Associational Cortex And Memory
The processes we look at in this chapter are those that we humans tend to be inordinately proud of, and to which we owe any temporary biological success that we have managed to achieve. Many animals are more agile and better coordinated than we are, can hear better or see better, and nearly all of them have more sensitive noses. Our special virtue is that we are quite good at storing and processing such sensory information as gets through to us, so that we use it to respond more effectively to our environment. But this difference is quantitative, not qualitative: there is no very sharp distinction between us and other creatures in this respect. It is simply that the parts of their brains that carry out these functions in a rudimentary way have in us been very greatly expanded and developed, above all the cerebral cortex.
The organization of associational cortex
If we compare the cerebral hemispheres of a series of animals from different evolutionary stages, what is striking when we get to Man is not just the expansion in the absolute mass of neural tissue, but the dramatic changes in the relative proportions of the cortex devoted to different functions. Very little of a rat’s cortex is not either primary motor or a projection area for one of the senses; in Man, by contrast, most areas of the cortex neither respond in an obvious way to simple sensory stimulation, nor produce movements when electrically activated: they are what have sometimes been called silent areas. 

![]() |
![]() |
Now these are precisely the properties we would expect from neural levels in the middle of the model of the brain shown above, that was first presented in Chapter 1. Because a neuron in any level is activated only by a particular pattern of activity in the preceding layer, as we penetrate deeper into the sensory side we find that individual neurons become fussier and fussier about what they respond to, and eventually the chance of our finding out, in an experiment of finite duration, what they do actually do becomes vanishingly small. Stimulation is equally frustrating: unless we happen to stimulate them in a pattern that makes some kind of neural sense, corresponding to what is needed to activate the next layer along, nothing will happen at all. Both these problems are accentuated in cortical areas, where there is an immense degree of convergence and divergence from neurons in one column to those in others.
The third weapon in the neurophysiologist’s armoury, lesioning, is similarly blunted. Because these areas
integrate or associate information from diverse sources which cut across the conventional divisions of sensory modality (which is why the corresponding cortical areas are called association areas), the effects of lesions often lack the functional specificity found, for example, in damage to the primary visual or motor cortex: we are at a high hierarchical level, in the sense discussed in Chapter 9. Lesions can often lack spatial specificity as well, and there is little of the topological orderliness found at more peripheral levels. This situation is rather like what happens in a telephone exchange: at the periphery – the region where the incoming cables arrive – there is a systematic relationship between a subscriber’s number and the position of his particular connection, but the circuits in the heart of the exchange that set up the connections and form, in effect, associations between different subscribers, are shared by all of them and used to set up different circuits on different occasions, and therefore have no obvious spatial organization.
integrate or associate information from diverse sources which cut across the conventional divisions of sensory modality (which is why the corresponding cortical areas are called association areas), the effects of lesions often lack the functional specificity found, for example, in damage to the primary visual or motor cortex: we are at a high hierarchical level, in the sense discussed in Chapter 9. Lesions can often lack spatial specificity as well, and there is little of the topological orderliness found at more peripheral levels. This situation is rather like what happens in a telephone exchange: at the periphery – the region where the incoming cables arrive – there is a systematic relationship between a subscriber’s number and the position of his particular connection, but the circuits in the heart of the exchange that set up the connections and form, in effect, associations between different subscribers, are shared by all of them and used to set up different circuits on different occasions, and therefore have no obvious spatial organization.
![]() |
The analogy of the telephone exchange, introduced in Chapter 1, is a suggestive one. Just as an exchange is capable of connecting any subscriber to any other, so cortical convergence and divergence guarantees to provide a neural pathway from any sensory stimulus to any motor response, clearly a necessity for complete flexibility of behaviour. Similarly, the capacity of an exchange – the number of associations it can make at any one time – is simply proportional to the quantity of the common switching equipment it contains. Might the neural elements of associational cortex also be in some sense shared in this way? Such a notion, of associational cortex being uncommitted to any particular task, but providing a reserve of computing power that can be applied to whatever job is on hand, was originally suggested by the experiments of Karl Lashley described on p. 15 – Lashley’s extreme statement, his ‘Law of Mass Action’ – that the effect of lesions in associational cortex depends more on how large they are than on their exact location – is now less in favour. Recording from units in associational areas shows in many cases that they are ‘silent’ because we are using unnatural or boring stimuli; with adequate sensory patterning they can often be made to respond, in a way that may be complex and highly time-dependent, but does not alter radically from one experiment to the next. And it is clear from clinical observations in particular that discrete lesions in associational cortex can often lead to relatively specific functional defects, rather than something like a generalized loss of ‘intelligence’. What is true, as we shall see, is that these functional defects may be of the wide-ranging and subtle kind that is characteristic of damage at a high hierarchical level – for instance the loss of the ability to speak French, while spoken English is unimpaired – and also that there is little reproducibility from subject to subject, in the sense that a lesion in a particular place in one person may have a completely different effect in another person. Such nuanced deficits are not readily appreciated by the busy non-specialist clinician, for several reasons. First, the commonest cause of discrete cortical lesion is a cerebral infarct, usually as a result of occlusion of a large cerebral artery or even a carotid. Among the devastation wrought by such a large lesion – paralysis, hemianopia, aphasia, hemineglect – impaired performance of higher, more specialized areas is often obscured; recognition is made particularly difficult as patients often appear curiously unaware of their loss. Second, despite advances in our diagnostic techniques and our understanding of the pathogenesis of stroke, our ability to do much about it is frustratingly rudimentary and, certainly in the acute and early management of a stroke, any variation in the treatment administered is guided by the grossest of clinical signs, such as the presence of significant limb weakness or a marked visual deficit. Subtle difficulties with mental arithmetic or recognizing street names are not identified because they are not sought, and not sought because, at this stage, they have only a limited bearing on clinical management.
In addition, we shall see clear evidence that cortical neurons can change their function to help cope with a change in functional demand. Thus the idea of a completely uncommitted pool of ‘brain power’ – rather like the ‘cloud computing’ that enhances the capabilities of smartphones – is an over-simplification; at a given moment the neurons are specialized in their function, but at a high level in the hierarchy: it is this that gives lesions in associational cortex their subtle and unpredictable quality. Cortex might be described as a community of specialists, acting in concert through an astonishing communication network by which they can share their ideas.
Structure
In Chapter 4 (p. 83) we saw how the cerebral cortex can be parcelled up into the Brodmann areas on the basis of variations in the size and composition of its six layers. Conversely, it can be useful to group them together into larger functional units. In terms of gross anatomy, primate cortex is classically divided into four areas: frontal cortex (FCX) anterior to the central sulcus, temporal cortex (TCX) along the thumb of the cerebral boxing glove, occipital (OCX) at the back, and parietal (PCX) in between. In fact, the functional boundaries between PCX and OCX and TCX are not very distinct,
and many neurologists prefer to lump them all together as parieto-temporo-occipital cortex (PTO CX, or POT). What we are then left with is a binary division of cerebral cortex by the watershed of the central sulcus into just two areas, front and back. Just as in the spinal cord, where the ventral half is essentially motor and the dorsal half sensory, so – broadly speaking – everything anterior to the central sulcus is, in a deep sense, motor, everything behind it sensory. Furthermore, the posterior half is itself divided into an upper part concerned largely with localization and movement (the ‘where’ stream – see p. 158) and a lower part concerned with recognition (‘what’).
and many neurologists prefer to lump them all together as parieto-temporo-occipital cortex (PTO CX, or POT). What we are then left with is a binary division of cerebral cortex by the watershed of the central sulcus into just two areas, front and back. Just as in the spinal cord, where the ventral half is essentially motor and the dorsal half sensory, so – broadly speaking – everything anterior to the central sulcus is, in a deep sense, motor, everything behind it sensory. Furthermore, the posterior half is itself divided into an upper part concerned largely with localization and movement (the ‘where’ stream – see p. 158) and a lower part concerned with recognition (‘what’).

![]() |
![]() |
Another classic way of dividing it up is into primary sensory and primary motor areas – and the rest. As we have seen, it is this remnant, association cortex, that has grown most in the course of evolution, particularly the frontal associational area. Nowadays, more of the associational cortex tends to get called secondary or tertiary cortex, for example the large number of secondary visual areas. This diagram shows human cerebral cortex in a highly stylized, topological form, based on contiguity. It demonstrates how these associational areas seem to form bridges between primary cortex devoted to different sensory modalities, and between primary sensory and primary motor cortex.
Right in the middle is the posterior parietal cortex (Brodmann areas 5, 7, 39 and 40), and one might therefore expect it to be concerned with the coordination of information from the visual, auditory, somatosensory and motor areas which surround them; and on the whole this seems to be true. There are massive fibre bundles connecting these neighbouring cortical regions with the parietal region, and it also receives a projection from the pulvinar and lateral posterior nuclei of the thalamus. The pulvinar in turn receives sensory information from visual areas 18 and 19, and from the colliculi and lateral and medial geniculate bodies; in addition it receives the usual reciprocal fibres from the parietal cortex itself. The lateral posterior nucleus obtains its input partly from the pulvinar and partly from the (somatosensory) ventral posterolateral thalamic nucleus. Efferents from parietal cortex go to the premotor and supplementary motor areas, to the frontal eye fields, to basal ganglia (and hence to colliculus) and indirectly to the cerebellum. In addition to connections between neighbouring areas, there are also bands of fibres (fasciculi) that link distant areas, and can be big enough to see easily with the naked eye in gross dissection. Meanwhile, the corpus callosum with its staggering 100 million fibres shuttles information backwards and forwards between the two hemispheres.

![]() |
![]() |
Hence, by receiving a range of sources of sensory information and enjoying reciprocal communication with motor planning and execution, posterior parietal cortex mediates bidirectional sensorimotor communication: the posterior parietal cortex is ideally placed to provide a working model of our environment which may then guide an appropriate motor response. Indeed, patients suffering vascular lesions to the posterior parietal cortex exhibit difficulty in tasks requiring sensory guided activity such as reaching for visually or somatosensorily presented objects, clinically manifesting as apraxia (see below, p. 263). On the other hand, receiving output reports from motor areas allows one to account for any motor
activity that might influence interpretation of the sensory data reaching the posterior parietal cortex – did I walk into the chair, or did the chair walk into me? Consistent with this idea, patients with posterior parietal lesions often suffer from tactile agnosia (discussed below, p. 261), the inability to recognize objects based on purely tactile information, a feat usually accomplished by interpreting the somatosensory consequences of manipulating an object. ‘Feeling’ is, after all, a very active process.
activity that might influence interpretation of the sensory data reaching the posterior parietal cortex – did I walk into the chair, or did the chair walk into me? Consistent with this idea, patients with posterior parietal lesions often suffer from tactile agnosia (discussed below, p. 261), the inability to recognize objects based on purely tactile information, a feat usually accomplished by interpreting the somatosensory consequences of manipulating an object. ‘Feeling’ is, after all, a very active process.
Neuronal responses
The responses of units in associational cortex show progressively more specific recognition of specialized features of the outside world, especially those that are behaviourally important such as hands and faces and eyes, compared with those of primary projection areas: some visual examples were noted in Chapter 7. In addition, as might be expected from such a diversity of input, neurons in PTO cortex often show complex responses to stimulation of more than one modality. The figure shows an example from such a neuron in the monkey, responding both to visual and somatosensory stimulation. Visually, it responds best to objects close to the eye, in one quadrant; it also responds to touching the skin in a corresponding quadrant near the orbit. Sometimes one observes responses when an animal expects a stimulus even though it is not present: in a sense the ‘near the eye’ unit could be thought of one that fires in response to a very near visual target because through experience it has come to expect contact to be made with the skin after visual stimulation.
![]() |
Responses are also often greatly influenced by context and attention. For instance, many parietal units are visually driven, with receptive fields that can be mapped out; but unlike visual cells in visual cortex itself, they may or may not fire when a stimulus appears within the field, depending on whether or not the stimulus is sufficiently interesting to evoke a subsequent motor response such as an eye movement. For this reason, this area is better regarded as sensorimotor than purely sensory (if indeed such a distinction has much meaning).
A compelling example of the intimate association of ‘sensory’ and ‘motor’ areas is the coupling that has been shown between a region of the premotor area that is active in monkeys when doing precision grip with the fingers, and another area in parietal cortex that is visually driven, and only active when the monkey sees himself – or indeed another monkey or human – doing the same task. Recording from PMA, we find that even though it is a motor area, its neurons are more active when the monkey is looking at the same task being performed – even in a video – and conversely the sensory area is activated when the monkey carries out the task, even if it can’t in fact see it.
The functional importance of such ‘mirror neurons’, and their system of mutual association is twofold: partly to enable one to learn tasks by looking at them, but also to understand what someone else is doing when you see them doing something – prediction. All of this appears to be due to a rather precise set of fibre connections that links the two areas together – both ways – in a remarkably specific way. Presumably there are many such mutual systems of association forming hierarchical ladders of predictive links between stimulus and response, extending from the most basic, semi-reflex level (for instance, the links between primary somatosensory and primary motor cortex) up to the most subtle conceptual relationships, many stages removed.

![]() |
![]() |
Association
These associational connections, so obvious even with the naked eye, are the sole reason that we have a cortex at all. We saw in Chapter 4 that the basic microstructure of the cortex seems to be of columns in which direct input from subcortical structures, especially the thalamus, mingles with associational projections from the pyramidal cells of other columns, the result being sent back down again through the projection efferents. This columnar arrangement appears to apply to all areas of cortex, association and motor as well as sensory: in the section of retrosplenial association cortex overleaf, fibres
have been labelled by using local injection of tritiated leucine into a different area, frontal association cortex. It is important to emphasize that cells in any one column talk a great deal to each other but essentially turn their backs on their neighbours, like people sitting gossiping at different tables in a pub. The intracortical associational links are rather as if they keep ringing the other tables on their mobile phones to tell them the conclusions of their discussions.
have been labelled by using local injection of tritiated leucine into a different area, frontal association cortex. It is important to emphasize that cells in any one column talk a great deal to each other but essentially turn their backs on their neighbours, like people sitting gossiping at different tables in a pub. The intracortical associational links are rather as if they keep ringing the other tables on their mobile phones to tell them the conclusions of their discussions.

![]() |
![]() |
Why is this useful? Because the resultant network of links between neurons mimics the relationships between things in the outside world, what we end up with is a probabilistic model of the world in the brain, a predictive model that can be used to anticipate what is likely to happen next: when we see a knife we think of – and expect – a fork.
![]() |
But to make such models work, it is not enough to provide the largest possible number of potential connections between all the neurons representing stimuli and actions, things in the outside world that we might want to link together. We must also have a mechanism for making the strength of these connections change to reflect the associations that are actually observed in the outside world. And this is the secret of cerebral cortex: it provides a mechanism for creating physical connections between neurons that are often active simultaneously (fire together, wire together). Artificial neural networks, programmed with this rule, can be implemented in computers and are increasingly being used to perform complex tasks of pattern recognition that must by any criterion be regarded as ‘intelligent’.



From the very earliest days of experimentation on cortex by investigators such as Sherrington, it became clear that even non-associational cortex like motor cortex had extraordinary properties of plasticity, with functions rather diffusely represented and maps that could expand or contract as the result of stimulation or disuse. Simply having an arm in plaster for a few days leads to the arm ‘losing its place’ in the sensory cortex. Conversely, relatively short periods of localized stimulation (in this case, monkeys receiving tactile stimulation of their fingertips by means of a rotating textured wheel) lead to very obvious expansion of the corresponding areas of the cortical map at the expense of neighbouring areas. Cortex is a turbulent region: stimuli jostle each other in a desperately competitive way. 

![]() |
Functions of association
When Hubel and Wiesel published their amazing findings – that cells in the visual cortex code for a wealth of information about the visual world, looking for spots and edges and lines of a certain orientation, of a particular length and moving in a particular direction and so on – people thought At last we understand how the brain works! Clearly, having once seen the principle of what is sometimes called feature extraction, we could go on like that for ever. We can join line detectors together to make detectors for squares, for numbers, for letters of the Russian alphabet, for teacups and so on ad infinitum. Is there any limit to this? Is there a special cell for recognizing every single thing we see? It is not at all clear that even with the countless numbers of neurons at our disposal we could actually do something like that. For teacups, just possibly: but for porcelain teacups, for eighteenth-century French
porcelain teacups? It doesn’t sound very likely, if only because the number of cells required would soon exceed even the million million that we are provided with. So how is it done?
porcelain teacups? It doesn’t sound very likely, if only because the number of cells required would soon exceed even the million million that we are provided with. So how is it done?
![]() |
Recognition implies classification. A classification based on only one criterion is trivial to implement: it is easy for example to build a machine that will sort peas according to size before stuffing them into appropriate tins. The problems start when there is a large number of attributes to be taken into account before a stimulus can be assigned to one or another category, when they are subject to random variation, and when it is the relation between them that is the crucial factor, rather than exact correspondence with some kind of template. If the objects to be recognized are highly stereotyped, like £10 notes, it is not difficult to make a device that looks for a match between a stored ‘ideal’ bank note in the machine’s memory, and the actual specimen that is presented. But how, for instance, do we recognize that a set of objects as different as this set of A’s actually belong in the same category? It is hard to define an ‘ideal’ letter A, or say what essentially is the A-ness that all these particular examples have in common. And we recognize a rose not because it is identical to some archetypal rose but because some aspects of it are similar to other specimens that we have seen. Although in other respects – perhaps its size or its colour – it may be different, we know these aspects are irrelevant and can be ignored. Thus there are two components to recognition: one is to do with associating together those attributes of a stimulus which define what it is; the other is the filtering out of aspects of the stimulus that are irrelevant. 

![]() |
Filtering out irrelevance
A stimulus is a function not only of the object but also of really quite accidental things like how it happens to be illuminated, what angle you’re looking at it, whether it is heard through a blocked ear or felt with numbed fingers, and so on. So while it is easy to make a machine recognize banknotes fed into a slot in a fixed position and with constant illumination, real recognition means being able to do this when one is just waved briefly in front of you, or felt, crumpled, in one’s back pocket. So the job of any sensory system is to take the stimulus and filter out those aspects of it that are accidental in this sense, and leave behind those that are intrinsic to the object, or essential. This is what we mean by recognition.
People often get muddled about the difference between the stimulus – the pattern of energy falling on receptors – and the object that gave rise to that pattern in the first place. Of course it is the object that has to be recognized, not the stimulus: stimulus is, in a sense a coded version of the object that has to be decoded again. And this is the essential problem in recognition, because the same object can give rise to very different stimuli on different occasions. Objects in the real world are perceived at different times under lighting of different intensities and colours, and from different distances and directions. The stimulus is a coded version of the object that gave rise to it, some aspects being essential, and due to the object itself, and some being merely accidental, and nothing to do with the object at all. A particular retinal image of a cube under particular conditions is as much a coded version of the cube, that has to be deciphered, as are the four letters CUBE: in many ways the latter presents an easier task. So the job of the visual system (and indeed any sensory system) is to separate off those aspects of an object’s image which are its essential attributes in the sense of defining
its essence, and those which are merely accidental and the result of temporary circumstances.
its essence, and those which are merely accidental and the result of temporary circumstances.
![]() |
This mechanism of filtering out is something we have already met when considering the functions of adaptation, more particularly in the eye. We saw that one of the functions of dark adaptation is to enable us to perceive the intrinsic albedo of an object despite the fact that on different occasions it may be brightly or dimly illuminated. It may seem strange that whether something is perceived as white or black should apparently bear so little relation to how much light the eye gets from it, but if you think about it for a moment you can see that it is exactly what is needed in order to recognize objects. What the brain has to do is to make allowance for different levels of illumination, so you actually perceive albedo rather than luminance. Albedo is what tells you what sort of object it is – a snowball or a lump of coal – whereas luminance is messed up with how brightly the sun happens to be shining, quite irrelevant to deciding what you are looking at. By filtering out the accidental aspect of the stimulus – illumination – we are left with the essential property, albedo, which is characteristic of what we’re trying to recognize.
It is not hard to extend this idea – of discarding accidental properties to leave behind what is most characteristic of an object – to much higher levels of perception. When we look at a coin on a table, it looks circular even though its retinal image is in fact an ellipse. It is easy to see how a mechanism of associative memory will do it: as we move our head, different ellipses tend to occur together and the corresponding neurons get wired together; as a result, we are filtering out the accidental aspects of the stimulus that depend on us and not on the coin. Or again, when we look at that set of As, we are filtering out the accident of the way in which they happen to have been designed. At a higher level, we recognize that arbre, baum and tree are all essentially the same by disregarding the accident of what language they happen to have been expressed in. The same principle operates at the very highest levels of thought: in chess, rather than laboriously calculating all the possible consequences of a given move, a good player will perceive that the position he is faced with, though never experienced before, is in some essential way the same as one he knows how to win. Intelligence in fact is the ability to discern an underlying similarity between things when they are obscured by irrelevant detail.
![]() |
Thus filtering, classification, recognition and association are simply different aspects of the same underlying phenomenon. Our brains are not genetically programmed to recognize lines and faces and letters of the alphabet and so on: they get wired up through experience. We recognize a figure ‘3’ because it has certain topological features that are found in association together: a single continuous line with a cusp in the middle to the left and a couple of bulges to the right. If we imagine individual neurons that respond to each of these features, we can see in general terms how, with sufficient repetition, they would tend to strengthen their mutual connections and form a functional cluster corresponding to the existence of 3s in the outside world.
Learning to classify
Consider, for example, line-detectors in the visual cortex discovered by Hubel and Wiesel, which are essentially created by wiring together retinal ganglion cells that lie in a row. Experiments have shown that even these relatively simple detectors require the animal to have actually experienced lines for them to wire themselves up properly. If, for example, you rear kittens from birth in such a way that they never experience horizontal lines, then one finds on testing their cortical units that the great majority of them respond only to vertical or near-vertical lines and not to horizontal lines at all. It seems therefore that the stimuli themselves wire the units up.
![]() |
It is not difficult to think of a plausible mechanism by which this could happen. Imagine a ‘naive’ or untrained cortical cell, that starts with quite random retinal connections as shown in the figure. If we then present a line at a particular orientation to it, it is clear that though the field is initially random, nevertheless the line may well stimulate it enough to make it fire. If now we make the usual Hebbian assumption, that those synapses where the pre- and postsynaptic cells are active simultaneously get strengthened, you can see that the useful inputs to that cell will get stronger and the others presumably relatively weaker, so that eventually it builds its own linear receptive field, and is also capable of responding to incomplete stimuli.
(Such a model can be extended to cover the generation of inhibitory surrounds as well; but
it must be said that recent work has indicated that the true mechanism, though not understood in full, is not quite as simple as the one presented here.)

it must be said that recent work has indicated that the true mechanism, though not understood in full, is not quite as simple as the one presented here.)
![]() |
Extending such a notion to yet higher stages of cortical processing, we can easily imagine units that could learn in exactly the same way to respond to the more complex sets of essential features that make up things like teacups and human faces. It is certain that some such mechanism of learned connections must exist, for we know that the cortical units of young kittens brought up in a visual environment consisting entirely of lines having a single orientation will on subsequent testing respond only to lines of that same orientation. Once such a set of features have been associated together in this way, the detector may not mind very much if some of its inputs are missing on a particular occasion: so long as it fires more actively than any of its neighbours in response to a particular object, then it will in effect form a hypothesis about what is present. We not only recognize the A, but are convinced we can actually see phantom contours that we expect but are not actually there. In this way we can deal with situations where – as is almost always the case in real life – parts of objects are obscured because they are hidden behind other objects. So our perceptions are conditioned all the time by our expectations, and in fact the cortex’s job is to be a sort of Sherlock Holmes, jumping to conclusions on minimal evidence: having recognized, we perceive associated elements we expect to see but not actually present in the stimulus at all. Decision-making columns, receiving fragmentary clues from the outside world, but also exchanging gossip with each other through their associational fibres, provide an ideal mechanism for doing this. The figure below shows how this could operate in a particular case. An ambiguous letter (A? H?) is perceived as A because of the predictions from neighbouring columns that respond to part of the local context.
![]() |
![]() |
![]() |
Recent work has revealed something of the dynamic properties of associative networks of this kind. Many individual units all over the cortex and in some subcortical regions show electrical activity that appears to rise steadily before an action is about to be performed, collapsing again once the movement actually starts. A well-studied example is the saccade. In the colliculus, as well as in the frontal eye fields and posterior parietal cortex and elsewhere, there are units whose firing starts to accelerate as long as two or three hundred milliseconds in advance of a saccade made to fixate a visual target, and similar early responses can be seen in the electroencephalogram (EEG) long before other kinds of movements. When this build-up of activity reaches a particular level, it appears to trigger the movement itself, and variation in the rate of rise from trial to trial seems to be reflected in variation
in reaction time. (These mechanisms of decision are discussed further in Chapter 14, p. 282). It is not difficult to see how this kind of behaviour might arise through associative circuits, with information from the stimulus being combined with associative links representing the probability of the target existing (given the context), to form a crescendo of agreement between widely scattered units that nevertheless act in synchrony and finally decide to initiate action. The parallels with how decisions are reached in human organizations are obvious: a recent example that comes to mind is the crescendo of Facebook activity among would-be protestors in Egypt in 2011 before the joint occupation of central Cairo.
in reaction time. (These mechanisms of decision are discussed further in Chapter 14, p. 282). It is not difficult to see how this kind of behaviour might arise through associative circuits, with information from the stimulus being combined with associative links representing the probability of the target existing (given the context), to form a crescendo of agreement between widely scattered units that nevertheless act in synchrony and finally decide to initiate action. The parallels with how decisions are reached in human organizations are obvious: a recent example that comes to mind is the crescendo of Facebook activity among would-be protestors in Egypt in 2011 before the joint occupation of central Cairo.

![]() |
Lateral inhibition
Lateral inhibition is an important component of models of this kind. It plays an important role in discarding accidental information when distinguishing the aspects of visual, auditory or tactile stimuli that allow recognition; but it also plays a part in association. As we saw when discussing the process by which line-detectors might be generated, during the learning process it will ensure that only the cells that are most stimulated by a particular pattern will be activated enough to increase the strength of their afferent synapses. And subsequently it will help to sharpen up the discrimination between stimuli that differ only slightly by enhancing any differences in the patterns of neural activity that they evoke. Lateral inhibition of this kind is not strictly spatial, of the kind introduced in Chapter 4, but operates rather along what might be called an abstract sensory dimension. For example, the mutual inhibition between red- and green-sensitive channels in the retina that generates colour-opponent responses can be thought of as lateral inhibition along a wavelength axis, that sharpens up colour discriminations. In the same way, lateral inhibition between line detectors in the visual cortex acts along a dimension of orientation, improving angle discrimination. A consequence of this is that if two lines are presented at once, forming an angle, the effect of the lateral inhibition is to exaggerate the difference in their orientation, and thus make the angle seem larger. Many well-known optical illusions can be explained by angle-expansion of this kind.
In the Zöllner illusion, above, the thick zigzags twist the apparent orientation of the thinner lines away from their true orientation. The same mechanism is at work in the ‘café wall’ illusion, below. In the same way, the square (below left) becomes distorted in shape because of the tilted lines behind it, and on the right (the Poggendorf illusion) angle expansion produces the strong impression that the two parts of the oblique line will not in fact line up. While these illusions appear merely to distort our interpretation of the visual environment, in less artificial
circumstances the same mechanisms serve to exaggerate differences and facilitate distinction between objects.

circumstances the same mechanisms serve to exaggerate differences and facilitate distinction between objects.
![]() |
![]() |
![]() |
The most abstract example of all is perhaps in the olfactory bulb.
We saw in Chapter 8 that olfactory receptors are very unspecific as to the chemical stimuli they respond to: to take a simplified example, while one receptor might respond to substances A, B, C and D, its neighbour might respond to A, B, C and E. But the effect of lateral inhibition between second-order neurons within the olfactory bulb will be to eliminate the overlap between the two ‘receptive fields’ and thus considerably sharpen up their modality specificity to particular stimuli – X now responds only to D and Y only to E. Here we have lateral inhibition that is in effect operating in a multidimensional stimulus space.

![]() |
The final goal of recognition is not of course simply the identification of individual objects, but of attaching meaning to them. This implies, in effect, associating them not only with each other, but with words, actions, and above all with emotional states and with the satisfaction of physiological needs. The only way to make sense of what the brain does is to take a firmly pragmatic line, to insist all the time on asking what use things are. Our senses are not after all merely there to provide some sort of in-flight entertainment for the soul: they have evolved because they help us to survive. They are required by the motor system both in the planning and execution of actions, and also by the motivational systems that decide what action to take: whether an object is nice or nasty, whether it is something to eat, or something that will eat us. It is not difficult to see how this can be done, by an extension of the mechanism for forming associations by means of synaptic strengthening, and this is what the next section is about.
Neural mechanisms of association

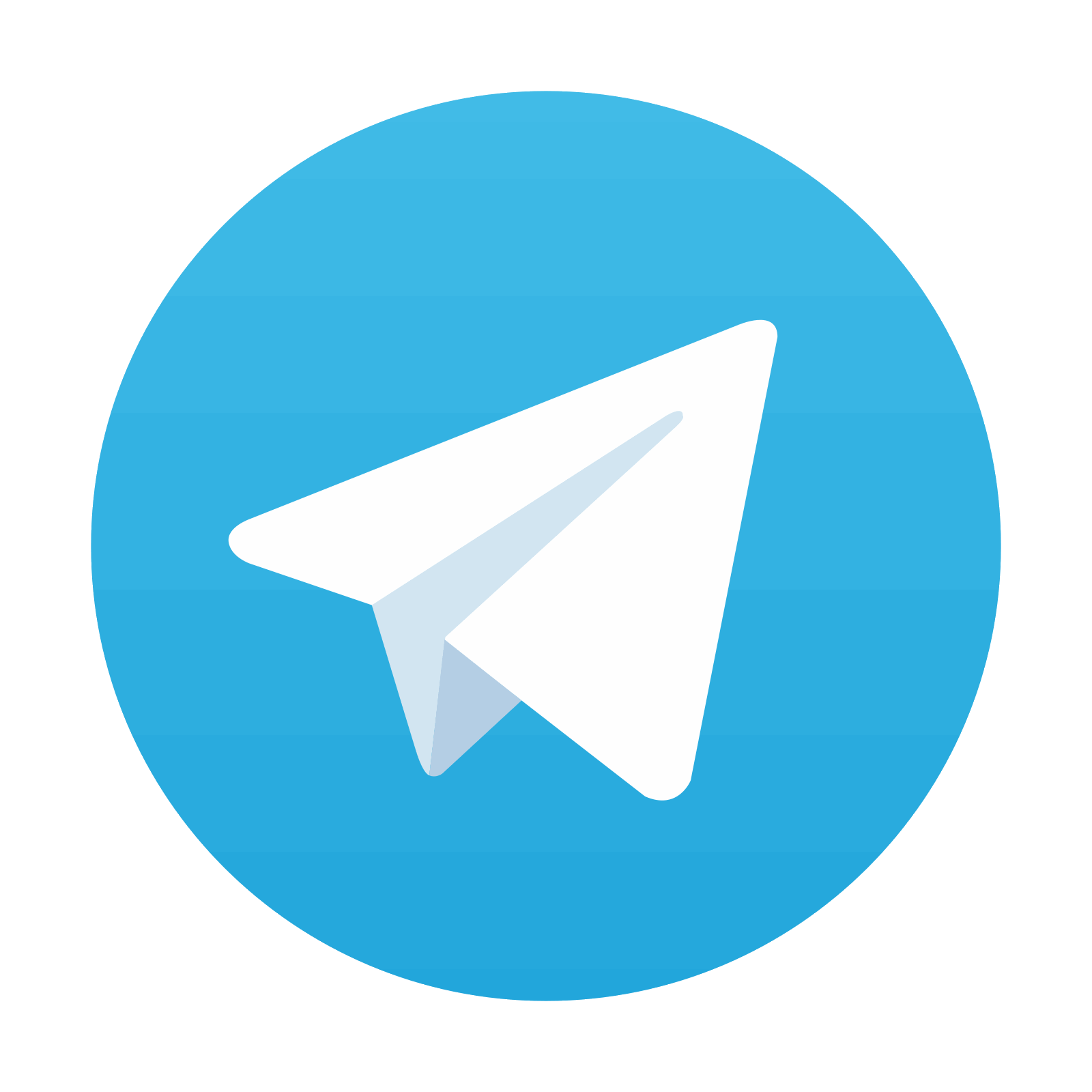
Stay updated, free articles. Join our Telegram channel

Full access? Get Clinical Tree
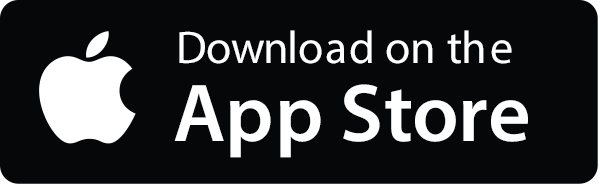

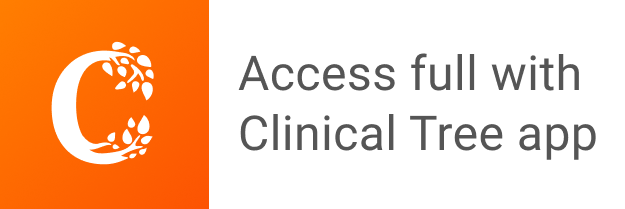