Fig. 9.1
Stages in the drug discovery process. The drug discovery process often begins with the selection of a target using a variety of validation criteria. High-throughput screening (HTS) campaigns are then conducted to identify pharmacological modulators of the target. The output of high-throughput screens are relatively low potency, non-selective molecules called “hits”. Hits are evaluated through a number of secondary assays and further optimized through chemical modifications to identify the most promising chemical matter, or “leads”. Leads are further optimized to improve potency, increase specificity, and achieve the preferred physiochemical and metabolic properties
One way to reduce this risk is to attempt to improve on existing drugs with well-established modes of action. However, to most significantly impact unmet medical need, novel drug targets with novel mechanisms are required. New drug targets are discovered through a variety of means, from serendipitous discoveries in basic biology research to directed target identification screening efforts. Regardless of the source, it is critical to build a strong case that the target is relevant to the pathophysiology of the disease and that intervention would result in therapeutic benefit (Table 9.1). Furthermore, there must be a high likelihood that a successful drug can be developed with the appropriate pharmacological effect on the target. Often the first question is whether expression of the target is altered in the disease or associated with disease pathophysiology. Increased expression of a target in disease, for instance, not only increases confidence that the target contributes to the disease process, but also suggests that inhibition of the target may restore balance to a healthier state without resulting in significant adverse effects. Reduced expression of a target in the disease state, on the other hand, may point towards a need to augment functional activity. Unfortunately, it is particularly challenging to generate target expression data for CNS disorders compared to other disease areas, since human CNS tissue is obviously not as accessible as peripheral tissues where biopsies or blood draws are routine.
Table 9.1
Linking target to disease
Factors that support the role of a putative drug target in disease |
---|
Altered expression in disease |
Component of disease pathophysiology |
Mechanistic link to disease etiology |
Genetic association with disease |
Demonstration of beneficial effects in disease-relevant in vitro model |
Knockout animals display beneficial phenotype |
Pharmacological intervention using tool molecule shows therapeutic benefit in animal model |
Alterations in expression are merely correlative and do not demonstrate any functional consequences. For this reason, demonstration that the target shows a reproducible genetic association with disease often provides more confidence of a mechanistic role. Mechanistic information can also be gleaned by pharmacologically or genetically assessing the function of the target in disease-relevant in vitro or in vivo disease models. As we discuss below, the latter criterion is fraught with many challenges, since multiple factors need to be met in order to ensure that the target function is indeed being accurately probed and that the models are indeed disease-relevant.
There are other more pragmatic considerations for selecting a target, in addition to linking it to the disease (Table 9.2). Notably, the target must be considered chemically tractable, or “druggable”. This is a somewhat amorphous term that is open to broad interpretation. Traditionally, a druggable target would be one in which a small molecule can bind with high affinity and specificity and mediate the desired biological effect. For instance, enzyme inhibition is considered a chemically tractable approach since a small molecule could be envisioned to occupy the active site and antagonize normal activity. Activation of a G-protein coupled receptor would also be considered feasible since a small molecule may be able to alter the conformation of the protein in the same way that the endogenous ligand would. By contrast, blocking a protein-protein interaction has traditionally been considered less druggable; since these are generally large surface interactions mediated by van der Waals force as well as electrostatic interactions, they were thought to be difficult to disrupt with a small molecule. However, scientific advances have changed this view, and modulation of protein-protein interactions by small molecules is now an area of active growth (Higueruelo et al. 2013) . Indeed, the scope of druggable targets continues to expand as new precedents are set, as drug screening technologies become more sophisticated, and as new drug modalities, such as monoclonal antibodies and antisense oligonucleotides, become available.
Table 9.2
Criteria for selecting a new drug target
Target selection criteria | Examples |
---|---|
Link to disease | Genetic association, altered expression, preclinical or clinical validation |
Chemically tractable | Precedents for target class, small molecule endogenous substrate/ligand |
Likelihood for specificity | Some divergence from related family members |
Safety | Restricted expression to target cell/organ, no overt knockout phenotype |
However, it is not merely necessary to be able to generate a potent pharmacological agent against the target; it is also important that the molecule can specifically modulate the target. Understanding the gene family to which the target belongs is useful in predicting the likelihood for specificity. For instance, if the target is highly related to another protein that it is not desirable to inhibit, then it may be difficult to identify specific inhibitors, and the value of the target will be diminished.
The final, and arguably most important, criterion for target selection is safety. Many drugs fail in the clinic, not because of lack of efficacy, but due to adverse safety events. Therefore, it is important to select targets with the greatest likelihood of being safe from the outset, and to address any potential safety concerns experimentally as early as possible. The first question to ask is how broadly the target is expressed. A target expressed specifically in the target cell and tissue presents some advantages, as there is less likelihood for adverse effects unrelated to the desired mechanism of action. Unfortunately, in the case of microglial targets , there are very few genes expressed in microglia that are not expressed in peripheral cells of monocytic origin, so immunosuppression is a persistent concern. This is in contrast to neuronal or astrocytic pathways , where it is often possible to identify targets with expression restricted to the CNS.
The potential safety of a target can also be assessed to some extent if a knockout animal for the target is available. If the knockout animal does not have any observable phenotypes, this is a good sign, with the major caveat that other genes may compensate for the complete loss of function of the target throughout development. A conditional knockout model where the target can be globally ablated only in the adult animal would be more informative than a constitutive knockout. If there are safety concerns, whether due to a knockout phenotype, suspected mechanistic roles, or some other information, it is important to address these directly in an experimental system. For instance, if immunosuppression is a concern, it might be important to challenge animals with an infection after drug administration to determine whether they are able to resolve it. Potential safety issues may not necessarily lead to immediate termination of a program, since there may be a large gap between doses that result in therapeutic efficacy and those that result in toxicity, thereby providing a therapeutic window. However, it is not sufficient to merely hope for the best and determine whether there is a viable therapeutic window in the clinic. The therapeutic window must be diligently determined in preclinical models using all tools available.
9.3 Assay Development
Once a target is identified, the next step is to screen for modulators of the target. A common approach is to employ high-throughput screening (HTS) of small molecule libraries to identify agonists, antagonists or allosteric modulators. In the pharmaceutical industry , an HTS campaign traditionally prosecutes libraries containing 105–106 compounds, and therefore must be run in a high-density plate format, usually 1,536-well plates. More recently, screening has become more sophisticated, employing smaller diverse libraries, fragment libraries, or other emerging technologies, rather than brute force approaches (Manly et al. 2008; Langer et al. 2009; Mayr and Bojanic 2009) . The screens themselves are generally quite rapid. Most of the time and effort goes into developing the assay for the screen, and it is the quality of the assay that often determines the success of the screen.
High-throughput screening assays require more quality control (QC) than assays run in research labs (Table 9.3). This is in part because the assays must be robust enough to perform consistently from day-to-day executed by different scientists, and in part because each compound is run as a single replicate for practical reasons. A common metric used in research for assay performance is the coefficient of variation (CV), the ratio of the standard deviation (σ) to the mean (µ). However, this is not a sufficient metric for an HTS assay, since it does not incorporate the variability at the extremes of the assay and size of the effect. A better metric to QC of an HTS is the Zʹ (Z-prime, also known as Z-factor) (Zhang et al. 1999) . The Zʹ is a measure of the magnitude of the window between the positive (p) and negative (n) controls as well as their variability:
Table 9.3
Assay development quality control
Factor | Measure |
---|---|
Precision of liquid handling | Coefficient of variation of volume transfer |
Reagent stability | Assay performance remains the same over course of screen |
Plate effects | Coefficient of variation of endpoint across plate; discernible patterns |
Assay performance | Zʹ |
Day-to-day consistency | Coefficient of variation of Zʹ over different assay runs |
Assay connectivity | EC50 or IC50 values comparable across multiple assays |

(9.1)
The factor of 3 is chosen to multiply the sums of the standard deviations because in a normal distribution, 99 % of values occur within 3 standard deviations of the mean. An assay with no variability (σ = 0) will have a Zʹ of 1, and therefore 1 is the highest Zʹ possible. However, due to the factor of 3 in the numerator, Zʹs are very sensitive to assay variability. Indeed, in an assay with three replicates, a Zʹ can be negative and still show highly significant differences between positive and negative controls, whereas a cutoff of 0.5 is generally required for HTS. This highlights the increased rigor required for screening compared to assays run in a more traditional basic research setting.
Developing in vitro assays in microglial cultures poses additional unique challenges. Microglia are specifically adapted to respond dramatically to the slightest signal of damage or infection. Therefore, even tiny amounts of contaminants, such as endotoxin present on most labware, can have major consequences on assay performance, even though they would not cause any noticeable effects on most other cell types. Standard protocols must be adapted so that special care is taken to minimize the effects of environment on microglia (Witting and Möller 2011) .
There are additional considerations when establishing a screening assay. If automation is used, which is usually the case, the precision of liquid handling needs to be assessed. This can be achieved by transferring a dye, such as tartrazine (Petersen and Nguyen 2005) , at the desired volume to several plates and measuring the optical density against a standard curve. The assay endpoint should also be assessed across several plates to determine whether there are any artifacts amongst wells of a plate or from plate-to-plate. Since automation often adds time delays and assays are run over several hours, the stability of the assay components and of the assay itself needs to be determined. Finally, the assay must perform robustly from day-to-day over the course of the entire screen. Each run should include the appropriate controls, such as a whole column of the positive control and a whole column of the negative control, to ensure that the assay continues to perform with the appropriate metrics.
9.4 Hit-to-Lead and Lead Optimization
The output of screening campaigns using compound libraries are generally poor pharmacological agents, called “hits” or “actives”, that serve as mere starting points in the drug discovery process. Hits must go through a number of quality assurance steps, secondary assays and some chemical optimization before promising lead compounds are identified (Fig. 9.2).
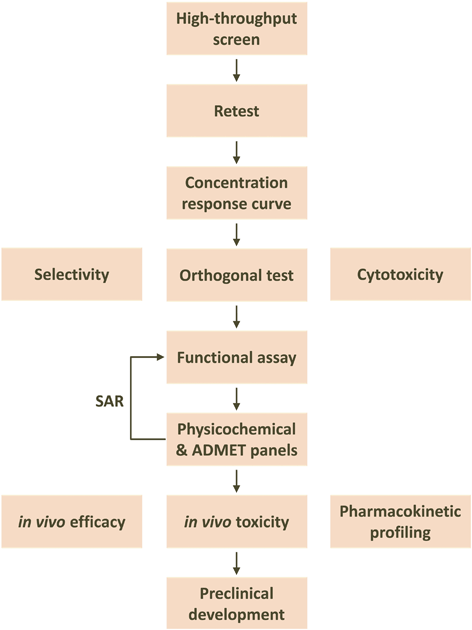
Fig. 9.2
Generic drug discovery screening cascade. After hits are identified from a high-throughput screen, they are first confirmed through retesting at the screening concentration. Confirmed hits are then tested over a range of concentrations to generate concentration response curves and determine potency. Orthogonal tests confirm hits using technology completely independent of the screening assay to rule out potential screening artifacts. Orthogonal assays are usually more physiological than the HTS assay. At this point, compounds may also be evaluated for selectivity against proteins related to the target and, if they are going to be taken from a cell-free to cell-based assay, for general cytotoxicity. Secondary assays are then run to confirm the functional consequences of modulating the target. Compounds are then evaluated through a variety of in vitro physicochemical and ADMET (absorption, distribution, metabolism, excretion, toxicity) assay panels used to predict pharmacokinetic (PK) properties and potential safety liabilities. Structure-activity relationships (SAR) are determined via reiterative medicinal chemistry to optimize PK, safety and activity, producing lead compounds. Lead compounds are tested in vivo to determine their PK, safety and efficacy profiles. The best compounds are progressed towards full preclinical development to identify candidates that will enter the clinic. It should be noted that this is a generic example. In keeping with the spirit and scope of this work, greater details are given for the early part of this process, and much less so for the later one. (For details on the later part of the preclinical drug development process, the reader is suggested to access a number of references (Wermuth 2008))
The first step is to retest a relatively large number of actives to confirm the biological activity found in the N = 1 screen. At this stage, some compounds will replicate the finding, and some will not. The former are considered “confirmed actives”. Often a freshly-prepared sample is then tested to further confirm the in vitro activity. A number of counterscreens are performed to eliminate the possibility of false positives, such as tests on a parental cell line not expressing the receptor being studied, as well as a number of orthogonal tests which are usually run under more physiologically-relevant conditions than the screening assay.
This exercise often delivers up to a few hundred compounds ready to move forward. Sometimes an early readout of possible structure-activity relationships can be established. This is extremely valuable at this early stage, as it supports chemical tractability of the biological activity, which should not be taken for granted. Compounds are then typically analyzed based on a number of in silico physicochemical descriptors, which are used as surrogates of the drug-like quality of the compounds being studied. The most common descriptors used include molecular weight (MW), lipophilicity (cLogP), polar surface area (PSA), rotatable bonds (RB), and hydrogen bond donors and acceptors (HBD and HBA, respectively). For the specific case of CNS drugs a drug likeness central nervous system multiparameter optimization (CNS MPO) algorithm may be used (Wager et al. 2010) .
After all these efforts, generally a number of distinct chemical series, called leads, are prioritized and the lead optimization work starts, aiming at delivering highly optimized compounds known as drug candidates. The optimization process navigates through a number of hurdles to maximize everyone’s confidence that the compound will be efficacious and safe when taken to the expensive clinical trials . For most projects this implies assuring oral bioavailability, good absorption and pharmacokinetics, efficacy at a reasonable daily dose, lack of drug-drug interactions derived from cytochrome P-450 (CYP) inhibition or induction, minimizing interactions with active transporters (e.g., P-glycoprotein for CNS drugs), establishing an effect-exposure relationship in vivo, efficacy in phenotypic and mechanistic pre-clinical models (hopefully with good translational validity, vide infra), as well as a large battery of selectivity and safety tests in vitro and in vivo, to determine a therapeutic index and a maximum tolerable exposure during the clinical work (van de Waterbeemd 2009) .
It is important to understand that, for the vast majority of new drug projects, the development candidate is not a compound that already exists as a member of a chemical library. On the contrary, medicinal chemistry efforts are often described as “threading the needle”, to symbolize the highly sophisticated process that generally results in a very small number of molecular entities with acceptable attributes. Another point worth discussing is that in vitro potency should not be used in isolation as the key driver to rank-order compounds for further profiling. Indeed, it has been concluded that marketed oral drugs seldom possess single-digit nanomolar potency (50 nM is the average potency) (Gleeson et al. 2011) . Therefore, a development candidate is often a compound with a number of balanced attributes rather than the molecule that performs significantly better in every possible test in the screening cascade.
9.5 Biological Validity Criteria: Predictive, Face and Construct Validities
A critical step in validating targets and evaluating lead molecules is to test them in preclinical animal models. A major pitfall has been the indiscriminate use of preclinical animal models without sufficient understanding of their validity. This paradigm worked for a while, when the biological target space exploited was a continuation of past successes. However, once these “low hanging fruits” of drug discovery were eventually depleted, and to continue to address unmet patient needs, industry had to venture into newer, more complex areas and hence, explore more sophisticated animal models (Meier et al. 2013) .
The risk of failure is especially high for compounds reaching late clinical trials , where hundreds of millions of dollars may be at stake in just one clinical study. In order to manage the risk involved in clinical translation of efficacy from preclinical models, a number of concepts were developed under the umbrella of the validity. While the concept is not new, (Willner 1984) it is now a subject of increased focus (Fineberg et al. 2011) .
There are three primary domains of validity that are sought after in animal models of disease: predictive, face and construct validity (Willner 1984; Markou et al. 2008; Becker and Greig 2010; Dzirasa and Covington 2012) . Predictive validity refers of the ability of the model to predict pharmacological efficacy in the clinic. For instance, the Forced Swim Test (Porsolt et al. 1977) in which rodents are placed in a beaker of water has some predictive validity for depression because monaminergic-based antipressants reduce animal immobility in this assay (although the observed rapid onset of efficacy in this model does not reflect the clinical experience). The major limitation for models with predictive validity is that they may not respond to drugs with novel, and maybe more efficacious, mechanisms of action. Furthermore, if there are no effective drugs in the clinic for a given disease, it would not be possible to know whether the model possesses any predictive validity for that disease.
Models with face validity overtly resemble the symptoms of the disease. For example, models in which dopamine neurons are ablated using exogenous toxins, such as 1-methyl-4-phenyl-1,2,3,6-tetrahydropyridine (MPTP) (William Langston et al. 1984) , exhibit some of the same motor symptoms as Parkinson’s disease . The caveat for preclinical models with face validity is that they may not reflect the etiology of the disease in humans, and therefore may not respond to therapeutic interventions that target disease pathogenesis. They may however respond to treatments aimed at the symptoms of the disease.
The third type of biological validity is construct validity . Construct validity means that the model captures the underlying pathophysiology of the disease. This could be at the molecular level, such as when a transgenic animal model is generated by overexpressing a human mutant protein that causes an inherited monogenic disorder; or at the systems levels, such as by altering neuronal circuitry in a way that mimics alterations found in disease. The phenotypic manifestation of a model with construct validity may not resemble the disease at all. For instance, Drosophila mutants with loss of the parkin gene are arguably a model for early-onset Parkinson’s disease with construct validity, yet the phenotype manifests as difficulty emerging from their pupal cases and defects in flight due to degeneration of muscle cells (Greene et al. 2003; Whitworth et al. 2005) .
Ideally, a disease model will have all three types of biological validity. This is rarely, if ever, the case. Nevertheless, models with only one or two types of biological validity can be very useful in the drug discovery process, so long as their limitations are clearly understood and they are used to answer the right questions.
9.6 Additional Validity Criteria: Chemistry, Quantitative Pharmacology and Bias-free
In addition to the well-described types of biological validity, we propose three additional types of validity to consider when conducting preclinical studies using pharmacological probes in animal models. These are chemistry, quantitative -pharmacology and bias-free validity (Fig. 9.3).
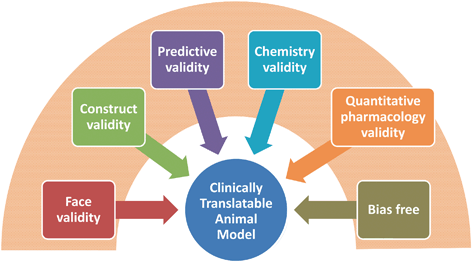
Fig. 9.3
Schematic proposal for enhancing biology-based validity criteria to include chemistry, quantitative pharmacology and decision-making principles useful in supporting scientific research aiming at validating biological targets for drug discovery projects
9.6.1 Chemistry Validity
The primary intent of a chemical probe is to establish the relationship between a molecular target and the broader biological consequences of modulating that target (Frye 2010) . To this aim, a certain level of qualification of the compound being used across a number of areas is required. The goal of this exercise is to make sure the compound is actually doing what one thinks it must do, the way one expects it should do it, by ruling out other potential interferences of chemical origin.
Answering a number of key questions will go a long distance to support phenotypic observations and link these to a true pharmacological modulation of a biological target by a tool compound or drug candidate. For example,
Is there a class effect? Do different compounds, with diverse chemical structures but similar target profiles, demonstrate similar pharmacology? Or is this a “one-off” effect, only seen with one compound and not seen with very close analogs?
Does the tool compound have any druggability flaws derived from its chemical structure?
A number of properties related to the chemical structure of a certain tool compound may cloud the interpretation of phenotypic screens. Among these, the most common are shown in Table 9.4 (Davis and Erlanson 2013) .
Table 9.4
Some fundamental factors to be considered for available probe compounds depending on the nature of the study being planned
In silico | In vitro | In vivo, single-dose | In vivo, multiple-dose |
---|---|---|---|
Unequivocal structure | Functional activity and binding affinity at the target | Dosing routes: IV, IP, PO, SC, ICV | Dosing routes: IV, IP, PO, SC, minipump, ICV |
Chemically synthesizable | Solubility in buffer used | Unbound drug concentration commensurate with in vitro activity | Understand exposure time profile and unbound drug concentration commensurate with in vitro activity at peak and trough |
Selectivity (binding and functional screens) against anti-targets and broad panel | Selectivity | Selectivity | |
Possible active metabolites | Possible active metabolites | ||
CYP induction |
It is important to understand that these are not rigid rules, and that are meant only to exemplify some of the weaknesses that may be encountered that bias data interpretation. For example, not all compounds containing a certain chemical moiety will be pan-assay interference compounds (PAINS) (Baell and Holloway 2010) ; it varies on a case-by-case basis and should be considered only as a risk management strategy.
9.6.2 What Type of Study Are You Planning?
Not all of a compound’s properties are equivalent or critical in terms of qualifying it as an appropriate tool compound for the planned study. So, which molecular attributes are truly needed and how to define them? A useful way to address this issue is based on the type of pharmacological study to be conducted: in silico, in vitro , acute in vivo or multiple-dose (sub-chronic) in vivo.
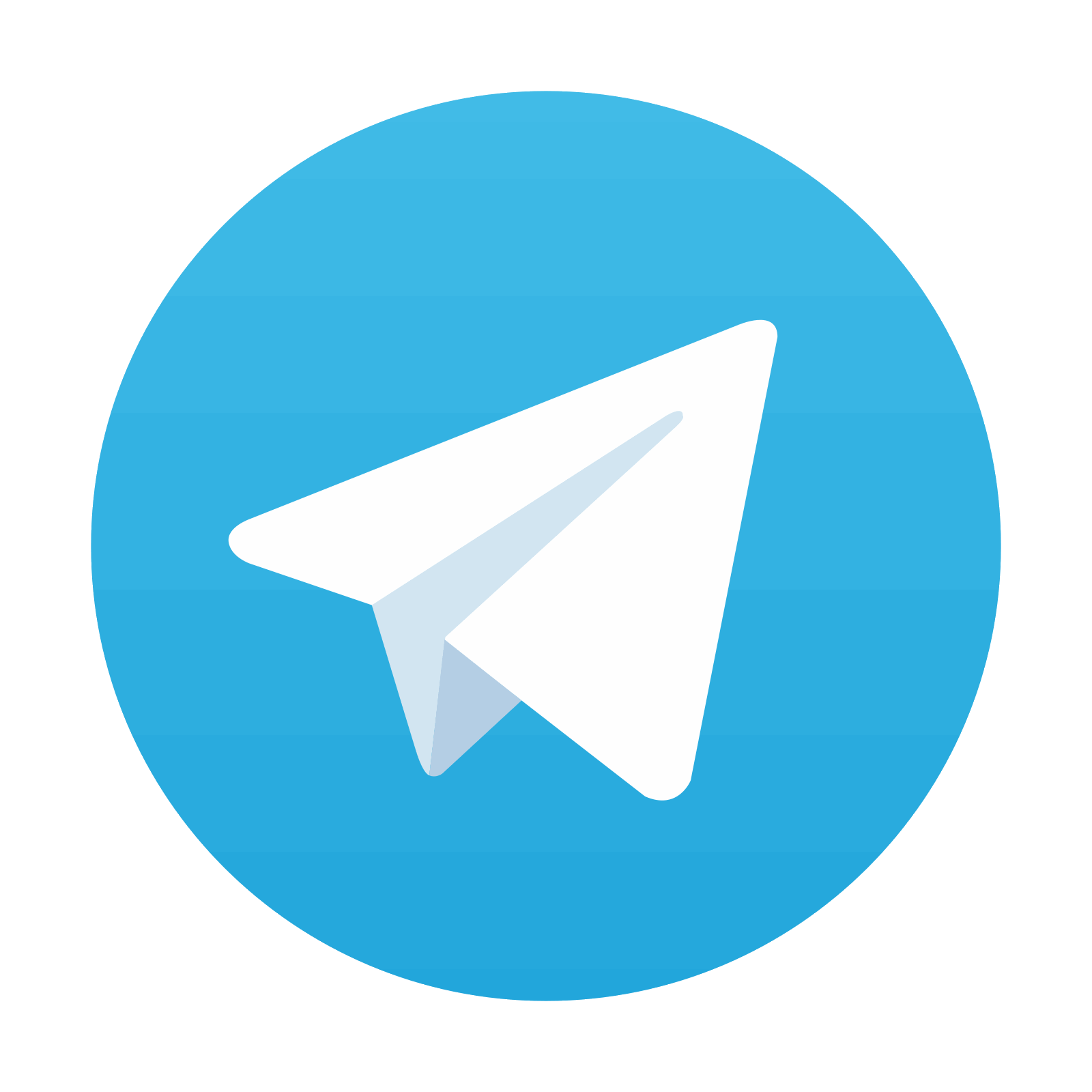
Stay updated, free articles. Join our Telegram channel

Full access? Get Clinical Tree
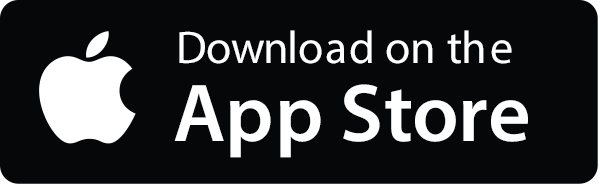
