(1)
for 1 ≤ i ≤ N, where v i denotes the membrane voltage of neuron i, μ is a dc component in the noisy synaptic input and τ is the membrane time constant for the subthreshold dynamics. We adopt the standard threshold-spike-reset condition [8]: a spike is emitted whenever v i (t) = V T , and after that the voltage is reset and held at v i (t +) = V R during a refractory period τ R . Additionally, s(t) is a common component in the input and the Gaussian white noise η i (t) (1 ≤ i, j ≤ N) models the internal stochastic component for the ith neuron. For each neuron, the output spike train y i (t) = ∑ k s(t − t i k ) with t i k being the kth spike time is of interest, and the average spike train
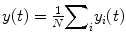
![$$ \left\langle {\eta}_i(t){\eta}_j\left(t+\tau \right)\right\rangle =\left[{\delta}_{i,j}+c{\delta}_{i,i+1}\right]\delta \left(\tau \right) $$](/wp-content/uploads/2016/09/A315578_1_En_30_Chapter_Equ2.gif)
(2)
Since the local spatial correlation does not affect the response of each neuron, it is necessary to obtain the spectral statistics
for the ensemble output spike train, where
is the Fourier transform of the zero average output spike train with r 0(D) being the stationary firing rate at the noise level D. For this aim, let us resort to technique of linear approximation [9–11]. According to linear approximation, each neuron can be regarded as a linear filter, and thus the frequency domain linear response for each neuron can be approximated as

where
is the unperturbed part of stationary spectral density
and A(ω, D) is linear susceptibility on the noise level of D. The stationary spectral density [12] and the linear susceptibility [13, 14] are explicitly given as


with
. And then from Eq. (3), the auto-spectral density
for the ith neuron is obtained as

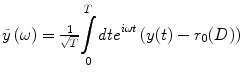

(3)

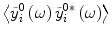

(4)

(5)
![$$ \gamma =\left[{v}_R^2-{v}_T^2+2\overline{\mu}\left({v}_T-{v}_R\right)\right]/4D $$](/wp-content/uploads/2016/09/A315578_1_En_30_Chapter_IEq6.gif)
