Introduction
Neurocritical care monitoring generates enormous amounts of various kinds of data from multiple sources. The information obtained serves the purpose of detecting secondary brain insults and guiding the immediate management of the individual patient. Stored data also may be used for quality assurance and to capture the intracenter and intercenter management variation that can be fundamental to being able to show positive effects in phase 3 trials. Furthermore, the collection and storage of monitoring data into databases fosters research, for example, defining critical threshold levels for managing raised intracranial pressure (ICP). Such research may increase the understanding of secondary brain injury mechanisms and define the proper use and value of new monitoring techniques. Ultimately, this may lead both to better detection of dangerous secondary brain insults and to new treatment approaches. If the future expectations of neurocritical care and multimodality monitoring are to be realized, it is essential to improve information processing and to standardize data acquisition storage and analysis of data. In these aspirations, information technology (IT) plays a crucial role.
Information Technology
IT is defined by the Information Technology Association of America (ITAA) as “the study, design, development, implementation, support or management of computer-based information systems, particularly software applications and computer hardware.” It deals with the use of electronic computers and computer software to convert, store, protect, process, transmit, and securely retrieve information. Informatics in the neurosciences was first applied to basic science research but there now are several large databases, such as the Alzheimer’s Disease Neuroimaging Database (ADNI) or the Glioma Molecular Diagnostic Initiative (GMDI), that provide researchers in any location open access to data that can drive research forward. It is clear that the computer science expert also must be included in the neurointensive care team today and in the future. The application and development of IT in the neurocritical care unit (NCCU) is the challenge faced to further develop neurocritical care. In particular, informatics in the NCCU can be leveraged to help provide insight into the disorders that are treated there and so help design new treatment strategies.
There is accumulating evidence that demonstrates that IT can improve patient health care. Bates and Gawande describe trials in which technology was associated with a reduction in medication errors and errors related to transitions of care, and in which technology aided in earlier detection of adverse events. For example, Kupermann et al., in a randomized controlled trial of technology for early detection of adverse events, showed a reduction in time to treatment of 11% and a 29% reduction in the duration of dangerous conditions to patients. Rosenfield conducted a study of IT-based remote monitoring of a 10-bed intensive care unit (ICU) and reported a reduction in mortality of greater than 40% and a reduced length of stay of 30% compared with historical controls.
Critics of this type of research point out that better resolution of events is of no value unless their direct management influences clinical outcome. Single-center studies with small numbers of patients are not suited to answer such questions, and multicenter randomized controlled trials to validate IT-driven management are not readily funded nor easily justified as a research priority. Paradoxically, the patient populations that may benefit most from better IT-based event detection, such as the brain injury population, are the most challenging in which to conduct a controlled management trial because of continuing intercenter management variation fostered in large part by a lack of evidence for any type of effective therapy.
Nevertheless, indirect evidence is available and continues to become available. There are increasing numbers of reports that indicate the importance of providing specialist neurocritical care in the management of traumatic brain injury (TBI) patients. For example, the report by Patel of 2300 patients treated in nonneurosurgical hospitals showed a 2.15-fold increase in the odds of death compared with those treated in a neurosurgical center. These studies do not indicate which aspects of critical care management are key, but management (whether surgically or medically focused) aimed at earlier detection and treatment of adverse events must in part be responsible. In support of this and despite criticism from enthusiasts of evidence-based medicine (EMB), neurointensive care centers with a track record in the aggressive management of secondary insults continue to report improvements in outcome statistics of patients compared with historical controls.
The Brain Monitoring with Information Technology (BrainIT) group has been a strong proponent for adopting IT methods for the early detection and management of secondary insults in patients with brain injury ( www.brainit.org ). Analyses in progress from this group on the minute-by-minute physiologic data of 200 head-injured patients obtained from 22 neurointensive care centers across Europe also may indicate that more complex summary measures, such as the Pressure-Time Index, better relate to clinical outcome than do simple measures such as the mean, median, or total duration of insult burden.
Researchers continue to hope for definitive controlled trial evidence that IT-led management yields improved patient outcome, but experience to date of funding and conducting such studies is limited. Perhaps research time and funds are better spent on conducting trials of new forms of management without the need to trial new health care support systems as well? However, to demonstrate benefit of a therapy requires that all other aspects of management be homogenous. In conditions as complex as TBI and subarachnoid hemorrhage, there remain many opinions as to what constitutes “standard” or “optimal” management, and without information about this, even effective therapies may appear to be ineffective in clinical practice because of variation in care. The authors are inclined to agree with the sentiments of Socrates as portrayed in the “letter of dissent” when arguing against “Enthusiasticus” that perhaps the loudest supporters of EBM are the hospital accountants keen to keep health care costs down. There is no question that better monitoring and event detection technology for health care is needed and that more research to optimize that technology is also needed, but should their adoption depend upon large-scale clinical trials? Perhaps now the questions to focus upon are no longer if but when and no longer why but how .
Data Acquisition
Data acquisition is the sampling of the real world to generate data that can be manipulated by a computer. Collection of minute-by-minute physiologic monitoring data is routine in many NCCUs. However, there are no agreed-upon standards to define the collection of data. Cerebral perfusion pressure (CPP = Blood pressure[BP] − ICP) monitoring is a good example. When CPP values are reported, how does one correct for policies that differ between centers on the level to which the BP transducer is zeroed? Tracking changes in bed tilt (which affects the size of hydrostatic pressure gradients between the head and heart) is also a significant technical issue without defined standards. As a result, it is often difficult to compare even the most common forms of monitoring when data are presented. Development of standards and guidelines in this area is an important step for the future design and conduct of trials of new intensive care monitoring and treatment methods. In an attempt to standardize how to approach standardizing correction of hydrostatic pressure gradients, the BrainIT group has published a BrainIT web standard ( http://www.brain-it.eu/ ).
With this background in mind, certain individuals working within the field of TBI research met to discuss the foundation and development of a network to create an IT-based infrastructure aimed at improving the standards for multicenter studies of monitoring and managing TBI patients during their acute stay in intensive care. (These same principles can be adapted to patients with other disorders admitted to the NCCU.) It was agreed that a different approach was needed, one that focused on using IT-based methods to increase the resolution of data capture and the quality and validation of data captured. The pervasive nature of the Internet and extensive use by clinicians of email systems fostered the creation of such a network as an Internet-based e-Infrastructure: the BrainIT group.
The BrainIT group works collaboratively to develop standards to collect and analyze of data from brain-injured patients toward providing a more efficient infrastructure to assess new health technology. In several international meetings, the group has defined a core dataset designed to be collected using PC-based tools and to provide a common minimal dataset for all studies, regardless of the underlying research question. This data definition period was funded as part of a European Community study (QLGT-2000-00454). The meetings brought together clinical and scientific experts from the domain of TBI basic research and of multicenter clinical trials, such as the European Brain Injury Consortium, as well as representatives from the medical device and pharmaceutical industries. A series of meetings and workshops conducted over 1 year enabled the group to define a minimum set of data that could be collected from all patients with TBI, which would be useful in most research projects conducted in this population of patients. To facilitate discussion, the core dataset was subdivided into four logical groups: (1) demographic and clinical information, (2) minute-by-minute monitoring information, (3) intensive care management information, and (4) secondary insult treatment information.
From these meetings a consensus dataset was formed, which includes nine categories:
- 1.
Demographic and one-off clinical data (e.g., pre-neurosurgical hospital data, first and worst computed tomography scan data)
- 2.
Daily management data (e.g., daily summary measures of the use of sedatives, analgesics, vasopressors, fluid input/output balance)
- 3.
Laboratory data (e.g., blood gas, haematology, biochemistry data)
- 4.
Event data (e.g., nursing maneuvers, physiotherapy, medical procedures such as line insertion, calibrations)
- 5.
Surgical procedures
- 6.
Monitoring data summary (e.g., type and placement location of ICP sensors, BP lines)
- 7.
Neuroevent summary (e.g., Glasgow Coma Scale [GCS], pupil size and reactivity)
- 8.
Targeted therapies (e.g., mannitol given for raised ICP, pressor given for arterial hypotension)
- 9.
Vital monitoring data (e.g., minute-by-minute BP, ICP, systemic arterial oxygen saturation [SaO 2 ] collected from the bedside monitoring)
The full details of the core dataset definition and the collaboration structure of the group can be found in the BrainIT publication: BrainIT Group: Core Concept and Data Definition . This same approach has been undertaken in the United States, sponsored by several federal agencies including the National Institutes of Health to develop the “Common Data Elements” ( http://www.commondataelements.ninds.nih.gov.easyaccess2.lib.cuhk.edu.hk/ and ). Unique to other dataset definitions, the BrainIT core dataset defined a special approach to quantify secondary insult management. This is medical management therapy given to patients specifically to treat secondary insults that occur despite the patient’s baseline intensive care medical management. To distinguish therapy given to patients to treat secondary insults from those of baseline intensive care, the authors have devised a coding system that allows specific categories of therapy to be assigned a “therapy target.” For example, vasopressors may be given to treat systemic hypotension or to treat reduced CPP secondary to raised ICP. Choosing an appropriate target for each secondary insult therapy will enhance the usefulness of the database on medical therapy. Each therapy must be assigned a target chosen from a drop-down list. If drugs are given, then one can indicate continuous infusion if drugs are delivered by a continuous infusion pump or one can indicate boluses if it is delivered noncontinuously. Figure 43.1 summarizes the minimum choice of therapy categories and associated targets for the BrainIT core dataset. This therapy-tracking model is designed to be implemented easily in software.

It is one thing to define on paper a dataset and another to actually collect the data. Although a paper-based feasibility exercise established some baseline information, the acid test was still to develop a series of IT-based tools to collect the core dataset and to prospectively trial the collection of core data from a number of centers with NCCUs.
A 3-year follow-up EC-funded study (QLGC-2002-00160) enabled the group to develop IT methods to collect the core dataset and to assess the feasibility and accuracy to collect this core dataset from 22 neurointensive care centers. The main data collection instrument for the episodic nonmonitoring data was a personal digital assistant (PDA)-based data collection tool.
Bedside nursing staff entered clinical data on hand held PDAs that supported the BrainIT core dataset definition through a Java Struts-based tool. This allowed the core dataset to be entered by roaming research nurses using a set of PDA documents accessed via a series of buttons and tabs. With this system, indicators were present that showed data documents that were fully complete, partially complete, or totally incomplete. When convenient, the PDA was connected to a docking station and a client program allowed viewing and saving of patient data collected. An anonymization routine removed patient identification elements from the collected data and labeled the patient data file with a unique BrainIT study code generated from the BrainIT website. A local database held in each center linked the anonymized data to local center patient ID information that was needed during the data checking stage of the study. The multicenter ethics approval precluded connection of the PC client system holding the data to any computer connected to the Internet. Local research nurses downloaded the anonymized data from the PDA system client PC onto a memory stick or CD and transferred the data to an Internet-connected PC for upload of the data to the BrainIT database via the website data upload page.
Because this was a multicenter study collecting data from several countries with different languages, a multilanguage implementation was needed to foster ease of use by local nursing staff. A training course was held for the data validation nursing staff in Glasgow on the use of this data collection instrument, which also included using their medical term and language expertise to translate all PDA labels and text output into six European languages (English, French, German, Spanish, Flemish, and Italian). Data could be entered in the local language, exported in an XML file format where a table lookup driven by an XSL transformation converted the data into a standard English language version.
Data validation research nurse staff were hired on a country-by-country basis to check samples of the collected data against gold standard clinical record sources to quantify the accuracy for collection of the BrainIT core-dataset using the group IT-based data collection methods. Anonymized data was uploaded via the BrainIT web-upload services where a server-side data-converter tool converted data from center-based formats into BrainIT data format to generate data category files that were imported into the BrainIT database (SQL Server 2005). A validation request tool sampled 20% of the data sent for each data category and generated a validation request file listing the timestamps and data items to be checked by local data validators. Emails were generated to the data validation staff that contained the validation data requested documents listing the data items to be checked. Data validators entered into a data validation tool the requested data items for checking from source documentation held in each local center. Validation data then were uploaded to the BrainIT data coordinating center via the website and using data validation checking software tools, the validated data were checked against the data items originally sent, from which percentage accuracy data was calculated. Figure 43.2 shows the flow of data between a remote center and the BrainIT database with the validation procedure selecting a random sample of 20% of data items uploaded per data type, which are sent to data validation nurses. The data validation nurses enter requested data into a PC-based validation data tool and upload the data to the data manager, who can then check the accuracy of data for each data category and estimate an overall error rate.

As part of this validation process, in addition to the categorical and numeric clinical data being checked for accuracy, the BrainIT system also assessed the minute-by-minute monitoring data. Random samples of monitoring data channels uploaded (e.g., ICP, SaO 2 ) were selected and validation staff asked to manually enter the hourly recorded values from the nurse’s chart (or local gold standard data source) for the first and last 24-hour periods of bedside monitoring for a given patient for a given channel. These validation values could then be compared with a range of summary measures (e.g., mean, median) from the computer-based monitoring data acquired from the patient.
By late 2008, 384 TBI patients’ core data had been collected from 22 European neurointensive centres. The first 200 patients, data were cleaned and validation analyses conducted. In total, 19,461 comparisons were made between collected data elements and source documentation data. The number of comparisons made per data category was in proportion to the size of the data received for that category with the largest number checked in laboratory data (5667) and the least in the surgery data (567). Table 43.1 summarizes error rates by data class. Error rates were generally less than or equal to 6%, with the exception being the surgery data class where a high error rate of 34% was found.
Data Class | Error Rate (%) | Common Errors |
---|---|---|
Laboratory | 2 | PaCO 2 , FiO 2 value |
Demographic | 4 | Monitoring on arrival at neurosurgery, intubation on arrival at neurosurgery |
Neuro observations | 5 | Pupil Size, GCS versus (code 1 versus unknown) |
Monitoring summary | 5 | ICP type, ICP location |
Daily management summary | 5 | Infusion type (bolus vs infusion or both), drug number (1, >1) |
Targeted therapy | 6 | Nonstandard target, no target specified |
Surgeries | 34 | ICP monitor; surgery for skull fracture or mass lesion |
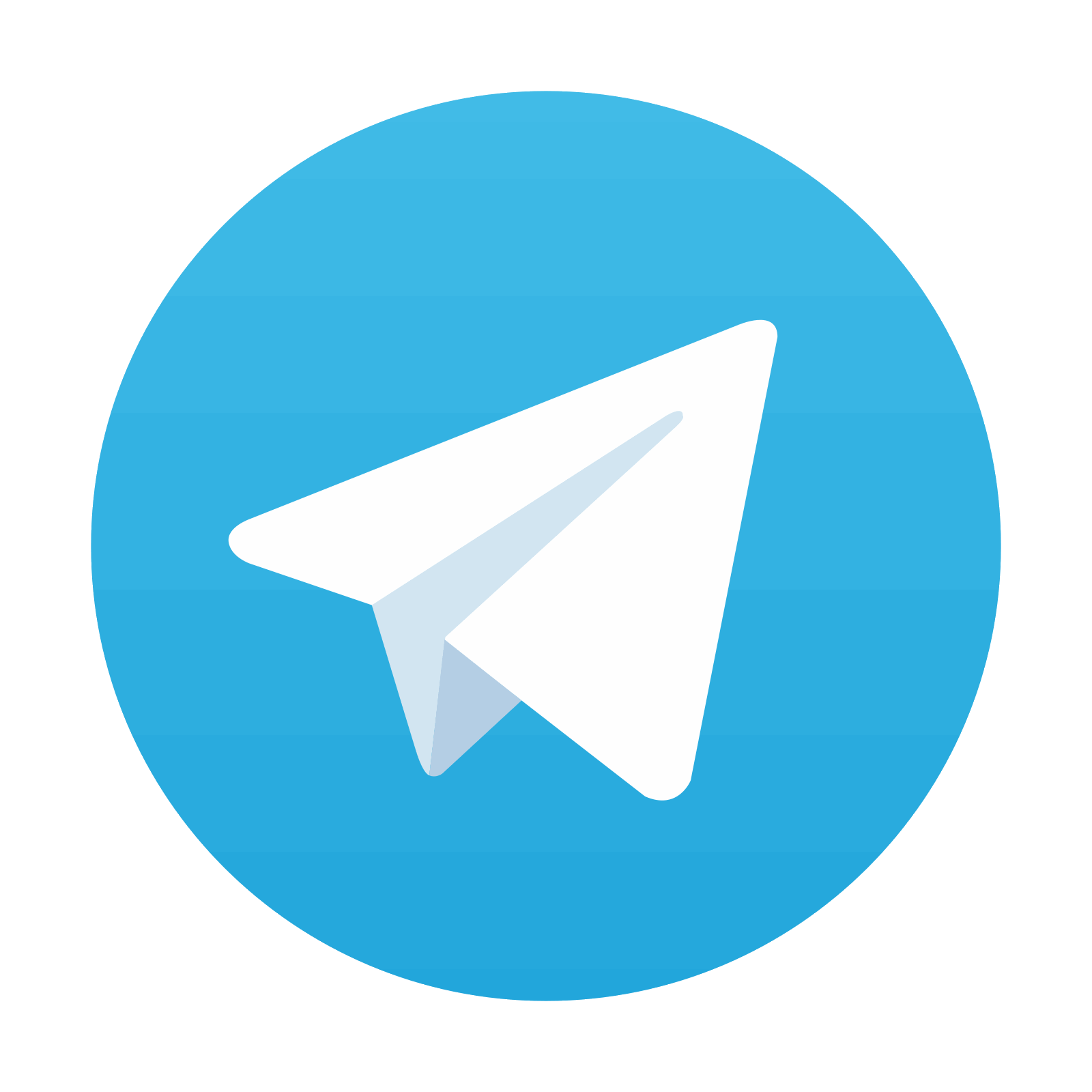
Stay updated, free articles. Join our Telegram channel

Full access? Get Clinical Tree
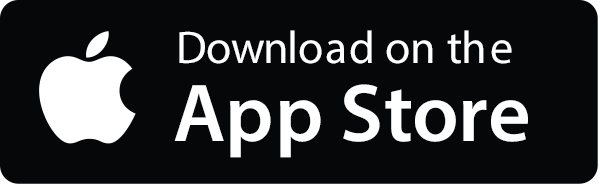

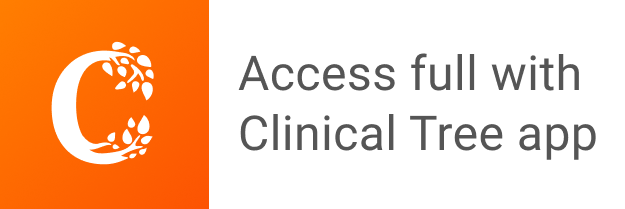