(1)
Cognitive Function Clinic Walton Centre for Neurology and Neurosurgery, Liverpool, UK
Abstract
This chapter examines the methodology of diagnostic test accuracy studies. It emphasizes the critical importance in defining participants and of test methods (diagnostic test and reference standard). The role of pragmatic diagnostic test accuracy studies, recruiting consecutive patients with few exclusion criteria, in comparison to proof-of-concept experimental studies with patient groups preselected by diagnosis, is emphasized.
Keywords
DementiaDiagnostic test accuracy studiesParticipantsTest methodsMethodology is key in the conduct of diagnostic test accuracy studies if their results are to “travel” (i.e. be generalizable, have external validity; Larner 2014a:21–40). Many factors need to be considered in the planning and execution of a diagnostic test accuracy study (Knottnerus 2002) but this should not alienate such studies from the day-to-day arena of clinical practice. Pragmatic diagnostic test accuracy studies (Larner 2012a, 2014a:33–5) may be seen as a reflection of the idiom of clinical practice, and should therefore be well placed to inform day-to-day clinical decision making.
2.1 Participants
2.1.1 Study Population
The study population for a diagnostic test accuracy study should ideally be similar to the population to whom the test is intended to be applied in clinical practice.
2.1.1.1 Inclusion/Exclusion Criteria
The study population for a diagnostic test accuracy study may be defined in terms of inclusion/exclusion criteria, as is the case for randomised controlled trials of new treatments. These criteria are critical since they focus on the research question (Sect. 1.3.1) and determine selection biases (e.g. the clinical disorder evaluated, disease severity, study setting; Knottnerus and van Weel 2002:13; Sect. 1.4.1) and hence whether test results have external validity and are transferable to other clinical situations. With many exclusion criteria, a sample of convenience may result, albeit a very homogeneous sample.
Proof-of-concept studies examining new tests for dementia and cognitive impairment often have stringent inclusion/exclusion criteria (as for randomised controlled treatment trials). These criteria may relate to demographic features, such as patient age (e.g. age <50 years and/or >90 years might be exclusion criteria), as well as disease-related features such as severity (e.g. Mini-Mental State Examination [MMSE] score < 10/30 might be an exclusion criterion, or MMSE >10/30 and ≤26/30 an inclusion criterion). Patient age, gender, and stage of disease may all or individually be possible modifiers of test accuracy.
Comorbidities may also feature amongst exclusion criteria (i.e. any dual pathology), such as the presence and/or history of other neurological disorder (e.g. cerebrovascular disease, head injury), psychiatric illness (e.g. depression), alcohol misuse, or a selection of other general medical and neurological disorders which might potentially impact on cognitive function (of which there are many: Larner 2013a). Certain prescribed medications may also be exclusion criteria, perhaps particularly those with anticholinergic actions which may impact on cognitive performance in both the short and long term (Hejl et al. 2002; Gray et al. 2015).
Such criteria relating to comorbidity can be problematic: for example, since cerebrovascular disease (at least that evident on brain imaging) is often comorbid with Alzheimer’s disease, and a mixed picture may be the most common neuropathological finding in studies of cohorts of patients with dementia (e.g. Neuropathology Group of the Medical Research Council Cognitive Function and Ageing Study (MRC CFAS) 2001; Schneider et al. 2009), significant numbers of potential study participants might be excluded by application of the criterion of “no cerebrovascular disease”, rendering study results less generalizable than if such patients were included as study participants. Likewise, dementia and depression are often comorbid (Wragg and Jeste 1989; Lundquist et al. 1997), and clinically diagnosed depression is not uncommon in patients attending memory clinics (e.g. Knapskog et al. 2014; Hancock and Larner 2015).
Participant ethnicity or, perhaps more pertinently, culture may also be a relevant factor in diagnostic test accuracy studies. Testing individuals with cognitive screening instruments developed in the English language may be difficult if English is not the participant’s first language, hence the need for translation of many commonly used cognitive screening instruments, such as the Addenbrooke’s Cognitive Examination and its iterations (Davies and Larner 2013) and the Montreal Cognitive Assessment (see www.mocatest.org), into different languages (methodologies to undertake such translations are available, e.g. Beaton et al. 2000). The need not only to translate items but also to develop different normative data for different cultural groups has long been understood (Kelly and Larner 2014), but this is still lacking for many of the standard neuropsychological batteries. A number of cognitive screening instruments are claimed, sometimes on the basis of cultural modification and cross-cultural testing, to be culture-fair, such as the Clock Drawing Test, the Mini-Cog, the 7-minute screening battery, and the Time and Change test (Parker and Philp 2004). It is accepted by many neuropsychologists that whilst testing can be language free it cannot be culture free.
Another issue arising in the consideration of study participants is the inclusion of a control group. Some proof-of-concept studies include a “normal” control group, perhaps made up of the spouses of patients attending the clinic, other patients (e.g. attendees at orthopaedic or gynaecology clinics, deemed unlikely to harbour cognitive disorders), or specific institutional panels of control subjects (e.g. the UK Medical Research Council subject panel; Mathuranath et al. 2000). Of course these study participants are not necessarily those amongst whom it is clinically sensible to suspect the target disorder (phase II question: Sackett and Haynes 2002:24). By exaggerating the contrast between patients and controls (ideal or extreme contrast settings), test specificity will be inflated (Sect. 3.3.2). Another rider is that a control group comprising individuals with “normal ageing” may harbour individuals with subclinical disease, a problem generic to any study of age-related signs (Larner 2012b). Knottnerus and van Weel (2002:6) argue that for new tests it is still possible to define an appropriate control group to whom the test is not applied to investigate influence on prognosis.
Pragmatic diagnostic test accuracy studies ideally minimise exclusion criteria by including consecutive patients among whom it is clinically sensible to suspect the target disorder in the study cohort. This may be all-comers if a diagnosis of cognitive impairment or dementia is possible, or for specific disorders those in whom this disorder falls within the differential diagnosis on clinical assessment (Sect. 1.3.1.2). Clinicians do not have the option (luxury?) in day-to-day practice of excluding patients from assessment on the grounds that they happen to have had a stroke, or head injury, or misused alcohol at some stage in their lives. Patients referred to clinic with a pre-existing dementia diagnosis (e.g. for evaluation of new-onset seizures, or behavioural disorder) might reasonably be excluded in studies which are examining diagnostic test accuracy in previously undiagnosed patients (Larner 2015a); likewise those receiving medications used in the treatment of cognitive disorders such as cholinesterase inhibitors or memantine.
For studies dependent upon an informant for eliciting neurological signs (e.g. head turning sign: Ghadiri-Sani and Larner 2013; Larner 2012c) or collateral information about the patient (e.g. IQCODE: Hancock and Larner 2009a; AD8: Larner 2015a; Zarit Burden Interview: Stagg and Larner 2015), patients attending alone will be excluded, unless the necessary information can be obtained by other means (e.g. telephone interview, email).
Application of stringent inclusion/exclusion criteria ensures that heterogeneity in the study population is minimised. It also facilitates matching (as in case-control studies) for factors such as patient age and education level. An inevitable corollary of consecutive patient selection in pragmatic studies is greater heterogeneity which precludes such matching.
2.1.1.2 Study Setting: Disease Prevalence
Study setting is an important consideration, since it will determine the nature of the study population.
Broadly speaking three types of setting may be considered for diagnostic test accuracy studies: community; primary care; and secondary care. It may be important to distinguish between these settings, for example in meta-analyses of tests which may be applied in any one of these settings, such as the Mini-Mental State Examination (MMSE; Mitchell 2009, 2013).
Study setting determines disease prevalence (Sect. 4.1.2.3), which is equal to the pretest probability of the target disease (Sackett and Haynes 2002:28, 29 table, 33).
For dementia, community and primary care settings have a low prevalence of disease (hence lower pretest probability) whereas secondary care settings, particularly dedicated memory or cognitive disorders clinics, may have a high prevalence (and hence higher pretest probability). The latter may sometimes have disease prevalence around 50 % (e.g. Steenland et al. 2010), although in this referral setting prevalence may change over time, possibly as a consequence of national policies related to dementia (Larner 2014b; see Sect. 4.1.2.3). Disease prevalence has an influence on test accuracy and predictive values (Sects. 3.3.1 and 3.3.3).
Because of these differences in prevalence according to setting, there may be different requirements for diagnostic test instruments: ideally in primary care, tests with high sensitivity are desirable to ensure that no cases are missed (false negatives avoided) but at the risk of false positives (e.g. Upadhyahya et al. 2010), whilst in research settings high specificity and avoidance of false positives may be more desirable (Dubois et al. 2014; Sect. 3.3.2).
The measured disease prevalence (pretest probability) is the a priori or prior probability of correct classification without application of any diagnostic test. It allows calculation of the pretest odds of disease:
Comparing pretest odds with the posttest odds (Sect. 3.3.4) gives an indication of the informativeness of a test (the ratio of these odds defines the likelihood ratio): a large change from pretest to posttest odds suggests a highly informative test. Pretest probability is also the basis for calculating another test parameter, the net reclassification improvement (NRI; Sect. 3.3.1).

Although disease prevalence has only one specific value in any particular study, it is possible to make calculations for variable disease prevalence, or equivalent prevalence. This can be informative about test performance in settings other than that in which the reported study is undertaken.
2.1.2 Recruitment: Study Design (Cross-Sectional vs Longitudinal)
The methods by which patients are recruited to a diagnostic test accuracy study must be made transparent.
As mentioned, some studies have significant numbers of inclusion and exclusion criteria for study subjects (Sect. 2.1.1.1). In this situation, recruitment may be tricky: in order to ensure a relatively homogeneous study population, as for phase I or II research questions (Sect. 1.3.1.1), recruitment may take place over a significant time period and require rejection of numbers of screened individuals (which numbers should ideally be stated in the report). In contrast, recruitment in pragmatic diagnostic test accuracy studies should be straightforward (consecutive patients, few exclusion criteria) although there will be significant heterogeneity in the study population.
Study setting will also impact on recruitment: numbers of potential study candidates will vary according to whether the study is undertaken in the community, primary care, or secondary care specialist clinic, or in a research setting. Recruitment may also sometimes involve a call for volunteers, or by advertisements in the press or media, factors which may potentially introduce patient-based biases into the study cohort (Sect. 1.4.1).
Study design is of critical importance in diagnostic test accuracy studies. Most such studies have a cross-sectional design. In other words, at one point in time, patients undergo evaluation and diagnosis according to a defined reference standard (Sect. 2.2.1), as well as receiving the diagnostic test of interest (ideally administered and/or evaluated by investigators blinded to any other patient-related information: Sect. 2.2.4). This cross-sectional design of course reflects the idiom of clinical practice.
Because the logistics of clinical practice mean that diagnostic test and reference standard are not done simultaneously, diagnostic test accuracy studies should report the interval between them (Sect. 4.2.1).
However, clinicians are aware that sometimes diagnosis is not possible in a single visit or with a single group of investigations. Sometimes, and perhaps particularly with cognitive disorders, especially in their early clinical stages, longitudinal patient assessment is required. Hence it is appropriate for some diagnostic test accuracy studies to have a longitudinal design, sometimes referred to as a delayed verification study design. This is particularly relevant for studies examining the evolution of various types of mild cognitive impairment, for example to Alzheimer’s disease or Parkinson’s disease dementia (e.g. for the use of disease modifying drugs), where the conversion rate to dementia may be low (perhaps 5–10 % per annum: Mitchell and Shiri-Feshki 2009). Duration of follow-up (how long?) is problematic, since some form of censoring is inevitably imposed whenever the dataset is closed. Patient dropout is also a factor requiring consideration in longitudinal studies.
Another important distinction is made between prospective and retrospective study designs, based on the direction of data collection (see Sect. 2.1.4).
2.1.3 Sampling
Sampling depends on many factors, not least the research question which the study is asking (Sect. 1.3.1). However, sampling methods are generally poorly reported in diagnostic test accuracy studies. Reports of dementia diagnostic biomarker studies were found to be missing important information on sample selection methods (Noel-Storr et al. 2013).
In proof-of concept diagnostic test accuracy studies (i.e. those addressing phase I/II questions), “extreme contrast” samples of convenience may be appropriate, sometimes amounting to a case-control design (abnormal vs. normal). For studies involving rare disorders (e.g. prion disease), a case-referent approach may be appropriate (Knottnerus and Muris 2002:44).
Sometimes a random selection of patients is made, set at a predetermined fixed ratio. For example Flicker et al. (1997:205) randomly selected 100 patients seen by aged care assessment teams as a 1:10 sample; of these 100 patients, 78 consented to participate in the study. Hence the final study sample represented only 7.8 % of all patients seen, and hence might possibly be vulnerable to selection bias (Sect. 1.4.1.1).
In pragmatic diagnostic accuracy studies (i.e. those addressing phase III questions), consecutive patients samples (sometimes referred to as “iatrotropic” samples) are used, to better reflect the idiom of clinical practice.
In clinical practice, tests are essentially used to provide arguments for a given diagnosis that is suspected on clinical assessment. Thus, not all patients may be administered a test which seeks to confirm or refute a particular diagnosis, if that diagnosis does not feature in the differential diagnosis. Thus selected patient cohorts may sometimes be appropriate for diagnostic test accuracy studies. For example, only a subgroup of patients attending memory clinics may be suspected of having behavioural variant frontotemporal dementia, and hence might reasonably be tested with cognitive screening instruments which focus on frontal/executive functions (Larner 2013b); or be suspected of having dementia with Lewy bodies (DLB) and hence be tested with screening instruments said to distinguish DLB (Larner 2012d) or subjected to functional imaging with DaTSCANs (McKeith et al. 2007). Examples of such pragmatic diagnostic accuracy studies in patient groups selected on the basis of clinical suspicion (i.e. raised pretest odds) are discussed in Sect. 4.3.
It is also worth noting whether studies are undertaken by the originators of a diagnostic test, or in independent samples. Reviewing instruments used to assess behavioural and psychological symptoms in dementia, van der Linde et al. (2014) found that few had been subjected to independent diagnostic test accuracy studies, most reports originating from the investigators who had first developed the particular test instrument.
2.1.3.1 Sample Size Calculation
Sample size (power) calculations have been recommended for diagnostic accuracy studies (Bachmann et al. 2006). It is recognised that for low prevalence settings or in pragmatic cross-sectional studies of consecutive patients the required sample size will be higher than for high prevalence settings or in proof-of-concept studies because of the lesser contrast between study subjects as compared with the ideal circumstances of phase I or II studies (Sect. 1.3.1.1).
Based on such sample size calculations, Mitchell argued that diagnostic test accuracy studies which recruited fewer than 160 patients (80 per group) had unreliable false negative or false positive results, and hence should be excluded from meta-analyses (Mitchell 2009), a policy which has been followed in some subsequent meta-analyses of diagnostic test accuracy studies of cognitive screening instruments (Mitchell 2013; Larner and Mitchell 2014).
A pragmatic approach to sample size estimates has suggested that normative ranges for sample sizes may be calculated for common research designs, with anything in the range of 25–400 being acceptable (Norman et al. 2012). A review of instruments used to assess behavioural and psychological symptoms in dementia found that study samples were often small (range n = 18–214; van der Linde et al. 2014).
2.1.4 Data Collection (Retrospective vs Prospective); Missing Data
Data collection in diagnostic test accuracy studies may be prospective or retrospective, a distinction based on the direction of data collection. This must be determined when a diagnostic accuracy study is planned.
In retrospective studies, data which has already been collected is accessed. Because disease course and final patient status is often known in these circumstances, this may facilitate a case-control (extreme contrast) approach to test analysis, and results may be generated fairly rapidly if data collection and access procedures are robust. However, there is potential for selection bias (no data available for untested patients, for example because of very mild or very severe disease). Estimates of diagnostic test accuracy are higher with retrospective data collection (Rutjes et al. 2006).
In prospective studies, it is not known in advance which participants do or do not have disease, and hence this approach is typical of practice settings. Because the contrast between cases is less than between known cases and non-cases, larger study numbers are required. Hence prospective data collection is generally more valid but takes longer. Studies asking phase III questions, and hence pragmatic diagnostic test accuracy studies (Sect. 1.3.1.2), must be prospectively planned. A possible drawback with prospective study design is the risk of behavioural influence, or the Hawthorne effect: knowing that a study is occurring, clinician behaviour may potentially change (Larner 2006a). However, this risk should be minimal in pragmatic diagnostic test accuracy studies which are examining tests already incorporated in day-to-day clinical practice, which should not change clinicians’ diagnostic behaviour.
Another, third, approach to data collection, termed “ambispective”, has also been described (Knottnerus and Muris 2002:39). Some use this terminology to denote an approach to study design encompassing two periods, one compiling data retrospectively and one prospectively (“ambispective comparative”; Ramia et al. 2012). Concordance between the results of retrospective and prospective diagnostic accuracy studies of the same test may add to evidence of its validity and diagnostic utility (e.g. Sells and Larner 2011). Perhaps a more appropriate use of the term ambispective is for a study in which subject recruitment is prospective but the data collection can be partly retrospective. For example, some informant questionnaires ask about patient symptoms and behaviour over periods of time before the cross-sectional assessment; for the Informant Questionnaire on Cognitive Decline in the Elderly (IQCODE) this covers the 10 years preceding clinical assessment (Jorm and Jacomb 1989).
To ensure adequate data collection, a protocol proforma may be designed. Nevertheless, loss of data or missing data is an occupational hazard in any diagnostic test accuracy study; for example, in a study of memory clinic patients, a full dataset was available for 299 of 437 consecutive patients, representing only 68 % of the cohort (Flicker et al. 1997:205). Since this loss of data may influence the results, some note needs to be made of this in any report. Reports of dementia diagnostic biomarker studies were found to be missing important information on handling of missing data (Noel-Storr et al. 2013). In pragmatic diagnostic test accuracy studies, data collection should be simplified to fit in with the time pressures of outpatient clinic routines/templates, but as the test under examination may be an integral part of the normal work of the clinic, rather than something new bolted on in addition to normal routine, this should minimise the labour of data collection.
Patients may sometimes not be tested with either the index test or the reference standard (Sect. 2.2.1), or these values may be lost or indeterminate. Missing data may be a reflection of the patient acceptability, or otherwise, of the test being administered. If the test is unacceptable to many patients (e.g. because it is invasive, and/or painful), it may not be generally applicable, likewise if it has any adverse effects (Sect. 4.2.4). More data may be lost as a consequence of dropout in patient self-administered tests than in clinician-administered tests; for example there was a 4.5 % patient dropout rate in a study of the Test Your Memory (TYM) test, a patient self-administered cognitive screening instrument (Hancock and Larner 2011).
In randomised controlled trials, missing data may be imputed by using an “intention to treat” analysis. Similarly in diagnostic accuracy studies, an “intention to test” imputation may be used for missing data (Sect. 3.2).
2.2 Test Methods
Standardization of study protocol is of paramount importance. Any shortcomings of study design (biases: Sect. 1.4) cannot be subsequently ironed out by statistical manipulations.
2.2.1 Target Condition(s) and Reference Standard(s)
Definition of the target condition(s) and of the reference standard(s) is central to diagnostic test accuracy studies.
Target condition(s) may seem obvious. However, clinicians working in memory clinics will be familiar with the question not infrequently posed by patients and their relatives: What is the difference between dementia and Alzheimer’s disease, or are they the same thing? Mutatis mutandis, is the target condition in a diagnostic test accuracy study “dementia” or “Alzheimer’s disease”? Or perhaps any of the many potential causes of dementia (Larner 2013a), or another specific form of dementia, such as “vascular dementia” or “mixed dementia”? Or rarer forms of dementia such as “dementia with Lewy bodies” or “frontotemporal dementia”? Or perhaps any degree of cognitive impairment greater than that expected for patient age, such as “mild cognitive impairment” (MCI), which may (or may not) be conceptualised as the specific prodrome of Alzheimer’s disease (Albert et al. 2011), or of another dementing disorder, e.g. Parkinson’s disease-MCI (Litvan et al. 2012), vascular MCI or vascular cognitive impairment (VCI; Gorelick et al. 2011)? Clearly the investigator(s) must define the target condition at the outset of the study, mindful of changing concepts and terminology (e.g. Dubois et al. 2010), sometimes related to current widely accepted diagnostic criteria.
Reference standard or criterion diagnosis (these terms are preferred to “gold standard” or “standard of truth” since full diagnostic certainty seldom exists, particularly in neurodegenerative diseases; e.g. Hughes et al. 1992) is critical if the discriminatory power of a test is to be measured by comparison. Issues around disease verification by the reference standard are important in diagnostic accuracy studies (De Groot et al. 2011). The reference standard should be applied to all patients included in a diagnostic test accuracy study using a standardized procedure, the latter stipulation to avoid intra- and inter-observer variability, particularly when diagnosis involves subjective interpretation of data. Many tests have a qualitative as well as a quantitative aspect, requiring interpretation by skilled clinician(s), interpretation of which may vary.
Ultimately, reference standard or criterion diagnosis is often based on the judgment of an experienced clinician, or a committee or multidisciplinary team of clinical experts. This judgment may be based on clinical data, with or without information from other routinely used investigations, such as neuroimaging, but not using the result of the diagnostic test under investigation in order to avoid (diagnostic) review bias (Sect. 1.4.2.4; Gifford and Cummings 1999). Clearly this is a “non-perfect” reference standard.
Error in the reference standard is a major limitation on measuring diagnostic test accuracy. With a chronic process such as cognitive impairment, patient follow-up for delayed verification of diagnosis may be incorporated into a diagnostic accuracy study, for example with “progression from mild cognitive impairment to dementia” being used as a reference standard. Since not all cases of mild cognitive impairment progress (Mitchell and Shiri-Feshki 2009), delayed verification is potentially an important factor in diagnostic test accuracy studies, although adding complexity and significantly prolonging study duration. Relatively few diagnostic accuracy studies in dementia have autopsy confirmation of disease as the reference standard (Cure et al. 2014). Though neuropathological confirmation may be regarded as the perfect standard, even here there may be uncertainty because of the pathological overlap of Alzheimer’s disease, vascular dementia, and dementia with Lewy bodies which is sometimes encountered. Certainly pragmatic studies cannot await pathology, with the possible exception of the very rare situations where cognitive decline is sufficiently rapid to mandate brain biopsy (Warren et al. 2005; Wong et al. 2010).
Diagnosis may be based on diagnostic criteria developed by expert consensus; these are available for dementia, mild cognitive impairment, and many dementia subtypes (Box 2.1). Though criteria may be widely accepted, their application may be problematic for a number of reasons. Different diagnostic criteria may significantly influence the calculated prevalence of dementia (Erkinjuntti et al. 1997). The test data should not form part of the diagnostic criteria to avoid incorporation bias (Sect. 1.4.2.3). Different clinicians or multidisciplinary teams may operationalize criteria differently, and some statement about this (“How we do it”) may be required (e.g. Larner 2012e:392). This has been a particular problem for MCI, with a lack of uniformity in the operationalization of this diagnosis in clinical trials (Christa Maree Stephan et al. 2013).
Clinician training and expertise for performance of both index test and reference standard are poorly reported (Noel-Storr et al. (2013)).
Box 2.1: Diagnostic Criteria for Dementia Disorders Sometimes Used in Diagnostic Test Accuracy Studies (Adapted from Larner 2014a:32–3)
Dementia:
Alzheimer’s disease:
Mild cognitive impairment (MCI):
Behavioural variant frontotemporal dementia:
Rascovsky et al. (2011)
Primary progressive aphasias:
Gorno-Tempini et al. (2011)
Frontotemporal dementia with motor neurone disease:
Strong et al. (2009)
Parkinsonian disorders:
Parkinson’s disease MCI:
Litvan et al. (2012)
Progressive supranuclear palsy:
Litvan et al. (1996)
Corticobasal degeneration:
Armstrong et al. (2013)
Corticobasal syndrome:
Mathew et al. (2012)
Vascular dementia, vascular cognitive impairment (VCI):
2.2.2 Technical Specifications and Test Administration
2.2.2.1 Validity and Reliability
Diagnostic and screening tests need to be both valid (i.e. measure what they purport to measure) and reliable (reproducible or repeatable). There are a number of different measures or types of both validity and reliability which are of potential relevance to diagnostic test accuracy studies.
Validity is particularly an issue during the development of new tests. Content validity describes the extent to which a test assesses the domain(s) of interest. For dementia and cognitive impairment, desiderata for screening tests have been suggested (Malloy et al. 1997), namely that sampling of all major cognitive domains, including memory, attention/concentration, executive function, visual-spatial skills, language, and orientation, should be included. These issues should largely have been addressed during test development and are not the major concern of diagnostic test accuracy studies, particularly those of the pragmatic kind.
However, some other forms of validity do impact significantly on the performance of diagnostic test accuracy studies. Concurrent or criterion validity evaluates test agreement with a reference standard (or “gold” standard; Sect. 2.2.1), which forms the basis for the construction of the 2 × 2 table (or confusion matrix; Sect. 3.2) and the calculation of various measures of discrimination (Sect. 3.3), such as sensitivity and specificity, which are used to characterise test performance.
Construct validity evaluates both convergent validity, or how well a new test correlates with tests measuring the same domain of interest, and discriminate validity, or how a new test does not correlate with measures of different constructs (Sect. 3.4.1).
Reliability, the reproducibility or repeatability of a test, is usually considered in terms of inter-rater and intrarater reliability (discussed in Sect. 3.5) and internal consistency. Reports of new diagnostic tests often give details of internal consistency, the extent to which all items reflect the same underlying construct. This may be assessed by calculation of Cronbach’s coefficient alpha, or split-half reliability, or by evaluating or correlating alternate forms of the test. These forms of internal validation need to be supplemented by external validation (Sect. 3.5).
2.2.2.2 Administration; Operationalization; Ceiling and Floor Effects
Test administration refers to how and when measurements are taken. Clearly there may be considerable variation in test administration, which, as has long been recognised (Kelly and Larner 2014), may affect performance. Outcome may be influenced by patient-related factors such as tiredness and/or fatigue, possibly related to a sleep-related disorder, or an affective disorder such as anxiety and/or depression; and testing-related factors such as location (home, primary care surgery, hospital clinic room) and administrator demeanour (stony silence, enthusiastic encouragement). Standardized test operationalization may obviate some of these issues.
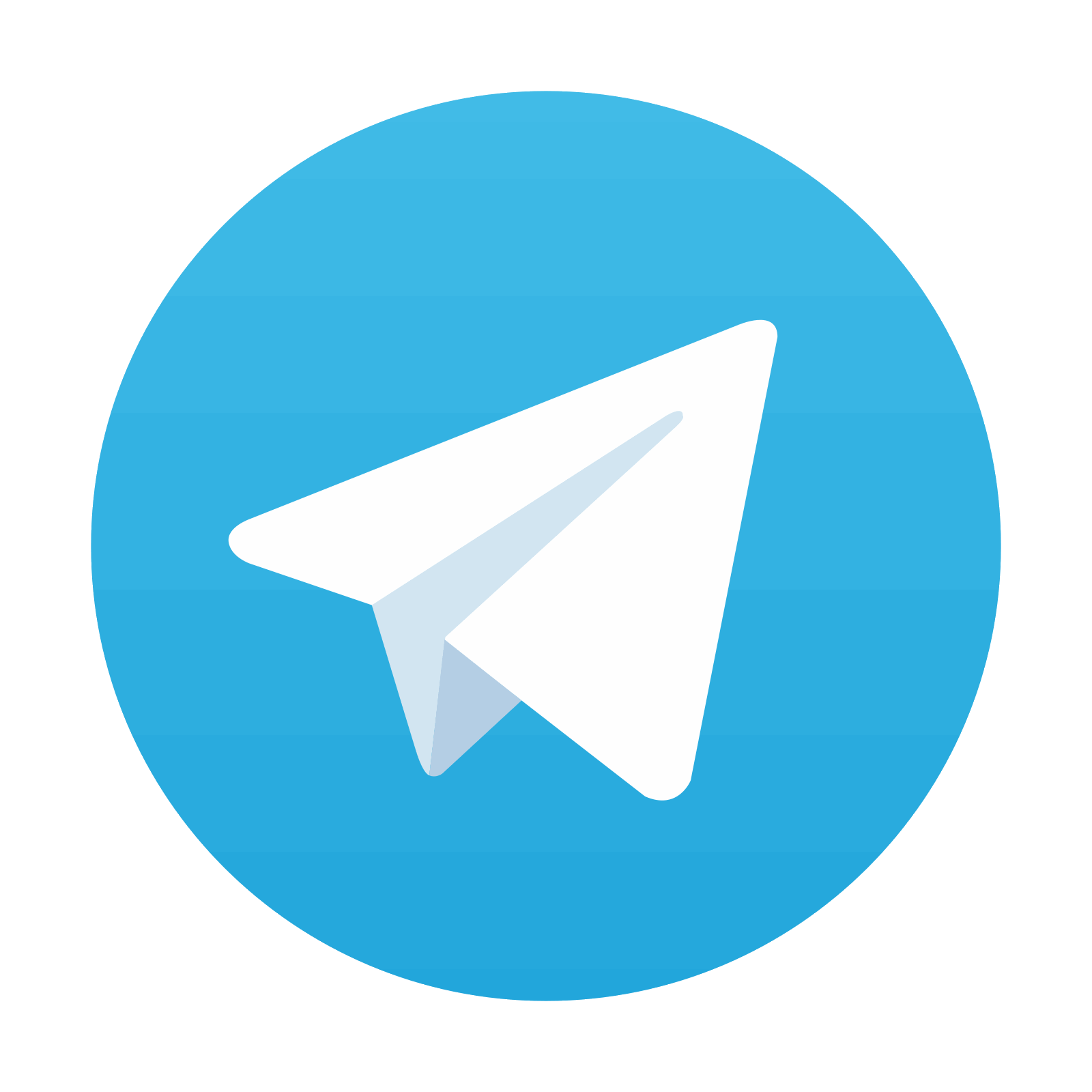
Stay updated, free articles. Join our Telegram channel

Full access? Get Clinical Tree
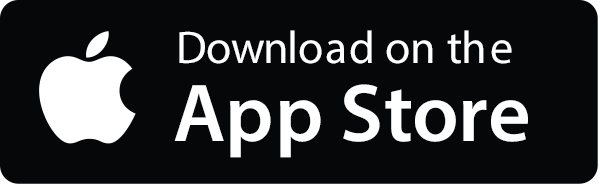

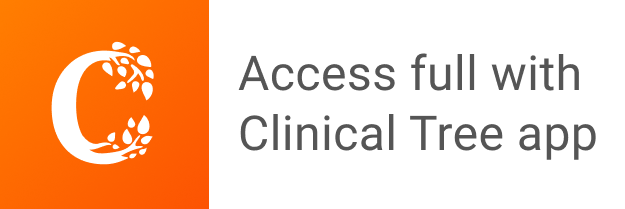