Fig. 3.1
Cost of sequencing a single human genome. Sequencing costs have fallen rapidly over the past five years as next-generation technologies become increasingly efficient. Graph is reproduced using data from the National Human Genome Research Institute [11]
Before looking in depth at how NGS technologies have improved our understanding of the complexity of cancer and made personalized treatments possible, we will review commonly used NGS techniques.
Whole-Genome Sequencing (WGS) and Whole-Exome Sequencing (WES)
The emergence of precision medicine is a direct result of technical advances in genomic sequencing platforms. While the human genome was largely assembled using automated Sanger technology (commonly referred to as “first generation”) [12], this approach was incapable of scaling to provide personalized data in routine clinical practice. The emergence of next-generation approaches has drastically reduced the cost and complexity of genomic analysis, such that enormous amounts of data can now be acquired in a relatively short period of time. Due to the availability of several excellent review articles on this topic [13–15], we will give only a brief overview of the technical steps involved in exome and genome sequencing.
NGS technologies rely on massive parallelization of sequencing tasks, allowing for rapid collection of data that scales with increasing sample numbers. The process begins with library preparation, in which a patient sample is fragmented into smaller genomic regions that can be sequenced continuously. The optimal size of fragments varies based on sequencing platform (i.e., Illumina, Pacific Biosciences, and Solexa); however, longer reads are generally preferred to reduce ambiguities in bioinformatics analysis, such as alignment. Platform-specific, universal adaptors are ligated to the ends of each fragment that permit hybridization to a flow cell, a substrate upon which sequencing will take place. These adaptors also allow for amplification to create a localized colony of each fragment, which increase the signal during the sequencing stage. With the flow cell prepared, sequencing commences with the stepwise addition of labeled nucleotides to each colony. After addition of each base, an image snapshot of the flow cell is collected that will be assembled chronologically to infer the fragment’s sequence. Because many colonies are imaged simultaneously, sequencing is performed in parallel and scale is only limited by the surface area and colony density of the flow cell. With the sequences collected, bioinformatics approaches are then used to align each read to its corresponding genomic region (further discussed below).
In exome sequencing, only the protein-coding region of the genome is sequenced (less than 2%), significantly reducing the expense of data acquisition and complexity of analysis [16]. The vast majority of human disease is driven by mutations in these regions, making this approach an efficient method for the detection of most pathogenic variants. From a technical standpoint, the biggest difference from whole-genome sequencing occurs during library preparation, in which an additional capture step is performed. This most often occurs through hybridization of adaptor-ligated fragments to biotinylated DNA baits, followed by selective pull-down, amplification, and sequencing [17]. Several commercially available kits provide bait coverage of the entire coding genome or selective regions, depending on the application.
Bioinformatic Analysis of NGS
Computational components of genomic data can be grouped into three stages: primary, secondary, and tertiary analyses [18] (Fig. 3.2). Primary analysis includes the conversion of raw image data captured from the flow cell in sequencing machines into human-readable representations of the input (i.e., sequences of nucleotides). A secondary analysis then compares this sequence to the reference genome, which was made available with the completion of the Human Genome Project. This step includes alignment of read sequences to the reference genome and identification of variations between the datasets, which represent possible disease-causing mutations. The alignment step tries to identify the genomic origin of each sequencing read; however, ambiguous alignments can sometimes occur in regions containing similar DNA sequences. Technologies that produce longer sequencing reads help to solve this problem by reducing ambiguity. Following the alignment step, variant calling identifies regions in the aligned read that differ from the reference genome, called mismatches. However, stochastic machine errors introduced during sequencing and image capture can introduce noise, which complicates this step. These kinds of errors are handled computationally by the use of redundancy in data, achieved by oversampling of the DNA [8]. Over the years, many programs have been developed for various components of the secondary analysis step, including quality control, alignment/assembly, and variant calling. Some of these tools are for specific technologies, such as CASAVA and ELAND for Illumina sequencing machines and Newbler/GS Reference Mapper for Roche/454 machines. However, it was the open access tools, providing standard and streamlined analysis of genomic and transcriptomic data, which paved the way for collaborative and large-scale studies such as TCGA and ICGC. Some of these tools include BWA [19], MAQ [20], and Bowtie [21] for short DNA sequence alignment, as well as toolsets such as SAMtools [22] and GATK [23] which create a streamlined analysis pipeline platform.


Fig. 3.2
Lifecycle of genomic analysis guided personalized medicine. Genomic or transcriptomic material extracted from each patient’s tumor is sequenced in NGS machines to produce raw sequence data. Bioinformatatic algorithms are used to align the raw data to the reference genome, and variations in each tumor are identified. Lastly, candidate alterations that may cause tumorogenesis are identified as targets and appropriate treatment regimens selected
In tertiary analysis, variations identified in the secondary analysis step are analyzed individually to assess the biological impact and ultimately decide whether an alteration is disease-causing, specifically a driver event for cancer cases. Whether it is genomic or transcriptomic data, identification of a driver event is computationally and biologically a challenging step due to the large plethora of variations that are called in most studies. To overcome this challenge, information from various sources is aggregated to improve understanding of the impact of the alteration on disease biology. These annotation steps include evaluating the functionality of the genomic region in which the alteration occurs, by leveraging data from projects such as the Encyclopedia of DNA Elements project (ENCODE) [24] and the encyclopedia of genes and gene variants project (GENCODE) [25]. Additional annotations use computational prediction algorithms to assess the impact of an alteration on the DNA residue based on 3D structure of the protein, or the conservation of the amino acid residue where the alteration occurs [26, 27]. In addition to the structural and functional annotation schemes, the occurrence frequency of the alteration in healthy populations such as 1000 Genomes [28] and disease cohorts such as COSMIC [29] is also used to separate the disease-causing alterations from passenger alterations or noise. Once a set of candidate variations are identified by downstream bioinformatics analysis, the next step is the biological validation of the candidates and clinical interpretation that will ultimately lead the way to a precise treatment of the individual case.
Gene Expression Studies
Gene expression studies have provided insight on the molecular mechanisms underlying malignancy and aided in subclassification of seemingly homogeneous tumors into clinically distinct entities [30]. In this approach, messenger RNA (mRNA) is extracted and purified from a tumor sample and undergoes transcript quantification using either expression microarray or RNA-sequencing (Fig. 3.3). In the former case, mRNA is washed over a substrate covered with complementary probes, with each probe emitting florescence that is proportional to the amount of RNA hybridized to it. The abundance of each transcript can then be calculated by measuring the signal of each probe [31]. In the case of RNA-sequencing, purified mRNA undergoes reverse transcription to produce a complementary DNA (cDNA) library. This library undergoes NGS, and the amount of reads that align to each gene is used to estimate the transcript’s abundance. In both approaches, careful normalization must be performed to ensure that accurate comparisons can be made between samples. This is often performed with respect to the overall number of RNA-sequencing reads, as well as the size of each gene (i.e., larger genes are expected to have higher number of reads mapping to them).


Fig. 3.3
Gene expression analysis pipeline. Gene expression analysis is most often performed using either expression microarray, or RNA-sequencing. Each technique offers benefits depending on the application and project goals
Proteomics
An emerging approach in precision medicine is the application of insights from systems biology to an individual’s disease [32]. DNA-sequencing and gene expression studies give an indirect view of cellular activity, since they measure activity upstream of the molecular effectors. By providing a complete picture of the functional status within a cell, proteomics has become an important tool in personalized treatment. While technological approaches in protein quantification lag behind equivalent tools in RNA and DNA measurement, recent progress has been promising. The most widely used methods are based on mass spectrometry, in which ionized protein fragments are detected according to their mass and charge. In many cases, an additional selection technique such as chromatography is used to limit the spectrum of proteins that undergo detection. While assessment of the complete proteome remains a technical challenge, measuring the status and abundance of individual biomarkers can be a valuable tool for clinicians. One such example is prostate-specific antigen (PSA), which can be differentially detected in patients with prostate cancer using proteomic techniques [33]. The packaging of multiple biomarkers can also be used to construct an oncogenic signature that is detectable using patient serum. A previous study used this technique in combination with machine learning to achieve a positive predictive value of 94% for the detection of ovarian cancer [34].
Genomic Approaches to Personalized Medicine
Precision or personalized medicine, a concept that existed long before the emergence of NGS technologies, is defined as the prevention and treatment strategies developed based on an individual’s biological and physiological variabilities [35]. With the application of NGS technologies, genomic variations that underlie disease mechanisms have been better characterized, leading the way to improved targeted treatments. Specifically for cancer, personalized treatment is the precise planning of treatment regimens based on the molecular, genomic, and transcriptomic profile of the individual tumor in addition to the pathological and physiological features.
Before the emergence of personalized medicine using NGS technologies, the first targeted cancer treatment developed for a specific genetic alteration was for chronic myelogenous leukemia (CML). Development of the drug imatinib, which targets the tyrosine kinase fusion protein BCR-ABL, has increased the 5-year survival for CML to 89% of patients [36]. This result ushered in a period of expectant optimism regarding the promise of targeted therapies, with many hoping that cancer might some day be eliminated using precision approaches. Unfortunately, most malignant cancer types, such as gliomas, have been found to be genomically heterogeneous, making treatments that target a single genetic alteration obsolete [37] (Fig. 3.4). The emergence of cost-effective genomic and transcriptomic profiling of tumors through NGS technologies helped to identify this complexity within most malignant cancer types, particularly in malignant tumors such as gliomas [38–40], medulloblastomas [41], and neuroblastomas [42] and also in more benign types such as meningiomas [43]. While these findings have diminished hope for a “silver bullet” to selectively melt away tumors, they have laid the groundwork for personalized combination targeted therapies that will increase survival.


Fig. 3.4
Types of tumor heterogeneity. The presence of several forms of tumor heterogeneity makes treatment of malignant brain tumors a challenge. Schematics of inter-tumor heterogeneity, intra-tumor heterogeneity, and temporal heterogeneity are shown
Insights on Malignant Brain Tumors from Genomic Studies
As genomic tools have become increasingly accessible for clinical use, attention has shifted to developing protocols for interpretation of patient results. Until recently, the genomic landscape of many cancers was poorly described, leaving the significance of variants found in a clinical dataset unclear. However, global efforts over the past decade have elucidated the oncogenic drivers underlying most tumor types, including genetic mutations, changes in gene expression, chromatin accessibility, and other molecular features. These efforts have largely occurred through national or international consortiums that sequence hundreds of tumors from large patient populations. By comparing the genome of each tumor to a matching non-tumor sample from the same patient, researchers can identify genetic changes that may drive oncogenesis. The variant databases that are generated from these studies provide an invaluable resource for clinicians, allowing direct annotation of patient results with aggregated data from across the spectrum of cancer, such as COSMIC [4]. In addition to confirming the presence of known oncogenic variants, prognostic and therapeutic insights can also be gleaned by studying the clinical course of patients harboring similar mutations in previous studies. The availability of large-cohort genomic studies has provided critical context necessary to carry out personalized medicine.
This section will briefly review several genomic studies that have made seminal contributions to understanding the molecular drivers of brain tumors. The discussion is not intended to be an exhaustive list of identified mutations for each tumor type, but instead will focus on a small number of pathways identified by researchers that hold clinical promise. For almost all tumor types, excellent review articles are available that extensively describe the landscape of genomic findings (Table 3.1).
Table 3.1
Common driver events associated with malignant brain tumors
Tumor type | Cell of origin | Driver events |
---|---|---|
Ependymoma | Radial glial cells [103] | Spinal: Chr7 amplification, Chr22 deletion, NF2 mutation [104], Intracranial: Chr1q amplification [105], genomic imbalance [106], CDKN2A deletion, C11orf95-RELA fusion [107] Epigenomic alterations with CIMP-positive [108] |
Schwannoma | Schwann cells | Bi-allelic NF2 loss with Chr22 deletion and NF2 mutation [109] |
Pituitary adenoma | Lactotroph Somatotroph Corticotroph Gonadotroph | Prolactionoma: Deletion of Chr 11p [110] |
Meningioma | Arachnoid cap cells | |
Medulloblastoma | Cerebellar lineage | |
Glioblastoma | Glial lineage |
Glioblastoma Multiforme
Perhaps the best studied of malignant brain tumors is glioblastoma (GBM), which was selected by TCGA as the first type of cancer to undergo extensive genomic characterization [44]. While previous studies had uncovered recurrent genetic events in these tumors, their approaches were largely hypothesis-driven and therefore did not investigate the full landscape of GBM using unbiased methods. The TCGA study was notable for establishing a systematic framework for the characterization of tumors, including standardization of biospecimen collection and integration of data modalities to draw actionable conclusions. They reported on alterations in DNA copy number, gene expression, DNA methylation, and somatic variants in a total of 206 GBM samples. In addition to confirming several suspected pathways underlying GBM, they also identified an association between promoter methylation of the DNA-repair gene O-6-Methylguanine–DNA Methyltransferase (MGMT) and hypermutation in treated samples. Methylation of this gene was previously correlated with response to the alkylating agent temozolomide [45], and subsequent studies have established this event as an important biomarker for prognosis and therapeutic course [46].
Collectively, genomic studies of this tumor have led identification of numerous molecular targets as well as classification schemes. Based on gene expression studies, GBM can be divided into proneural, neural, classical, and mesenchymal subtypes, each with distinct mutational profiles [47, 48]. Genomic evidence, including specific marker expression patterns, has suggested that these subtypes may arise from distinct cellular origins, although a common neural stem cell hypothesis has also been proposed [49]. The classification of a patient’s tumor may guide treatment decisions, as classical tumors tend to respond to aggressive therapy, while proneural tumors are often refractory. Altered expression and genomic events involving the epidermal growth factor receptor (EGFR) are associated with the classical subtype and are seen in a majority of cases. The most common variant in this gene involves deletion of exons 2–7 (termed “EGFRvIII”), which is a negative prognostic indicator in patients surviving greater than one year [50]. In addition to EGFR amplification, deletion of p16INK4a and mutations in the tumor suppressor phosphatase and tensin homolog (PTEN) are other common events seen in primary GBM. By contrast, secondary GBMs are associated with mutations in the gene isocitrate dehydrogenase 1 (IDH1), with frequent comutation of alpha-thalassemia/mental retardation syndrome, X–linked (ATRX) or tumor protein P53 (TP53) and deletion of cyclin–dependent kinase inhibitor 2A (CDKN2A), and PTEN, or retinoblastoma 1 (RB1) [51].
In addition to GBM, other malignant gliomas such as anaplastic astrocytoma and oligodendroglioma have also been genomically characterized. IDH1 mutations are common in these grade III tumors, which is consistent with their high prevalence in secondary GBMs. The malignant progression of oligodendroglioma, which initially harbors losses on chromosomes 1p and 19q as well as mutations in the growth regulators capicua transcriptional repressor (CIC) and far upstream element binding protein 1 (FUBP1), may be propelled by the loss of CDKN2A and PTEN [52, 53]. As discussed above, malignant astrocytoma (including grade III lesions) is more likely to be driven by mutations in ATRX and TP53, in addition to Rb pathway mutations.
Medulloblastoma
Like glioma, medulloblastoma has undergone extensive genomic and transcriptomic characterization [54, 55]. These studies have led to the classification of medulloblastoma into four molecular subgroups, including WNT, SHH, Group 3, and Group 4. While the WNT and SHH subgroups harbor overactivity of their associated pathways, Group 3 and Group 4 tumors are characterized by MYC and CDK6 amplification, respectively. Importantly, each of these groups carries specific clinical and demographic features as well as implications for prognosis and therapeutic options. For example, WNT medulloblastomas are more commonly found in older children and are almost always of the classic histological subtype [56]. By contrast, SHH tumors often present during infancy and are associated with the desmoplastic subtype (although all histologies are possible in this group). Until recently, the major drivers of Group 3 and Group 4 subgroup medulloblastomas were mostly unknown, as there were only a few recurrent somatic mutations identified. This changed with the publication of a large whole-genome sequencing project that identified recurrent structural variations in 33% of Group 3 and 5–10% of Group 4 medulloblastomas, leading to the juxtaposition of the growth factor independent-1 family proto-oncogenes, GFI1 and GFI1B, with upstream cis-acting regulatory elements such as super-enhancers [57]. Such genomic structural alterations, defined as the super-enhancer hijacking, lead to overexpression of the proto-oncogenes, GFI1 and GFI1B, and were shown to be oncogenic in mouse models in the same study [57].
Importantly, medulloblastoma subgroups also carry prognostic implications, with Group 3 medulloblastomas having a particularly poor prognosis, while WNT tumors are relatively favorable [58]. Interestingly, genomic approaches have suggested that these subgroups may arise from different cells of origin in the cerebellum, perhaps explaining the differences in features and presentations [59, 60].
The identification of medulloblastoma subgroups has opened novel treatment approaches that target specific pathways. The most notable of these is the use of antagonists that block activation of the SHH pathway. Previous administration of the SMO inhibitor GDC-0449 in an adult patient with metastatic disease caused a rapid regression of the tumor; however, resistant clones soon arose and the tumor returned [61]. Clinical trials are ongoing for pharmaceuticals that block SHH, and this subgroup may be the first to benefit from routine targeted therapy [62]. Owing to the favorable prognosis of WNT-driven tumors, most attention has focused on the optimization of current treatment approaches (i.e., radiation, non-specific chemotherapy, and surgery). With continued characterization of Group 3 and Group 4 tumors, new genomic targets may become available in coming years.
Meningioma
For decades, the only genetic alteration associated with meningioma was biallelic loss of the tumor suppressor neurofibromin 2 (NF2), which is found in approximately 50% of sporadic cases [63]. Prior to 2013, numerous studies had investigated the gene expression patterns and copy number events in these tumors or used candidate approaches to elucidate possible oncogenic mechanisms [64–66]. However, the recent use of unbiased methods has led to important insights about meningioma pathogenesis, identifying five pathways that are altered in over 80% of cases [67–69]. Besides NF2 loss, exome sequencing of tumor–normal paired samples has revealed recurrent activating mutations in the PI3K signaling molecule V–akt murine thymoma viral oncogene homolog 1 (AKT1) and sonic hedgehog (SHH) mediator smoothened (SMO). Additionally, mutations in genes not previously associated with cancer have been identified. Somatic mutations affecting the WD40-repeat domain of TNF receptor–associated factor 7 (TRAF7) were found in approximately one-quarter of sporadic meningioma. Interestingly, these mutations frequently co-occur with either AKT1 activating mutations, or a recurrent K409Q alteration in the DNA-binding domain of Kruppel–like factor 4 (KLF4). KLF4 is one of four Yamanaka factors sufficient to induce pluripotency from somatic cells [70]. Recent work has also identified recurrent mutations in the dock domain of RPB1, the largest and catalytic subunit of RNA polymerase II [69].
While activating mutations in PI3K and SHH signaling are involved in numerous forms of cancer, the mechanisms underlying other identified meningioma genes remain unclear. Downstream molecular studies, similar to those undertaken in glioma and medulloblastoma, will provide important insights over the coming years and may reveal pharmacologic targets. Despite the identification of genomic drivers in the vast majority of sporadic meningioma, the primary treatment modality remains neurosurgical excision. While this procedure is curative in most cases and carries relatively low risk, it is an invasive procedure that is not without complications [71]. Medical therapies for meningioma have been investigated previously, but this occurred prior to the identification of recurrent somatic events in these tumors and therefore targeted general candidate pathways [72, 73]. Higher-grade meningiomas (World Health Organization grades II and III) in particular may benefit from targeted therapies, as they are associated with aggressive features and carry relatively poor prognosis [74]. As the oncogenic mechanisms of TRAF7, POLR2A, and KLF4 remain largely unknown, clinicians have focused on leveraging treatments from other tumor types to target the well-established PI3K and SHH pathways in meningioma. Notably, clinical trials have started for the treatment of recurrent meningiomas that harbor activating SMO mutations using SHH pathway inhibitors [75].
Tumor Heterogeneity and the Need for Personalized Approaches
As the cost of NGS exponentially dropped after 2008, further analysis of individual tumors with higher resolution revealed another level of complexity: intra-tumoral heterogeneity (Fig. 3.4). Heterogeneity within a single tumor is caused by the presence of different genetic alterations in distinct subclones that carry separate biological functions for the tumor to survive and proliferate [76]. The complexity caused by both intra- and inter-tumoral heterogeneity is sufficient to make the classic “one-size-fits-all” impractical for most patients. Therefore, detailed genomic characterization of individual tumors is essential to tailor the most effective treatment regimen, making the treatment personalized.
In this section, we will use a series of studies to depict why conventional one-size-fits-all approaches for cancer treatment have not been consistently successful in malignant brain tumors and how advanced genomic technologies promise to improve outcomes. We will specifically focus on the causes of treatment resistance, how these resistance mechanisms have been revealed with genomic technologies, and how genomic information can be utilized to overcome these mechanisms.
Gliomas, the most common malignant brain tumor, are an excellent model to discuss the clinical use of advanced genomics. Genomic data from this tumor have revealed the reasons for variability in not only the treatment response, but also for prognostic markers. Temozolomide (TMZ) is an alkylating chemotherapeutic agent commonly used in gliomas as a standard of care treatment. As discussed earlier, a prognostic marker in gliomas for assessing the response to TMZ treatment is the methylation status of the MGMT promoter region [77]. However, it has also been shown that prognostic value of this marker is dependent on the genomic background of the tumor, such as existence of an IDH1 mutation [78]. In another prognostic marker study, the tumors with mutant TP53 were shown to be less sensitive to TMZ treatment. Even though there are no other chemotherapy agents as effective as TMZ that can be used as an alternative in gliomas, it is clear that genomic profiling of individual tumor improves the prognostic assessment for each patient.
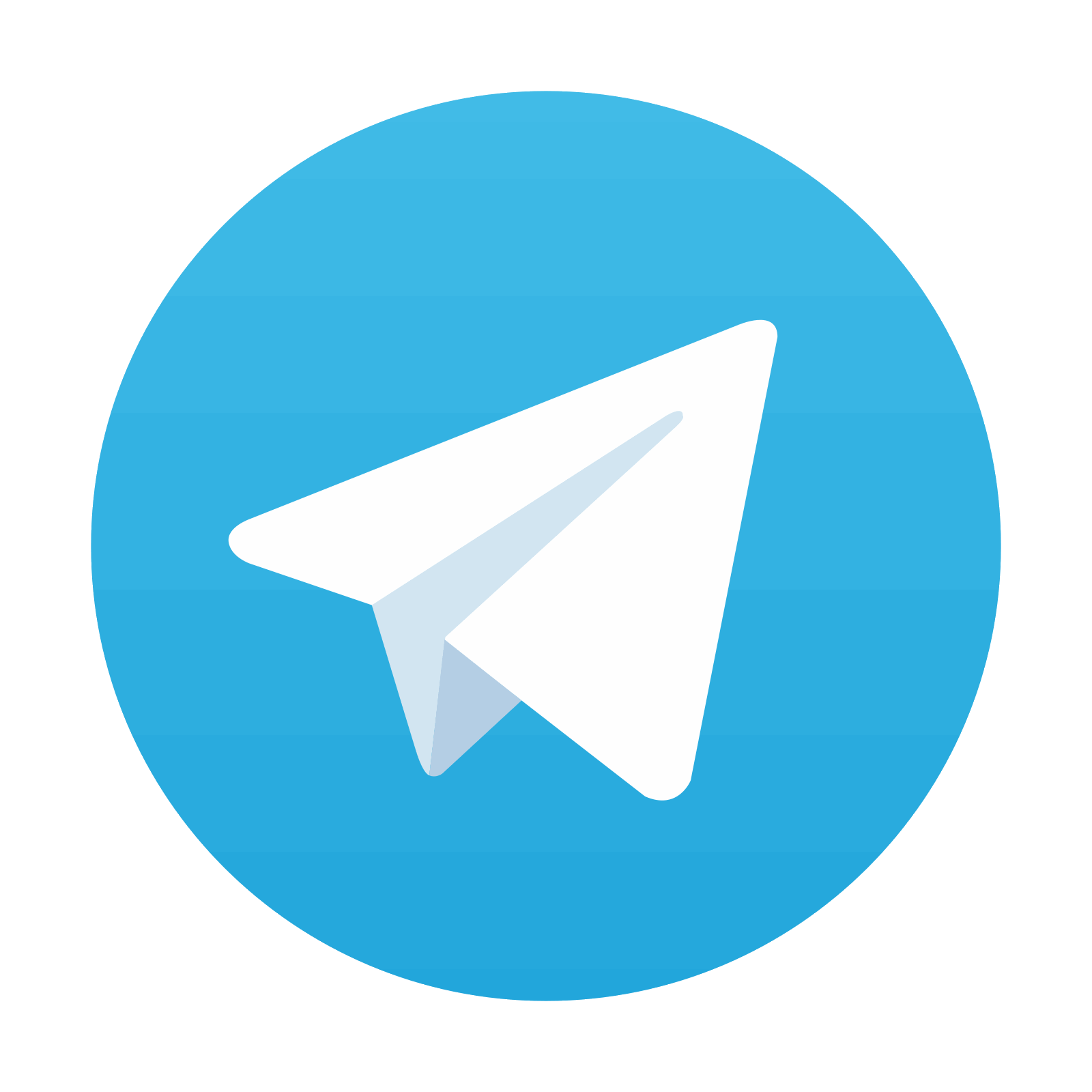
Stay updated, free articles. Join our Telegram channel

Full access? Get Clinical Tree
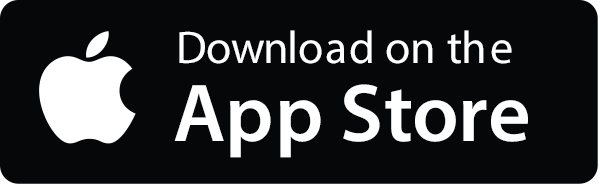
