleep homeostasis might be due to differences to REM sleep in baseline. Also the analyses of the rebound in EEG delta power do not allow for a careful evaluation of genotype differences in the sleep–wake dependent dynamics; given the poor time resolution over which EEG delta power was calculated (6 h intervals), it is impossible to establish whether the reported smaller increase after SD is due a slower build-up of sleep need during the SD , a faster decrease of sleep need during recovery sleep, or to differences in NREM sleep during the initial 6 h of recovery sleep. In both P385R Dec2 transgenic mice and in Dec2 KO mice the compensation of NREM sleep duration lost during the 6 h SD was compromised pointing to a role of Dec2 in this aspect of sleep homeostasis specifically. In line with the human and mouse short sleep phenotype, transgenic flies expressing the murine Dec2 gene carrying the human mutation P385R slept less than control flies (He et al. 2009). Unfortunately, the response to a homeostatic challenge (i.e., SD) was not assessed in these flies nor in humans. Although more data are needed, these data suggest that the P385R genotype shortens sleep independent of species background.
5.2 Clock Gene Expression Changes as a Function of Sleep
In line with the findings that several clock genes are involved in the control of sleep homeostasis , several studies have shown that expression of some clock genes in the mouse brain varies as a function of sleep propensity . SD results in a constellation of changes in gene expression in the brain (Cirelli et al. 2006; Terao et al. 2006; Maret et al. 2007; Thompson et al. 2010) that are sleep–wake related and thus, can serve as biomarkers for sleep loss and recovery (see Sect. 8). Among the transcripts that exhibit sleep-related changes in the cortex are the circadian genes Per1, Per2, and Dbp (Wisor et al. 2002; Franken et al. 2006, 2007; Mongrain 2010; Curie et al. 2013). The expression of Per1 and Per2 increases according to a linear function of the duration of the time mice are kept awake, whereas the expression of Dbp decreases (Wisor et al. 2002; Franken et al. 2006, 2007). These SD -induced changes were, however, strongly dependent on the time of day at which the SD was performed (Curie et al. 2013). Moreover, the SD -associated increase in corticosterone proved to be an important contributor to these increase in clock gene expression such that the expression of Per1 did no longer increase after SD in adrenalectomized mice (Mongrain et al. 2010). In addition to stress, SD seems to be able to alter the clock gene expression through directly modifying DNA-binding of the transcription factors CLOCK, BMAL1, and NPAS2 to specific E-boxes in clock gene promoters (Mongrain et al. 2011).
Together, the data from human, mice, and flies have contributed to the notion that, at the molecular level, sleep homeostasis and circadian rhythms are not independent, and that clock genes participate in both aspects of sleep regulation. According to the fact that several clock genes belong to a class of PAS transcriptional regulators that can act as sensors of environmental signals (Gu et al. 2000), we proposed that clock genes and their protein products act as molecular sensors and translate homeostatic sleep need into transcriptional signals at the cellular level, independent of the circadian machinery (Franken and Dijk 2009). Especially, the sensitivity of the clock gene machinery to redox state and metabolism (Bass and Takahashi 2010) is of interest in the context of sleep homeostasis as maintaining metabolic balance is often mentioned as a potential key function of sleep.
6 Genes of the Adenosine Pathway as Homeostatic Regulators?
Adenosine is an inhibitory neuromodulator that has been proposed to act as a homeostatic regulator of sleep and to link humoral and neural mechanisms of sleep–wake regulation (Porkka-Heiskanen et al. 1997, 2000; Basheer et al. 2004; Kalinchuk et al. 2011). In mammals, four subtypes of G-protein coupled receptors mediate the effects of adenosine : A1R, A2AR, A2BR, and A3R and two of them have been investigated for their role in sleep homeostasis (Fredholm et al. 2001). It is thought that A1R are responsible of the sleep effects of adenosine (Rainnie et al. 1994; Benington and Heller 1995), but a careful study performed in A1R KO mice showed that the homeostatic aspect of sleep regulation was unaltered in animals lacking A1R (Stenberg et al. 2003). This study revealed that mice lacking A1R had normal baseline sleep–wake distribution and responded normally to sleep pressure , with NREM sleep rebound and EEG delta power rebound being similar to WT animals. More recently, a conditional central nervous system KO of this adenosine receptor was created. A1R-/- mice were found to have reduced EEG delta power in NREM sleep during baseline, as well as during sleep restriction (Bjorness et al. 2009) whereas the sleep–wake distribution and amount were preserved. More importantly, when the mice were allowed to sleep for 2 h following 4 h of sleep restriction, the relative increase in EEG delta power during NREM sleep above baseline levels was smaller in the KO mice although the amount of time spent in NREM sleep was similar to WT. The authors suggested that the elevated sleep need signaled by adenosine is, at least in part, mediated through the A1R. However, the implemented sleep restriction protocol allows mice to recover during the 2 h sleep opportunity windows between the 4 h SD s, importantly affecting the level of EEG delta power . Moreover, the authors observed a general decrease in EEG delta power when calculated over all states (wakefulness, NREM and REM sleep) which might indicate a specific effect of the gene on general electrical brain activity rather than an effect on sleep homeostasis . Given the contradictory findings, the role of A1R in sleep homeostasis remains unclear. It might be interesting to generate inducible KO animals to study the loss of A1R in the adult stage only, thereby circumventing several potential confounds such as developmental compensation. The accumulated evidence indicates that besides A1R also A2AR contributes to the effects of adenosine on sleep. A preliminary report indicated that A2AR KO mice do not show a NREM sleep rebound following 6 h SD , revealing an altered sleep homeostasis in these mice (Urade et al. 2003). Changes in EEG delta power were not reported in these mice. Along the same line, a human study revealed that a distinct polymorphism in the gene encoding the A2AR, Adora2, modulates individual sensitivity to subjective and objective effects of caffeine on sleep (Rétey et al. 2007). It would be of interest to test the effect of this polymorphism on the homeostatic process by submitting individuals carrying the different polymorphism to a SD . In Drosophila, one single adenosine receptor gene has been identified, dAdoR, that is most closely related to the mammalian Adora2 gene. Study in dAdoR mutant flies revealed that sleep was not affected by the mutation, neither in baseline, nor in recovery from SD (Wu et al. 2009). These results suggest that in flies, adenosine receptors are not required to maintain sleep homeostasis .
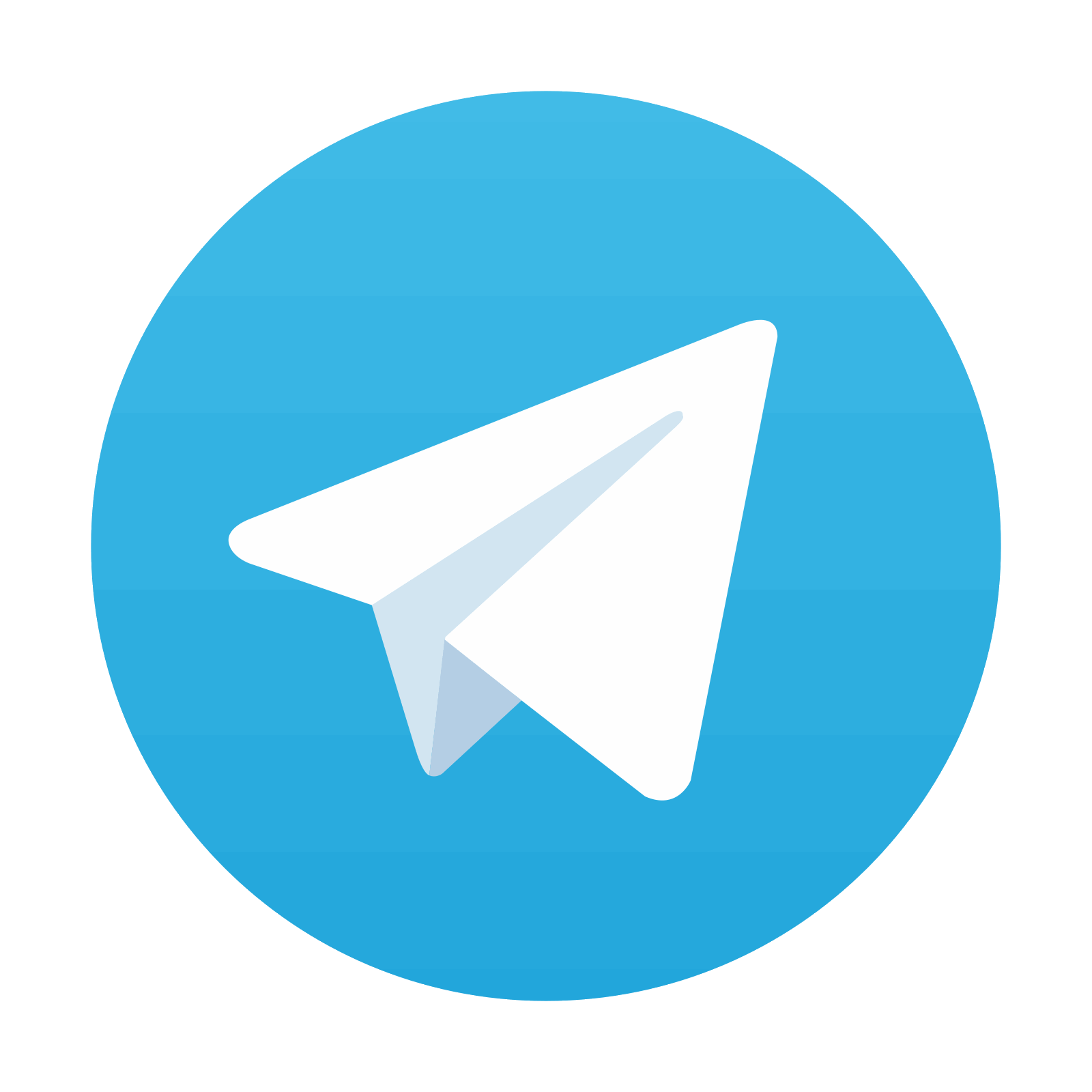
Stay updated, free articles. Join our Telegram channel

Full access? Get Clinical Tree
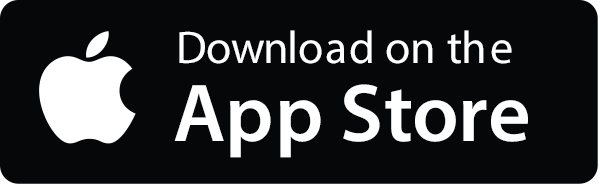
