Abstract
Surgeons, neurologists, researchers, and patients have lacked the technology-based tools to facilitate sharing the tremendously valuable data about patients’ treatment and research in regard to what is working and what is not. Today, only 9% of patients who could benefit from complex therapies to address neurologic conditions actually receive them, and the medical information for each patient who does is hidden away in disconnected databases. To optimize and accelerate our understanding of the brain, we need to gather intelligence around every case, every research subject, every study while connecting that information through a unified, Health Insurance Portability and Accountability Act of 1996 (HIPAA)-compliant network that leverages technology and harnesses the Internet to drive advancements and better connect patients to their care teams. In this chapter, we highlight the key aspects needed to fulfill the requirements of a robust, HIPAA-compliant archive for brain data and highlight the impact of normalization on the accuracy of statistical analyses.
Keywords
Atlas, Big data, Collaborative, CranialCloud, DBS, Normalization
Outline
Neurodegenerative Diseases Are Common Diseases With Significant Public Health Burden 138
Neurological Data Are Complex 139
Patient-Centric and Personal Health Information 139
Integration With Clinical Workflow 140
Assuring Data Quality and Completeness 141
Integration of Spatial and Temporal Data 141
Ready for Sensing and Clinical Surveillance Data 141
Respecting the Privacy and Ethical Implications of Neuroscience Research 141
Security From the Ground Up 141
Fostering Data Sharing 142
Data Normalization 142
Custom Patient-Based Medicine 143
Conclusion 144
Acknowledgments [CR]
Disclosure [CR]
References 144
Further Reading 145
Acknowledgments
This concepts and work presented in this chapter have been supported by the NIH through 3 R01 (1R01-EB006136, 2R01-EB006136, and 9R01-NS095291) grants and performed in close collaboration with the following partnering research institutions: Ohio State University (Dr. Ali Rezai, MD), the VA in Richmond (Dr. Kathryn Holloway, MD), Wake Forest Medical Center (Dr. Stephen Tatter), and Thomas Jefferson University (Dr. Ashwini Sharan, MD). Phase I (R41NS063705) and a phase II (9R42MH10000) STTR grants have supported the clinical/commercial translation.
Disclosure
Drs. D’Haese, Dawant, and Konrad are co-founder and shareholders of Neurotargeting, LLC. Neurotargeting has acquired some of the technology described in this chapter and developed at Vanderbilt University. The role of Neurotargeting has been to handle the regulatory issues related to the transformation of a research system into one that can be used clinically and integrated into the clinical flow at multiple institutions. The original system developed at Vanderbilt is called CranialVault its latest version is called CranialCloud.
Our healthcare ecosystem continues to evolve with three major forces at work: improving the quality of care, driving down the cost of care, and enhancing engagement and the patient relationship. Those forces are transforming health care into an “information-driven,” “evidence-based,” and “outcome-driven” model. In an era of open information, the big data revolution is in its early days in health care, and most of the potential value for creation is still unrealized. Health care has experienced a decade of digitizing medical record and aggregate years of research and development data in electronic databases. The government and hospital managers have also accelerated the move toward transparency and more security, allowing modern technologies to enter the premises of the medical centers. Innovative companies in the private sector are building application and analytical tools to help patients, physicians, and other stakeholders to identify value and opportunities. But the fuzziness of the big data concept and the complexity of data involved in patient care have slowed down the progress toward affecting health care. Business analysts predict the Healthcare Analytics Market will be worth US$24.55 billion by 2021. Clinical data are stored in electronic medical records (EMRs). While EMR systems provide a centralized electronic system that will archive evidence of care for each patient, these solutions have not been designed to structure data in a way that could be harvested and analyzed by modern technologies, build knowledge, and provide predictive medicine. The lack of major big data companies in that field shows how difficult it is to “plug into” an EMR system and exploit its data for population-based analyses.
Pharmaceutical and some life science companies have been able to create value by extracting data from EMR systems. For instance, Flatiron, Inc., a Google-funded, New York–based company, has set up a model to extract from EMRs the patient’s diagnosis, patient’s therapies, and their effect on treating the patient’s cancer. Flatiron leverages modern cloud-based technologies to connect a large number of medical centers to harvest data on a large number of patients. Researchers can mine the data to see what treatments are most effective for particular conditions, identify patterns, and gain knowledge to improve patient care and reduce costs. The pharmaceutical industry could indeed benefit from this revolution. The conclusion is not so clear for other therapies. If it is true that EMR data are exploitable for learning how therapies affect patients suffering from cancer, the data involved in treating disorders of the central nervous system (CNS) ranging from Parkinson to Alzheimer disease, to epilepsy or pain are more complex and not typically stored in EMRs. The reasons are manifold: the data cannot be easily extracted from the clinical flow and therefore are not typically stored in EMR systems, and when the data are acquired, EMR systems are not designed to store complex data; and finally, the creation of mathematical and statistical models requires a deep understanding of the therapies and data they generate, which are skills that are difficult to find in the industry.
In addition, the challenge is not limited to the flow and storage of data within one medical center. In order to provide access to data that will allow the creation of useful knowledge, there is a need for data standards and systems that could be used across medical centers. In neuromodulation, surgeons, neurologists, researchers, and patients have lacked the technology-based tools to facilitate sharing the tremendously valuable data about a patient’s treatment and information from patients regarding what therapies are working and what are not. The lack of solutions for information sharing has pushed physicians and researchers to create home-grown and disconnected databases. In order for big data initiatives in neuromodulation to succeed and accelerate our understanding of the brain, there is a need for a professional-grade system built on modern technologies that will complement EMRs to gather intelligence around every case, every research subject, and every study while connecting clinicians and researchers through a unified, Health Insurance Portability and Accountability Act of 1996 (HIPAA)-compliant, independent network.
Neurodegenerative Diseases Are Common Diseases With Significant Public Health Burden
Because neurodegenerative diseases strike primarily in mid to late life, the incidence is expected to soar as the population ages. By 2030, as many as one in five Americans will be over the age of 65. According to the World Health Organization, if left unchecked, 15 years from now more than 12 million Americans will suffer from neurologic diseases. The urgency of finding treatments and cures for neurodegenerative diseases is thus increasing. Neurodegenerative diseases require solutions that involve a broad range of expertise encompassing such different fields as neurology, genetics, brain imaging, drug discovery, electrophysiology, stereotactic neurosurgery, and computer science, all of which generate large data sets related to very small targets within the brain. Responding to the need to collect and share data, a number of research tools have been developed. The most successful examples focus on specific data types. In 2003, we have created a collaborative system called CranialVault ( ) focusing on deep brain stimulation (DBS); in 2005, offered to the community the XNAT system, which has a primary objective to support the sharing of clinical images and associated data. This is a goal shared by other successful initiatives such as NiDB ( ), LORIS ( ), or COINS ( ). The Alzheimer’s Disease Neuroimaging Initiative (ADNI) unites researchers with study data as they work to define the progression of Alzheimer disease ( ); focuses on sharing cell data, on cortical connectivity, and Van Horn on functional magnetic resonance imaging (MRI) ( ), to give only a few examples. Another example is the Collaborative Research in Computational Neuroscience (CRCNS) program funded by the U.S. National Institutes of Health (NIH) and National Science Foundation that focuses on supporting data sharing. The CRCNS provides a resource for sharing a wide variety of experimental data that are publicly available ( ). In parallel, tools to support structured heterogeneous data acquisition within or across institutions have been developed, such as the REDCap ( ) system, which is already in use for more than 100,000 projects with more than 150,000 users spanning numerous research focus areas across the REDCap consortium.
Neurodegenerative diseases such as the Parkinson disease, epilepsy, or Alzheimer disease have been studied through the prism of neuromodulation. As a result of pioneering work by Drs. Benabid and DeLong, DBS is now a primary surgical technique that reduces tremors and restores motor function in patients with advanced Parkinson disease, dystonia, and essential tremor. Much of the future potential in precisely focused therapy such as DBS has to do with understanding the exact location of delivered therapy and its impact on circuitry at a millimetric level of precision. DBS is one of the only minimally invasive therapies that allows for capture of complex data and relates it to an accurate location inside the patient’s brain. During these procedures, single neuron activity is often recorded; no other therapy provides such a fine access to data. DBS is therefore a chance for the clinical research community to study the electrophysiologic brain mechanisms. However, surgeons and neurologists have to limit the amount of data acquired during each operation because of time. To permit the creation of models, data should be aggregated across patient populations. Precise colocalization of population data in millimetric anatomical targets for DBS therapy has an enormous impact on our ability to create models and study accurately DBS mechanisms. The variation in human brain anatomy is large enough that a simple aggregation of data without accurate corepresentation within a common space leads to vague generalization and comparisons of treatment modalities and diagnostic capabilities ( ). While the impact of aggregation mechanisms will be discussed in further detail in this chapter, Fig. 13.2 illustrates the effect by comparing current and modern techniques used to aggregate data.
Neurological Data Are Complex
The lack of data analysis with the level of precision needed is a result of the complexity and heterogeneous nature of the data acquired during neuromodulation procedures. A system that can store and share data efficiently should be able to connect imaging data sets to electrophysiologic neuronal signals, patients’ responses to stimulation to disease progression and follow-up scores, amount of medications taken by the patients to neuromodulation parameters, and quality of life measures to clinical care. The absence of widespread standards for data acquisition and processing further complicates the task.
During the past 15 years at Vanderbilt University, a system that addresses these challenges has been under development at our institution. In the remainder of this chapter, we report what has been learned from this experience and its evolution from a single-site data repository to a system that can be integrated into the clinical workflow and that permits sharing of data across multiple sites. The original system was called CranialVault. Its newer version is called CranialCloud to reflect the fact that it has been implemented by using a more modern, cloud-based technology. Because acquiring data at the time and point of care requires integrating systems in the clinical workflow, these systems need to meet regulatory requirements. Academic institutions are ill equipped to handle processes associated with regulatory clearance, so a separate entity called Neurotargeting, LLC was created. This entity licenses the technology developed at Vanderbilt University and has been responsible for maturing the research system to a clinical system. Drs. D’Haese, Konrad, and Dawant are equity holders in this entity.
As discussed earlier, there is a need for a patient-centric system that can be integrated into the clinical flow to permit continuous data acquisition across phases of the therapy as well as collection of data from advanced and sometimes emergent research protocols. With the technologies that are currently available, our goal is to develop a system that would work as easily as a Dropbox system but is appropriately secure for storing valuable patient data. This system should not only allow clinicians and researchers to build interdisciplinary collaborations but also enable researchers to create a collaborative network without breaching any legal regulation; it should be able to connect with existing devices and data acquisition systems as well as with other existing archives; it should allow experts in data analytics to plug-in their algorithms for expert analyses; it should enable research while considering ethical implications; and it should facilitate the dissemination of techniques and methods developed by various groups to process and analyze the data.
Our first system relied on an SQL-based architecture ( ) to store any DBS-related data. This system permitted the collection of data without disturbing the clinical flow, and it included a processing pipeline used to normalize ( ) the data acquired from each patient onto a common reference system, called the atlas. The first CranialVault system was centralizing data at Vanderbilt University. While the data were easier to manage and share across groups with this type of architecture, difficulties arise over data ownership, cross-institutional data-sharing issues (e.g., obtaining multiple institutional review board (IRB) approval for data sharing across universities), as well as the permanence of a resource supported mainly by investigator-initiated federal grants at one institution. One possible solution to these issues is to rely on a distributed database approach (i.e., deploy a set of individual databases that are connected to each other). While this approach is commonly used in the industry, it necessitates both local and global management teams working on the same system. The local servers have to be maintained, while the global group has to manage interconnection between groups. A better approach that has become available in the recent past is to rely on cloud-based solutions. In this approach, the system consists in a set of interconnected nodes hosted in the cloud with each institution/group owning its own account. However, cloud-based systems such as the one we envision need to fulfill a series of the requirements to both offer the functionality that is needed and be HIPAA compliant. In the next subsections, we detail the main requirements these systems need to fulfill and, based on our experience, some pitfalls that ought to be avoided.
Patient-Centric and Personal Health Information
Because of legal and security requirements, many existing archives have chosen to ban personal health information (PHI) from their system. This approach allows easier data management, storage, and transfer without the security burden required to meet the Office for Civil Rights (OCR) and HIPAA regulations present in U.S. policy. However, working in a completely anonymized fashion can become a limiting factor when dealing with longitudinal data sets from patients/subjects who are followed for a long time or across multiple institutions. While longitudinal studies have certainly be done with deidentified patients, these have to be planned accordingly to track each patient in time. Moreover, an anonymized system complicates integration in the clinical flow.
Integration With Clinical Workflow
Data acquisition that is easy to use, automated, and nondisruptive is key for an archive that focuses on complex and large data sets such as those involved in the BRAIN initiative. It is imperative for the archive to be connected to the clinical tools that generate the data either directly or through dedicated interfaces. This requires the designer to be intimately familiar with the type of data that are acquired as well as with the data acquisition flow ( Fig. 13.1 ).
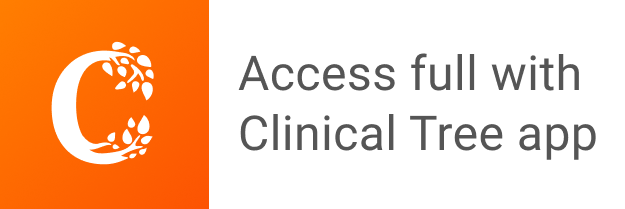