(2.1)
For the analysis of fMRI data, the given data Y i at time index i is


(2.2)
where x i1 ,…, x ik is the combined effect of k for different stimulus types in scan i and is often assumed to be additive but with different coefficients β i1 ,…, β ik that vary from voxel to voxel.
The combined fMRI response is modeled linearly as x i1 β i1 +⋯+ x ik β ik. The extra “responses” x i , k + 1 ,…, x i , m at time i show the considerable drift over time of some voxels in fMRI time series data. Accordingly, x i , k + 1 β i , k + 1 +⋯+ x im β m describes the drift. ε i is the random error.
The GLM aims to test whether the dynamic characteristics of neural responses in specific brain regions relate with some known input function (e.g., experimental design convoluted with hemodynamic function). GLM has arguably become the most widely used method for analyzing fMRI data and has proven particularly powerful for the analysis of event-related designs, given that a sequence of sparse events occurring at random intervals affords a relatively specific predicted response, and good fit is often interpreted in terms of signal evoked by a particular psychological event (Worsley and Friston 1995; Wager et al. 2005). However, when the precise timing and duration of psychological events cannot be specified using a priori defined regressors that model predicted responses to psychological events of interest, the GLM becomes impractical (Lindquist et al. 2007).
2.2.2 Independent Component Analysis (ICA)
The ICA transforms multivariate random signal into signal with multiple statistically independent components. ICA models have traditionally been used to perform blind separation, feature extraction, and signal detection, resulting in the development of many batch and adaptive algorithms. The ICA does not require a priori knowledge or specific assumptions about the time courses of processes contributing to the measured signals. In addition, ICA appears to have a broad developing prospect in the analysis of fMRI data from healthy and clinical populations, especially in the context of unpredictable transient patterns of brain activity associated with psychomotor task performance. A schematic diagram of ICA is shown in Fig. 2.1, where X is the result of multisource signals multiplied by mixing matrix A, X = AS. We can obtain the solution of mixing matrix B when S and A are unknown, resulting in Y = BS as the optimal approximation of S.


Fig. 2.1
Schematic diagram of the independent component analysis (Reprint with permission from Dai et al. 2013)
Given the central limit theorem, the sum of independent random variables tends to follow a Gaussian distribution. Accordingly, the sum of independent random variables should be closer to the Gaussian distribution than any one variable involved. As a consequence, the combination yields the maximization of non-Gaussianity, such that the random variables most remarkably deviating from the Gaussian distribution can be regarded as one independent component of X. This method is called the non-Gaussian maximization method. In addition, there are many other methods used to estimate the ICA, such as approaches to estimate the mutual information minimum and maximum based on the information theory. Many ICA algorithms have been derived from this, such as maximizing or minimizing some related cost function, the adaptive algorithm based on a stochastic gradient, the widely used FastICA algorithm, and the Infomax algorithm. Of note, the Infomax algorithm is commonly used in the analysis of the fMRI data and looks for solutions to a mixed matrix in order to effectively separate multiple Gaussian source signals.
McKeown and colleagues first applied the ICA method as an fMRI analysis in 1998 (McKeown et al. 1998). In this study, the authors demonstrated that ICA could be applied to distinguish artifacts, non-task-related signal components, as well as continuous or temporary assignment-related fMRI activations, based on a weak assumption about their spatial distributions and without a priori knowledge about the time source.
The temporal ICA (TICA) algorithm is commonly used under conditions where the times at which signals are collected are mutually independent from one another. In the TICA, data collected from the same voxel in L time points are regarded as a mixed component, so that the whole brain data is composed of M (the number of voxels) mixed components. TICA applied to whole brain data generates a series of independent time series and corresponding brain images.
Spatial ICA (SICA) is commonly used under the assumption that all components are mutually independent from one another, with each source represented as a spatially fixed pattern of activation. In the SICA, voxel-wise data throughout the brain collected at the same time point are regarded as a mixed component, so the whole brain data is composed of L (the number of time points) mixed components. SICA in whole brain data generates a series of independent images and corresponding time series.
2.2.3 Functional Connectivity Analysis
The concept of functional brain connectivity was proposed in early electrophysiological studies. In the early 1990s, Friston et al. introduced the concepts of functional and effective connectivity and applied them to issues in neuroscience (Friston et al. 1993a, b). Functional connectivity was initially defined as a temporal correlation between spatially remote neurophysiological events occurring in a neuroimaging time series. This definition was operational and provided a simple characterization of functional interactions. In 2000, Sporns et al. published a more precise definition of functional connectivity, stating that functional connectivity captures patterns of deviations from statistical independence between distributed and often spatially remote neuronal units, measuring their correlation/covariance, spectral coherence, and phase locking. Of note, functional connectivity does not provide any direct insight into how correlations are mediated (Sporns et al. 2000).
By contrast, effective connectivity can be defined as the influence of one neural system on another, occurring at either a synaptic or cortical level. Some studies have shown that structural equation modeling can be conceived as a linear regression model for effective connectivity, while dynamic causal modeling can improve analyses of brain connectivity to be more akin to analyses of regionally specific effects (Friston 1994; Mclntosh and Gonzalez-Lima 1994; Buchel and Friston 1997; Mechelli et al. 2003).
There are two popular methods for functional connectivity analyses: the seed-based correlation analysis method and the multivariate analysis method, which includes ICA and principal component analysis (PCA) approaches. The specific processing steps for functional connectivity analysis include (1) image preprocessing, including realignment, spatial normalization, spatial smoothing, band-pass filtering, and covariate regression, (2) region-of-interest (ROI) selection to investigate specific regional correlations, (3) calculation of the correlation coefficients between the reference time course (in previous studies, the averaged time courses of voxels within a given ROI) and the BOLD time course from all of other brain voxels, and (4) normalization of the distribution by applying Fisher’s Z transform to the correlation coefficients.
In step 3 of this approach, correlation coefficients less than the given threshold are set to zero. The calculation is described as follows:


(2.3)
where r is the reference time course, S is the signal for a given voxel,
is the reference time course for a given voxel, and
is the time series for a given voxel. The summation is performed for all time points. Because fMRI time series data can inform both temporal and spatial correlations, it can be expected that the distribution of correlation coefficients will be skewed due to intrinsic temporal and spatial correlations from the imaging method (Friston et al. 1994a; Lowe et al. 1998). The distribution of correlations can differ depending on the ROI selected as the reference for the correlation due to artifacts arising from cardiac, respiratory, or other physiological “noise” during volume collection (Lowe et al. 1998; Hampson et al. 2006).


In step 4, Fisher’s Z transform is applied as follows:


(2.4)
At present, major neuroimaging studies of acupuncture primarily report acupuncture-induced activation patterns. Functional connectivity analyses can be applied to correlations analyses of multi-brain region activation following acupuncture stimulation in order to better inform the neural networks that underlie acupuncture effects.
2.2.4 Time Series State Analysis Algorithm Based on the Change-Point Theory
As mentioned above, although the GLM approach has arguably become the most popular way to analyze fMRI data, it becomes impractical when the precise timing and duration of psychological events cannot be specified a priori. The hierarchical exponentially weighted moving average (HEWMA) model is an approach that allows the predicted signal to depend nonlinearly on the input, using ideas from statistical control theory and change-point theory to model slowly varying processes for which the onset times and durations of underlying psychological activity are uncertain. The HEWMA model is a multi-subject extension of existing EWMA models for individual participants (and accordingly a single time series), adapted to be applicable to a cohort analysis using a hierarchical model. The HEWMA method can thus be used for fMRI data analysis in a voxel-wise manner throughout the brain, for ROI data, or for temporal components extracted using an ICA or a similar method (Wager et al. 2005; Lindquist et al. 2007).
A schematic diagram of the time series state analysis algorithm based on the change-point theory is shown in Fig. 2.2. Given a process that produces a sequence of observations
(e.g., a fMRI time series), a two-state model can be produced where data is modeled as the combination of two normal distributions, one with mean θ 0 and covariance matrix Σ and the other with mean θ 1 and the same covariance. In the baseline acquisition period, the process generates a distribution of data with mean θ 0, and the process in this state is considered to be in control. Observations maintain this distribution until the change point τ, when the process changes (i.e., a new psychological state results in increased or decreased neural activity), generating fMRI observations from the second distribution with mean θ 1 (Fig. 2.3). In this second period, the process is deemed to be out-of-control or in the out-of-control state (Lindquist et al. 2007).




Fig. 2.2
Schematic diagram of the time series state analysis algorithm based on the change-point theory (Reprint with permission from Dai et al. 2013)

Fig. 2.3
Schematic of the model of true activation (Reprint with permission from Lindquist et al. 2007)
2.3 Acupuncture Neuroimaging Studies in Humans
Noninvasive brain imaging techniques, including positron emission tomography (PET) and fMRI, have provided the opportunity to examine the effects of acupuncture on brain activation. Accordingly, significant modulatory effects of acupuncture have been identified at various levels throughout the CNS. This include the endogenous antinociceptive limbic networks (the cingulate cortex, insula, and hypothalamus) as well as higher-order cognitive and affective control centers within the prefrontal cortex and medial temporal lobe (the amygdala and hippocampus) (Wu et al. 1999, 2002; Hui et al. 2000, 2005; Kong et al. 2002; Zhang et al. 2003; Liu et al. 2004; Yoo et al. 2004; Pariente et al. 2005; Napadow et al. 2007). Here, we will review literature reporting the effects of acupuncture on brain activation.
In 1998, Cho et al. first reported that the vision-related acupoint stimulation at VA1, VA3, and VA8 could activate the primary visual cortex (Cho et al. 1998). However, acupoint specificity has not been fully supported by other fMRI studies. For example, while Cho’s results were successfully reproduced by Siedentopf et al. (2002) using laser acupuncture, Gareus et al. (2002) failed to replicate these findings.
Neuroimaging data strongly suggest that acupuncture modulates many distributed cortical and subcortical areas of the brain involved in endogenous antinociception and the pain neuromatrix (Davis et al. 1997; Fields and Basbaum 1999; Hofbauer et al. 2001). Modulation of these areas may contribute to the therapeutic effects of acupuncture by shifting autonomic nervous system (ANS) balance and altering the affective and cognitive dimensions of pain processing (Peets and Pomeranz 1978; Clement et al. 1980). Wu et al. found that stimulation at ST36 produced specific increases in signal intensity of the hypothalamus and nucleus accumbens and specific decreases in signal intensity of the rostral anterior cingulate cortex (ACC), amygdala, and hippocampus (Wu et al. 1999). This evidence supported the hypothesis that acupuncture activates structures of the descending antinociceptive pathway and deactivates multiple limbic areas subserving the affective dimension of pain. Similarly, Hui et al. reported that needle stimulation at LI4 on either the right or left side produced consistent modulation of multiple cortical and subcortical limbic and paralimbic structures; moreover, this stimulation induced a wider range of negative signal changes in the limbic-cerebellar system such as the nucleus accumbens, amygdala, hippocampus, parahippocampus, hypothalamus, anterior cingulate gyrus (BA 24), temporal pole, ventral tegmental area, caudate, putamen, and insula (Fig. 2.4) (Hui et al. 2005). In addition, decreased neural responses were only identified in subjects that experienced deqi sensations not in the subjects who experienced pain sensations during needle manipulation. In contrast, two subjects who experienced sharp pain showed predominately increased signal intensity in the parahippocampus, ACC, posterior cingulate cortex (PCC), and putamen. Given that deqi is thought to play a pivotal role in the therapeutic effect of acupuncture (Takeda and Wessel 1994; Witt et al. 2005), observed decreases in activity in the limbic-cerebellar network may be a central characteristic of acupuncture’s therapeutic effect.


Fig. 2.4
The influence of subjective sensations on fMRI signal changes on major limbic structures, the secondary somatosensory cortex (SII) and the cerebellum during acupuncture at ST36 (Reprint with permission from Hui et al. 2005). (Row A) The amygdala showed signal decrease with acupuncture deqi, increase with sensory stimulation, and no significant change with acupuncture mixed sensations. (Row B) The hippocampus, bottom arrows, showed signal decrease with acupuncture deqi and no significant change otherwise. (Row C) SII, also shown by the right arrows in Row B, shows signal increase under all three stimulations. Acupuncture, being a form of sensory stimulation, would be expected to result in signal increases in SII, which is in stark contrast to the widespread signal decreases during acupuncture deqi. (Row D) With acupuncture deqi, the cerebellum showed signal decreases in the vermis and lobules VI and VII
Biella et al. (2001) demonstrated that true acupuncture at Zusanli (ST36) and Qi-ze (Lu 5) statistically increased regional cerebral blood flow (rCBF) in the ACC, bilateral insula, bilateral cerebellum, right superior frontal gyrus, and right medial frontal gyrus, whereas sham acupuncture (superficial needle insertion 1 cm lateral to each acupoint) increased rCBF in the raphe nuclei, hypothalamus, and left temporoparietal junction. Common areas of activation included the claustrum, caudate, putamen, medial and bilateral inferior frontal gyri, and (to a lesser degree) the right anterior insula. Chronic and acute pain can induce activation in pain-related areas including the ACC, insula, and cerebellum. Moreover, the ACC is thought to be involved in the estimation and modulation of sensory and cognitive signals. This is in agreement with indications that the ACC is involved in a number of pain responses, pain anticipatory mechanisms, and pain-related cognitive procedures (Derbyshire et al. 1997; Rainville et al. 1997, 1999; Casey 1999) including attention to pain sensation (Davis et al. 1997).
Yoo et al. reported that acupuncture at PC6 induced the activation of spatially distinct neural substrates, including cerebral structures and areas of the cerebellum such as the declive, nodulus, uvula, quadrangular/superior semilunar lobules, and left cerebellar tonsil. However, neither sham acupuncture nor tactile stimulation elicited cerebellar activation, as shown in Fig. 2.5 (Kong et al. 2002). Liu et al. demonstrated that acupuncture at LI4 (Hegu) but not at a sham acupoint induced activation of the periaqueductal gray (PAG) and moreover reported that the somatosensory cortex (BA 3, 40) and PAG show distinct activity patterns in response to acupuncture (Liu et al. 2004). Yan et al. found that acupuncture at Liv3 (Taichong) and LI4 (Hegu) but not at sham acupoints activated areas in the middle temporal gyrus and cerebellum and deactivated areas in the middle frontal gyrus and inferior parietal lobule, with some degrees of acupoint specificity. That may reveal that acupuncture at acupoints induces specific patterns of brain activity and these patterns may relate to the therapeutic effects of acupuncture (Yan et al. 2005).


Fig. 2.5
Group activation results among the three different stimulation conditions (Reprint with permission from Yoo et al. 2004). (a) Results comparing the rest condition to sham acupuncture and tactile stimulation. Group activation maps are shown for the (A) sham acupuncture > rest condition and (B) tactile stimulation > rest condition. (b) Results comparing real acupuncture to sham acupuncture and tactile stimulation. Group activation maps are shown for the (A) real acupuncture > sham acupuncture condition and (B) real acupuncture > tactile stimulation condition
Some studies have demonstrated that different acupuncture manipulations result in different patterns of brain activation. Napadow et al. (2005) reported that EA (particularly at low frequency) produced more widespread fMRI signal increases than manual acupuncture and that all acupuncture stimulations produced more widespread responses than placebo tactile control stimulation. Furthermore, only EA generated significant activation in the anterior middle cingulate cortex (MCC) and pontine raphe area.
2.3.1 Sustained Effects of Acupuncture and Its Influence on fMRI
To date, most neuroimaging studies of acupuncture have mainly focused on the acute impacts of acupuncture on brain activity, adopting the general linear model (GLM) for data analysis. Moreover, a large proportion of previous fMRI studies have employed a block experimental design, which is based on a stimulation-response model in which BOLD signal is assumed to change in response to stimulation and immediately return to the pre-stimulus state after the cessation of stimulation. With this approach, a specific stimulus sequence (i.e., design matrix) is used to define an ideal hemodynamic response function (HRF) , which is convolved with the actual hemodynamic response and produces predictors of the BOLD response (Worsley and Friston 1995). Block design fMRI paradigms are useful for detecting activation in response to typical visual or motor tasks, as temporal changes in the BOLD signal conform to the stimulation-response model in these settings. However, a block design may be not valid in cases when a stimulus-response behavior has not been confirmed, as in the case of acupuncture stimulation.
Acupuncture has been shown to exert sustained effects on brain activity even after the cessation of needle stimulation (Price et al. 1984), suggesting that acupuncture effects may have time-variant properties that are acupoint specific. To this end, many clinical reports have indicated that acupuncture produces therapeutic effects that long outlast the duration of actual therapy (Beijing and Nanjing Colleges of Traditional Chinese Medicine 1980). A psychophysical analysis by Price et al. (1984) also suggested that the analgesic effects of acupuncture peak long after the completion of a needling session. On the hypothesis that acupuncture has sustained effects, the temporal aspects of BOLD responses to acupuncture are likely to violate the assumptions of block design GLM estimates (Fig. 2.6) (Bai et al. 2009b) in that relevant BOLD responses are unlikely to return to baseline after initial stimulation. Indeed, an investigation using an ICA provided direct evidence that interleaved resting epochs in the block design paradigm retain acupuncture-related changes (Zhang et al. 2009). On the basis that the temporal profile of acupuncture is slow to develop and resolve, more appropriate design paradigms and statistical models should be selected in future studies in order to elucidate the actual effects of acupuncture.


Fig. 2.6
Activity patterns of representative areas in different epochs of multi-block following acupuncture stimulation at ST36 (Reprint with permission from Bai et al. 2009b). Corresponding t-values of representative regions in different periods are also indicated. Error bars indicate the standard error of the mean. (A, B) The amygdala (Amy), hippocampus (Hipp), and parahippocampus (PH) exhibited weakly positive responses in the first stimulation (A1) that decreased to below baseline level thereafter. (C) Early positive and negative signal responses were notable in the dorsal and pregenual anterior cingulate cortex (dACC and pACC), respectively, in sequential conditions. (D, F) The anterior and posterior insula (AI and PI) and secondary somatosensory cortex (SII) exhibited persistently increased responses during the whole trial. (A, E) Episodic responses were primarily distributed in the hypothalamus (Hyp) and brainstem structures (periaqueductal gray, PAG; substantia nigra, SN). The greatest positive activity emerged in R1 and plateaued in A2 but was nonsignificant. (G) Early responses in the cerebellum (culmen and declive) were positive and later became negative
One major obstacle to the multi-block design is the difficulty of distinguishing between brain activity related to acupuncture manipulation itself (e.g., sensation, attention, and cognition) and activity associated with its sustained effects. Napadow et al. (2009a) adopted a long duration (>30 min) stimulus paradigm and compared ST36 acupuncture with sham point acupuncture to evaluate time-variant brain responses (Fig. 2.7). They found that ST36 verum acupuncture modulated activity in the substantia nigra, nucleus raphe magnus, locus coeruleus, nucleus cuneiformis, periaqueductal gray, and limbic areas, whereas both stimulations produced linear decreases in the time-variant activation of sensorimotor brain regions (i.e., the SII, insula, and premotor cortex). These data indicated that acupuncture produces to both early (immediate) and late (30 min after the start of stimulation) phases of brain activation. To address this possibility, Qin et al. (2008) proposed a novel experimental paradigm, non-repeated event-related fMRI (NRER-fMRI) , to explore the prolonged effects of acupuncture on resting-state brain networks. Compared with the acupuncture at a nearby non-acupoint, ST36 acupuncture produced higher-level correlations among amygdala-associated networks; these areas mainly included limbic/paralimbic areas (the ACC and insula) and brainstem structures (the PAG ) (Fig. 2.8). Similarly, acupuncture but not sham stimulation produced increased connectivity in the default mode network and sensorimotor network (Dhond et al. 2007), including the medial temporal lobe, PAG, and supplementary motor area (SMA) . Connectivity between the hippocampus and DMN was also correlated with parasympathetic output following acupuncture stimulation in the abovementioned study. These data indicated that autonomic modulation might represent an important therapeutic mechanism of acupuncture leading to sustained effects, which is consistent with what is known regarding autonomic efferent nerve activity and the analgesic effects of acupuncture (Haker et al. 2000; Hsu et al. 2007; Sakai et al. 2007). In further support of this hypothesis, Baliki et al. (2008) reported differences in resting-state brain functional connectivity between individuals with chronic pain and healthy control subjects and proposed that differences were related to the cognitive and affective components of chronic pain. Future exploration of the alternating interplay between external acupuncture intervention and the reorganization of resting-state brain networks in chronic pain patients will better inform our understanding of the mechanism of acupuncture analgesia.



Fig. 2.7
An fMRI block design paradigm consisting of alternating 1-min stimulation (ST36 or sham acupoint) and rest blocks (Reprint with permission from Napadow et al. 2009b)

Fig. 2.8
Acupuncture enhanced activity correlations between the amygdala and the insula, anterior cingulate cortex, and periaqueductal gray poststimulus (Reprint with permission from Bai et al. 2009a), whereas sham stimulation enhanced activity correlations between the amygdala and the secondary somatosensory cortex and cerebellum. ACV, anterior commissure verticalization
If acupuncture exhibits temporally delayed effects, an understanding of its temporal properties is crucial. One pioneer study developed a novel approach (defining separate models) to evaluate dynamic signal changes between baseline activity and post-acupuncture neural activity in sequential epochs within a multi-block design (Bai et al. 2009b). The results showed that ST36 acupuncture induced time-varied response patterns in limbic-cerebellar and brainstem structures (Fig. 2.6). Moreover, it was noted that some regions only responded to initial acupuncture stimulation (i.e., the PCC), while others exhibited increasing or tapering activities for the duration of the experimental session (i.e., the PAG and rostral ventromedial medulla [RVM]). Further, other brain areas showed sustained responses (i.e., the insula) and continuously exerted controlling and coordinating influences throughout the scan. Limbic and brainstem areas have been indicated to support endogenous antinociceptive mechanisms as part of the pain neuromatrix. In addition, evidence from animal studies suggests that acupuncture analgesia is supported by endogenous opioidergic and/or monoaminergic antinociceptive networks (Hoffman et al. 2005). The PAG and RVM together serve as one such mechanism that modulates ascending nociceptive responses by promoting the release of endogenous opioids (Napadow et al. 2005). The RVM has distinct functional populations of neurons that inhibit (off cells) and facilitate (on cells) nociceptive transmission (Haws et al. 1989; Tortorici and Vanegas 1994). Accordingly, the PAG-RVM network may function as a unit to exerting discrete global control over dorsal horn pain transmission in the context of acupuncture analgesia. In addition, PAG activity is facilitated by top-down processes originating in higher centers such as the insula and ACC (Fields et al. 1991; Urban and Gebhart 1999; Millan 2002; Porreca et al. 2002). These areas, along with limbic regions including the hippocampus and amygdala, comprise the descending antinociceptive pathway. Thus, it can be theorized that the mechanism of acupuncture analgesia may relate to altered pain perception mediated by inhibiting the antinociceptive action in the affective pathway while mobilizing the descending mechanisms by controlling the transmission of nociceptive signals to the brain.
2.4 Brain Network Analysis Methods
2.4.1 Graph Theory in Brain Network Analysis
Brain systems are frequently comprised of smaller, dynamic, and highly interacting subsystems. Graph theory is one method used to study the general features of these systems. In the context of graph theory, dynamic subsystems are represented as network nodes, and subsystem interactions are represented as network edges. Graph theory model differs from traditional network analysis methods in that it is more focused on subsystem interactions than subsystem structure and function.
2.4.1.1 Common Network Parameters According to Graph Theory
The principle of graph theory is summarized in Fig. 2.9. A network consists of two sets: set V of nodes and set E of edges. N, the size of set V, represents the size of the network. Homogeneity among the elements of set V reflects whether nodes in a given network have the same properties. Element linkij in set E is a combination of two elements, node i and node j in set V, and indicates that there is an edge between node i and node j. If (k, j) is an ordered pair, the network can be seen as directed; otherwise, it is undirected. If each element in set E has a different value, the network is a weighted network; otherwise, it is unweighted. Generally, an edge that connects with itself without passing other nodes does not exist in set E, which means that the combination of (j, j) does not exist. Moreover, in an undirected network, there is only one connected edge between two different nodes.


Fig. 2.9
Different kinds of networks according to graph theory (Reprint with permission from Dai et al. 2013). (a) Undirected network; (b) dire cted network
In a network, the path between two nodes is defined as a non-repetitive sequence of nodes and edges passed from one node to another. The length of the path is defined as the sum of the length of mid-edges in the sequence. The shortest length of path is called as characteristic path (L). If any two nodes can be reached by a path, the network is a connected network; if a network cannot be connected as a whole, the largest connected network in it is studied and used to make generalizations.
The degree value k i of any node of a network indicates the sum of nodes that are directly connected with the given node. The degree value can therefore be obtained by summing over the elements of line i in an adjacency matrix; that is to say, k i = ∑j ∈ va j. If the network is a directed network, the degree of nodes can be divided into two parts, in-degree
and out-degree
, such that the total value of the degree is
. The degree value accordingly represents the role that a given node plays in a network.



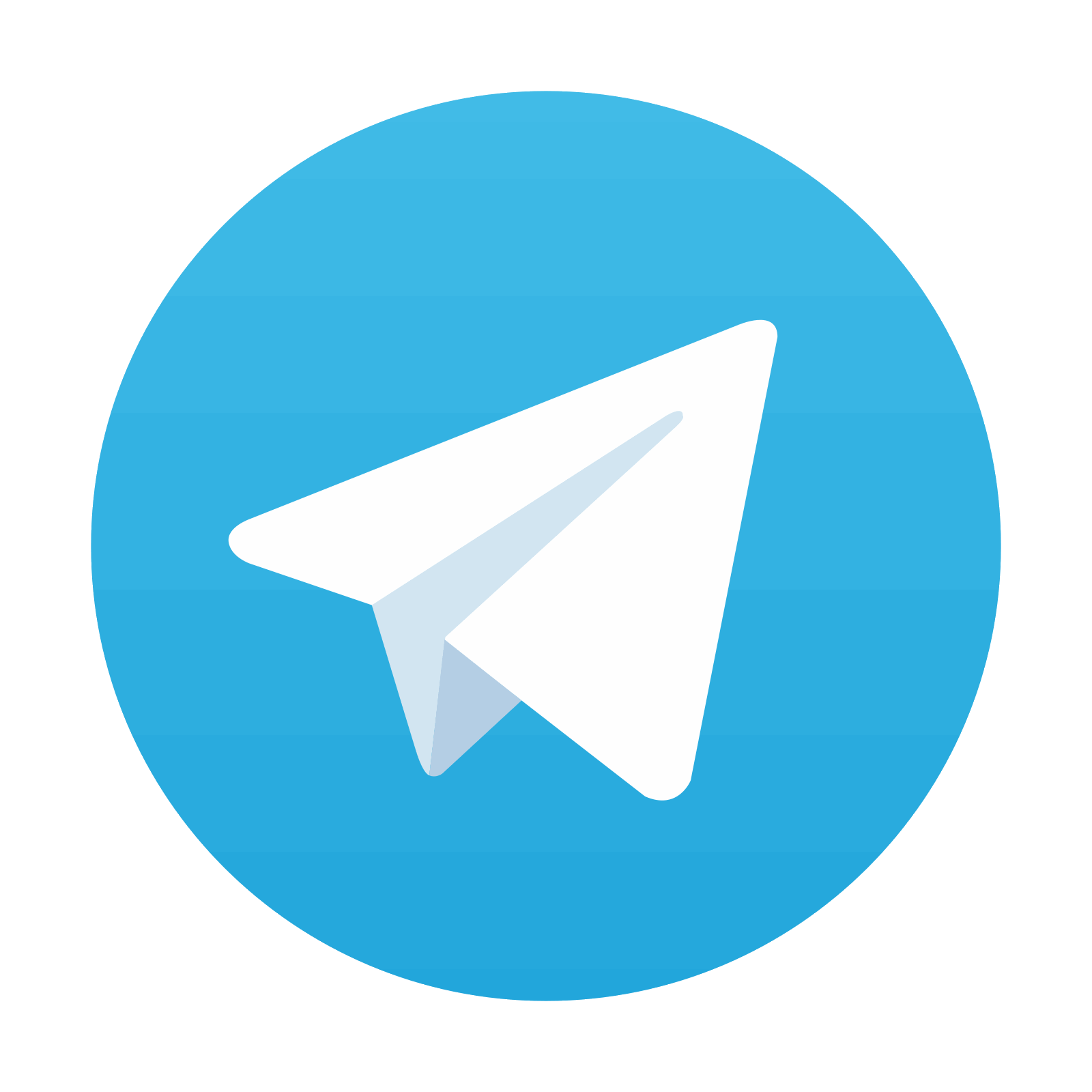
Stay updated, free articles. Join our Telegram channel

Full access? Get Clinical Tree
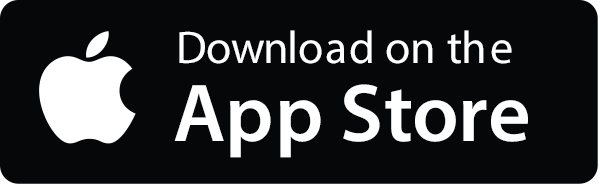
