Introduction
S.P. is a 60-year-old female with a history of hypertension who presented comatose with a left thalamic intracerebral hemorrhage associated with intraventricular hemorrhage, midline shift, and localized mass effect. Emergently, an external ventricular drain was placed into the left frontal horn, and on day 5 an additional drain was placed into the left temporal horn for persistent left temporal dilation. Invasive multimodality monitoring including intracranial pressure (ICP), and brain tissue oxygen tension (P bt O 2 ), and cerebral microdialysis was placed into the right frontal lobe. She received repeated doses of mannitol and hypertonic saline for persistent elevated ICP.
Managing patients with intracranial hypertension, like S.P., is a mainstay in neurocritical care and involves a structured treatment protocol to continually assess the effect of each intervention. Tracking and quantifying physiologic measures such as cerebral perfusion pressure (CPP) also is crucial and needs to be put into the context of sedation level, osmotherapies, ventilator settings, and temperature modulation among many other interventions. In a digital world clinical staff should be able to systematically, continually, and rapidly evaluate the effect of various treatments for increased ICP. For instance, when the impact of mannitol and hypertonic saline administration in S.P. is evaluated, the neurocritical care unit (NCCU) staff should have immediate access to basic variables such as osmolality and ICP at the bedside ( Fig. 4.1 ). As fundamental as this is, a plot that contains these core elements is difficult to create in real time and even retrospectively at most institutions. But imagine being able to look at the patient’s display screen in the NCCU and to be able to answer the following questions easily:
- 1.
When mannitol is administered, by how much (mm Hg) is ICP lowered; how quickly is ICP lowered, and how long until ICP again increases to greater than 20 mm Hg?
- 2.
Are repeated administrations of mannitol equally effective or does repeated administration fail to lower ICP as much, as quickly, or as long?
- 3.
Does adding hypertonic saline provide additional ICP reduction?
- 4.
Do osmolar data suggest that additional osmotic therapy would be dangerous to the patient?

Today clinicians generally are required to answer these questions in their heads, approximating when interventions were made by thumbing through the hourly recorded data, estimating each therapy’s impact on ICP, and manually checking laboratory data to estimate the osmolar gap. Ideally other aspects of ICP management protocols also should be evaluated, for example, end-tidal carbon dioxide (CO 2 ) and temperature. Further, analysis tools at the bedside should exist to evaluate the patient’s physiologic status, such as cerebral autoregulation, which would indicate how ICP would respond to changes in blood pressure. This then may reduce the need to discuss how other invasive neuromonitoring data (e.g., cerebral blood flow, P bt O 2 , cerebral metabolism) may facilitate ICP management. Any attempt to understand these dynamic relationships among ICP, interventions, and other physiology is complicated, and yet this is only one aspect of patient care.
The promise of clinical information systems is to improve patient care, reduce medical errors, reduce documentation time, and improve efficiency. Paper-based hospital medical records are generally inefficient (e.g., illegible handwriting, manual data entry) and have been shown to contribute to medical errors. Billions of dollars are being devoted to the development and implementation of electronic health records (EHRs) and significant progress has been made to bring these data together for documentation purposes. Intensive care units (ICUs) have been migrating from paper-based to computerized charting systems for more than a decade.
In the ICU, however, problems that face clinicians and nurses extend beyond the need for more efficient documentation. During rounds of critically ill patients each morning, a physician may be confronted with more than 200 variables, and some clinical information systems acquire and store physiologic variables and device parameters online at least every minute. People, however, are not able to judge the degree of relatedness between more than two variables. The ability to store high-dimensional data far exceeds the intellectual capacity to understand it unassisted ; this greatly contributes to conditions of constant “information overload” that can lead to preventable medical errors. A clinical informatics infrastructure in the NCCU that only supports documentation is simply not sufficient. Ideally NCCU staff should be able to use clinical decision support systems to help understand what a patient’s data is trying to tell them.
Currently there is a paucity of clinical decision support systems in use in the ICU, but this is understandable because the data and infrastructure to support their development and use are generally not in place. When patient data is collected in digital form, it usually resides on isolated systems without a way to bring this data together in meaningful ways. The objective of this chapter is to provide a blueprint for work with hospital administration, information technology, biomedical engineering, industry vendors, and clinical staff to create an informatics infrastructure that provides decision support. Many hospitals already have data-collection systems in place for electronic documentation, laboratory results, and physician-order systems but need strategies to integrate these data with high-resolution bedside monitor data to support clinical decision support systems. First, clinicians need to be able to articulate a clear message as to why patient data must be stored at a high resolution and be accessible in real time to support clinical decision making.
The Justification: Why Clinical Data Is Needed
Data are a commodity that has many purposes but in health care enable hospitals to provide medical care and meet associated legal obligations. Patient data must be maintained securely over a long period of time and done so in a way that maintains patient privacy. Storing data digitally can be more efficient, help reduce medical errors, improve medical device management, and reduce health care costs. It is important for clinicians and nursing staff to voice how patient data can be used to improve patient care. In the absence of that, other more mundane administrative concerns usually take precedence. In the NCCU the clinical need for real-time access to patient data and clinical decision support systems falls into three categories. First, NCCU staff need tools to evaluate the effectiveness of treatment(s) meant to modify physiologic endpoints or improve clinical conditions such as cerebral vasospasm or sepsis. Second, patient data should provide understanding of the patient’s physiologic status (e.g., dysautonomia, cerebral autoregulation failure), and how such physiology impacts other metrics of brain health (see Chapter 40 ). Third, complex relationships within patient data should be automatically processed to enable better understanding of prognosis and earlier diagnosis of secondary events before clinical symptoms occur. This automatic processing of data further supports clinical decision making, and can enable computerized implementation of clinical protocols (see Chapter 45 ) or decision making on prognosis or outcome prediction if appropriate models are used.
Clinical Computing Systems
The Blueprint
The goal is to create the informatics infrastructure necessary to enable health care providers to leverage data from database systems that contain physiologic, laboratory, pharmacy, and other clinical data for the continued development and implementation of better clinical support tools to provide patients the best care possible. There are many ways to accomplish these goals, but it is essential to work closely with the institution’s information technology (IT) department to devise the right solution for the ICU and institution because it is often difficult to tell what is needed before the project starts. Regardless of the specifics of the solution, each will contain the same basic elements: (1) collection and storage of different kinds of patient data to a database system; (2) creation of a data warehouse whereby patient data are integrated into a single database with copies of all databases to support real-time analytics; and (3) use of software to perform various methods of analysis that together create a clinical decision support system ( Fig. 4.2 ). Chapter 40 , Chapter 45 describe some NCCU specific solutions that have been implemented.

Planning
Informatics is a rapidly changing in field, and therefore a department should not be locked in any one system at an infrastructure level. Data should be stored in an open nonproprietary format such as Structured Query Language (SQL) (Open Database Connectivity [ODBC] compliant) database that will allow any system to access the data. Many ICU directors strive to include an informatics infrastructure when building a new ICU. This is a great time to do this because there will be a capital budget, and essential items such as installing power and internet connectivity in patient rooms will be cheapest during the construction phase. An ICU construction project also will mean that health care providers will have the attention of hospital administrators and information technology personnel. It also is vital to create an operating budget to maintain the infrastructure over time and to upgrade equipment and to decide whether remote data viewing is important to put such a system in place. There are commercial products, such as Citrix MetaFrame, that securely enable this functionality and are gaining favor in ICU and emergency department (ED) settings. Decisions also should be made about whether to integrate the NCCU data with other ICUs, both in the same institution and other medical centers. Creation of such integrative databases may help facilitate syndrome surveillance, decision support, comparative effectiveness research, and outcome research. Data collection systems and the EHR, however, do not replace verbal communication for efficient interdisciplinary exchange of patient information in the NCCU but can be used to supplement this.
Collecting Bedside Device Data
Overview
Current EHR systems generally are adequate to store clinical documentation and laboratory, pathology, and radiology information. However, most EHRs are not designed to capture and store high-frequency physiologic measurements obtained from patient monitoring equipment. In the NCCU, invasive and noninvasive monitoring techniques provide continuous measurement of physiologic parameters, and this high-frequency data can be vital to understand the course of critical illness and optimize treatment. Data documented in an EHR by the NCCU staff, typically on a hourly basis, are not sufficient to fully represent this physiologic data stream, and therefore it is desirable to establish a thoughtful strategy to collect and use data from bedside medical devices such as ventilators, patient monitors, infusion pumps, and other neurospecific monitors. Bedside medical device data are generally the most difficult data to obtain, whereas continuous electroencephalographic and associated patient video data create the greatest demands on network and storage capacity. The informatics infrastructure needed to collect and store continuous electroencephalographic data in the ICU environment is described in detail elsewhere.
Collecting Bedside Patient Monitor Data Via a Network Portal
Many bedside medical devices are plugged into the standard patient monitor, which serves to display all the digital and waveform data on a single bedside display in real time. Most clinical information systems from patient monitor vendors store these data up to 72 hours for clinical review purposes before they are deleted forever. Some may transfer hourly values into an EHR for nurse verification. Networked patient monitors facilitate patient data display in areas other than the patient’s room, such as a central station or the nursing pod area, by allowing other systems to receive that data from a central server. Networked systems represent one potential strategy to collect all data on every bedside patient monitor from a single networked portal at a high temporal resolution.
Some medical device manufacturers, including Philips Healthcare (Andover, MA) and GE Healthcare (Chalfont St. Giles, UK), have developed their own proprietary solutions for automated data acquisition through this single portal. Customers rarely use these solutions because they tend to be expensive, do not provide adequate data frequency, and sometimes do not integrate easily with other clinical information systems. These systems do continue to improve, however, and it is sensible to investigate data collection and storage solutions from the institution’s patient monitor vendor. Third-party products such as DataCaptor (Capsule Technologie, Paris, France) are designed to convert the nonstandard output from bedside devices into HL7 format, which can then be sent to EHR systems such as Cerner Millennium (Cerner Corporation, Kansas City, MO) and Eclipsys Sunrise Clinical Manager (Eclipsys Corporation, Atlanta, GA). Open-source efforts to collect bedside device data for both research and clinical care purposes is described elsewhere. In the Columbia University Medical Center neurological ICU, Bedmaster XA (Excel Medical Electronics, Jupiter, FL) is used to collect all the bedside patient monitor data including parameter data at least every 5 seconds and all visible waveform data at a resolution of 240 Hz. This system works for both the General Electric and Philips patient monitors.
Collecting Data from Individual Bedside Patient Monitors or Devices
It may not always be possible to collect bedside device data through a network portal, and many medical devices do not plug into the patient monitor, or if a device does plug into the monitor, not all the data are transferred. Data then need to be collected from the individual medical device or sometimes even each individual patient monitor. Most bedside devices (including the patient monitor) are equipped with RS-232 (digital) and analog (waveform) data output ports for automatic data output capabilities. Specifications for data communication are generally available in the device’s technical manual or can be obtained from the device manufacturer.
Medical devices output data usually as a comma-delimited text string (e.g., 21, 15.6, 78.24, 12, 15) every few seconds. This text string must be converted into data that fill specific fields in a database, which requires knowledge about what each number in the text string represents and in what field in the database it should be stored. This translation is referred to as a device interface (a small bit of software that automatically converts data from the device into sensible data for a particular database). Provided it is known what data output is sent from the machine, what each number means, and where it goes in the database, actually writing a device interface is not complicated.
One limitation of RS-232 for bedside device communication is that there are many different ways to send data over the serial interface. Several different pin-out and connector styles exist, and there are multiple options for baud rate, parity, stop bits, handshaking, flow control, and signaling. For example, many bedside devices output the data string at a set time frequency without prompting, whereas with other devices, such as the patient bedside monitor, the software interface must send a coded request to receive data. Therefore the device interface must be written to accommodate the specifics of each device or patient monitor configuration. Some newer modes to output data from devices include Universal Serial Bus (USB), 802. (Ethernet), IEEE 11073, or even wireless (e.g., 802.11 b/g, Bluetooth) data communication capabilities. Because the life span for some medical devices is 10 to 15 years, it is unlikely that RS-232 ports will disappear in the near future.
At Columbia University it was decided to use Bedmaster XA to collect bedside data in the NCCU, because the company also wrote device interfaces for devices that did not connect to the bedside monitor, or did so incompletely. For example, it is possible to collect all 22 parameters outputted from the Arctic Sun cooling device (Medivance, Louisville, CO). This allows caregivers to automatically track the device’s water temperature, which decreases when the machine works harder to maintain body temperature (i.e., fever spike), and other parameters such as ICP and P bt O 2 . There also is a device interface for the Camino ICP monitor (Integra Neuroscience, Paramus, NJ), even though this monitor can plug into the patient bedside monitor. Many brain injury patients may have an external ventricular drain and a parenchymal ICP monitor. It is important to distinguish each ICP from the other. However, there are limited methods to maintain the same data label for each device regardless of where it was plugged in on the patient monitor. Instead the Camino is now to both the patient monitor and the secondary system, where plugged in the data label can be controlled. It is important to understand how the patient monitor handles different device scenarios when considering how to collect patient data.
Standards in Bedside Device Collection
Technical standards developed for medical device communication are available. These standards are supposed to simplify the problem of getting device data into multiple data systems, but often are not adopted by device manufacturers. Efforts to establish a medical device connectivity standard began in the 1980s with the Medical Information Bus (MIB). The MIB was developed by representatives from medical device manufacturers, health care institutions, and academic departments who advocated “plug-and-play” data sharing among bedside devices irrespective of device vendor. In 1996 the MIB specification was approved by the Institute of Electrical and Electronic Engineers (IEEE) Standards Board under the name “IEEE 1073 Standard for Medical Device Communications.” The IEEE 1073 standard continued to evolve, and in 2004 the International Organization for Standardization (ISO) formally ratified IEEE 1073 as “ISO 11073 Point-of-Care Medical Device Communication Standard. Despite this, the ISO/IEEE 11073 standard still is not widely used by the medical device industry. Consequently, most hospitals have limited or no integration of bedside device data with their EHR systems and other clinical information systems.
Regulatory concerns present another barrier to medical device connectivity at least in the United States. The U.S. Food and Drug Administration (FDA) requires a manufacturer of a data collection device to test the connections to each device from which it receives data rather than just test to ensure proper implementation of a standard (such as ISO/IEEE 11073). This presents an unnecessary burden on the manufacturer, similar to a computer manufacturer’s having to test the connection to every printer rather than just test to the USB standard. The FDA realizes the benefits of medical device connectivity and the regulatory issues are being addressed as part of the mission of the Medical Device Plug and Play (MDPnP) program.
Transferring Data Over the Network
The simplest configuration to transfer data from a medical device to a computer is to plug a RS-232 cable purchased from the local computer store into the RS-232 output of the medical device and then plug the other end into the nine-pin communication (COM) port of a regular personal computer. A serial-to-TCP/IP converter enables one to send data from multiple bedside devices over the hospital or local network to a server located in another room or building. Placed in the patient’s room and “installed” on the server, it creates multiple COM ports that allow many devices to plug into it and transfer its data over the network using standard TCP/IP protocols. These converters are available in many configurations including virtual and wireless.
Special care is needed when wireless communication is used to acquire data from bedside medical devices. First, if mobile monitoring solutions rely on battery power, power consumption issues must be considered. Second, wireless communication devices are a cause of radio frequency (RF) electromagnetic interference (EMI) that may cause undesirable effects to medical equipment. It is recommended that testing be performed for EMI between mobile wireless communication and medical devices. Finally, with many devices and multiple wireless technologies competing to use the same frequency spectrum to transmit, crowding can occur that may decrease throughput and affect the reliability of data exchange. Device manufacturers that use RF communication should provide an analysis of data communication requirements, including estimated bandwidth utilization at periods of peak and normal usage.
Data overload is one of the greatest informatics challenges in the NCCU for both medical informaticians and intensive care clinicians. For example, at Columbia University NCCU bedside monitors store approximately 200 megabytes (MB) per day per bed, whereas continuous electroencephalograms (EEGs) can generate approximately 1 gigabyte (GB) per day for EEG alone, and 20 GB per day when there is video recording. When multiple systems in multiple patient rooms record simultaneously, network performance can slow down or data loss is possible. Modern Ethernet networks that use 1 GB per second or greater connections between switches and routers should be used throughout the network to help avoid this problem. It also is important to check for potential bottleneck areas where older systems that use 10 or 100 MB per second connections may exist (e.g., when networks span new and old buildings).
Linking Patients to Their Data
Medical device connectivity is complex, and this extends beyond simply the physical connectors and communication protocols. Perhaps more important is to establish patient context and ensure that device(s) data are reliably associated with the correct patient. A wired infrastructure simplifies patient association because each device can be connected unambiguously to a particular location (i.e., room or bed number). Use of existing electronic admission/discharge/transfer (ADT) systems to map a specific patient to a location can link device data to a patient. However, mobile devices (e.g., infusion pumps) and wireless data communication introduce additional challenges for patient identification. Some devices allow a patient’s medical record number to be entered into the device interface, or provide bar code scanning capabilities to accomplish the same task.
It is essential to consider how data will be linked to a patient before device purchase. There may be an advantage to automatic data documentation directly to an electronic medical record (EMR) from devices such as ventilators and infusion pumps; several studies show this is more accurate and saves nursing time. In some ICUs ventilators are always connected to the bedside monitor and their data automatically transmitted to an EMR for verification. Infusion pumps should have the capability to send streaming data, but not all are able to do this; some that do, send anonymous drug and dose data to a server to help biomedical departments with asset management and device maintenance. Transmission of anonymous data bypasses the Health Insurance Portability and Accountability Act (HIPAA) and privacy concerns. This alleviates potential headaches for biomedical engineering and informatics departments; however, this does not help clinicians manage patients. These various issues need to be addressed before making purchase decisions. No matter the mechanism used to link device data to patients, local testing and periodic validation should be performed to ensure accuracy.
Time Synchronization
It is critical to synchronize data together from multiple devices for accurate bedside device data collection. Each device may maintain its own internal clock, and additional clocks may exist for the data acquisition system and the hospital clinical information system. When data that are used for time-sensitive analyses (e.g., when does an infused medication affects vital signs, when do alarms trigger, and what is the duration?) are recorded, it is important to know exactly the order and delay between events. The Network Time Protocol (NTP) is the most commonly used Internet time protocol and can be used if utilities for time synchronization are otherwise unavailable.
Other Data Collection Considerations
It is important to anticipate how the data may be used to prepare an effective strategy for physiologic data acquisition in the NCCU. Is the purpose of data collection to facilitate clinical care, research, or both? If the data are to be used to provide patient care and there is an existing EHR system in use, it is important to decide whether bedside device data will be automatically transferred to the EHR, and if so, how this will be accomplished. Undoubtedly there will be workflow changes for care providers associated with automatic data collection (e.g., documentation and data review processes). To help anticipate some of the sociotechnical challenges that may arise, both technologic and associated with personnel or workflow, the following questions should be addressed:
- ▪
How often will data be transferred to the existing EHR system?
- ▪
Will manual verification be required before the data are included in the patient’s record?
- ▪
For each data element that is currently documented in the patient’s record, does that element exist in the bedside device’s output stream?
- ▪
Will filters be applied to the data to reduce artifact (i.e., “noise” in the signal) or select representative measurements?
- ▪
Will the same type of measurement be obtained simultaneously from separate devices (e.g., respiratory rate collected from a patient monitor and a mechanical ventilator)? How will these measurements be dealt with?
- ▪
How will downtime procedures be created and enforced (for the EHR system as well as the data collection system)?
Some clinical information systems may not be capable of handling second-by-second or even minute-by-minute recording of physiologic variables. If data are to be stored in a secondary or research database instead of or in addition to an EHR system, several other questions should be considered:
- ▪
How will data be stored (e.g., flat files, relational database, XML database)?
- ▪
How will data backups be performed?
- ▪
How frequently can or should data be acquired and stored (e.g., 500 Hz, 1 sec, 5 sec, 1 min, 15 min, 1 hr)?
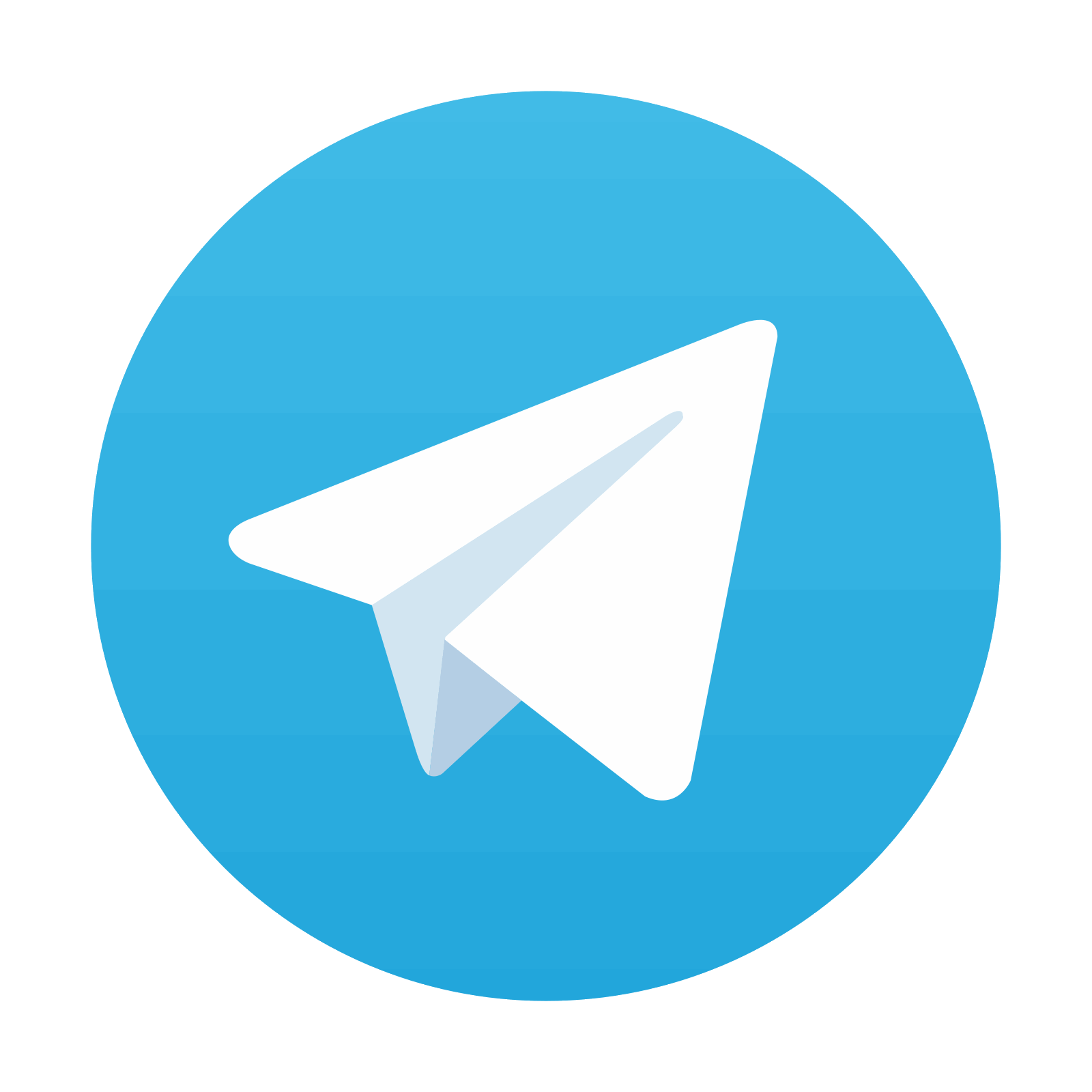
Stay updated, free articles. Join our Telegram channel

Full access? Get Clinical Tree
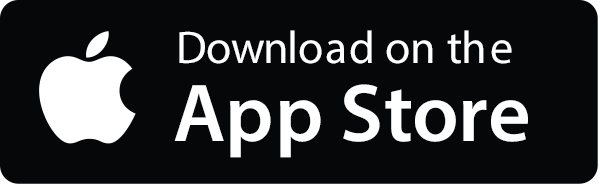
