Fig. 9.1
Basic electrophysiological principles of MEG and EEG. (a) Large neural cells—just like this pyramidal neuron from cortex layer V—drive ionic electrical currents. These latter are essentially impressed by the difference in electrical potentials between the basal and apical dendrites or the cell body, which is due to a mixture of excitatory and inhibitory postsynaptic potentials, which are slow (>10 ms) relatively to action potentials firing and therefore add up efficiently at the scale of synchronized neural ensembles. These primary currents can be modeled using an equivalent current dipole, here represented by a large black arrow. The electrical circuit of currents is closed within the entire head volume by secondary, volume currents shown with the dark plain lines. Additionally, magnetic fields are generated by the primary and secondary currents. The magnetic field lines induced by the primary currents are shown using dashed lines arranged in circles about the dipole source. (b) At a larger spatial scale, the mass effect of currents due to neural cells sustaining similar mixtures of postsynaptic potentials add up locally and behave also as a current dipole (shown in red). This primary generator induces secondary currents (shown in yellow) that travel through the head tissues. They eventually reach the scalp surface, where they can be detected with pairs of electrodes by EEG. Magnetic fields (in green) travel more freely within tissues and are less distorted than current flows. They can be captured with arrays of magnetometers by MEG. The distribution of blue and red colors on the scalp illustrates the continuum of magnetic and electric fields and potentials distributed at the surface of the head
In turn, predicting the electromagnetic fields produced by these source models requires another modeling step. This head modeling process is necessary to address the influence of the head geometry and electromagnetic properties of head tissues on the magnetic fields measured outside the head. Briefly, several well-justified and simplifying assumptions following from MEG physics allow for head geometry to be simplified as a sphere (see: Feynman, Leighton, & Sands, 1964; Hämäläinen, Ilmoniemi, Knuutila, & Lounasmaa, 1993), and hence, the simplest and consequently by far most popular model of head geometry in MEG is a single-layer sphere. In MEG, only the location of the center of the spherical head geometry matters. The respective conductivity and radius of the spherical layers have no influence on the measured MEG fields. This is not the case in EEG, where the location, radii, and respective conductivity of each spherical shell (typically: brain, cerebrospinal fluid, skull, and scalp) influence the distribution and amplitude of surface electrical potentials. Thus, for applications involving simultaneous EEG acquisition (and their source analysis), more complex head modeling approaches such as boundary and finite element head modeling are strongly suggested (Wolters, et al., 2006). These provide more realistic head models via geometric tessellations of the different head tissue envelopes extracted from the subject’s individual MRI volume data, though they remain significantly more intensive computationally.
While the topics of forward and inverse modeling are complex and would require an in-depth review per se (see Hansen, Kringelbach, & Salmelin, 2010), this is beyond the scope of this chapter. To a clinical investigator embarking on an MEG evaluation program, it is necessary to be aware that MEG source analysis is fundamentally a modeling problem, involving various tradeoffs and approximations, which can be approached in a number of ways. The specific approach taken should be dictated by the goals and constraints of the study. Today, a reasonable degree of technical maturity has been reached by electromagnetic brain imaging using MEG, and the approaches to source estimation reduce to only a handful of well-identified classes.
MEG Source Modeling: Localization vs. Imaging Approaches
The localization approach to MEG source estimation considers that brain activity at any time instant is generated by a relatively small number (a handful, at most) of brain regions. Each source is therefore represented by an elementary model, such as an ECD, that captures local distributions of neural currents. Ultimately, each elementary source is back projected or constrained to the subject’s brain volume or an MRI anatomical template, for further interpretation. Localization models are essentially compact, in terms of number of generators involved and their surface extension (from point-like to small cortical surface patches).
The early MEG literature is abundant in studies reporting on single-dipole source models. The somatotopic, tonotopic, and retinotopic responses are examples where the single-dipole model contributed to the better temporal characterization of primary sensory area responses. However, later components of evoked fields usually necessitate that more elementary sources be adjusted, and this is detrimental to the numerical stability and robustness of the inverse model. The number of elementary sources in the model is often qualitatively assessed by expert users, which may undermine the reproducibility of the analyses. Hence, special care should be brought to the evaluation of the stability and robustness of the estimated source models. With all that in mind, source localization techniques have proven to be effective, even in complex experimental paradigms (Helenius, Parviainen, Paetau, & Salmelin, 2009). Indeed, as we outline below, dipole-based approaches currently constitute the most widely applied and best-replicated paradigms for presurgical language mapping with MEG. The alternative imaging approaches to source modeling were originally inspired by the plethoric research in digital image restoration and reconstruction in other fields of engineering.
In these approaches, dense grids of current dipoles are predefined over the entire brain volume or limited to the cortical gray matter surface. These dipoles are fixed in location and, generally, orientation, and their amplitudes are estimated at once. Thus, the nonlinear parameters (e.g., the elementary source locations) now become fixed priors as provided by anatomical information, and the remaining free parameters are the amplitudes of the elementary source currents distributed on the brain’s geometry. The resulting image source models do not yield small sets of local elementary models but rather the distribution of “all” neural currents. As the estimated sources are spatially fixed in this approach, they are homologous to the pixels of a digital image. Hence, the resulting estimations are a set of images or maps, representing an estimation of the intensity of neural currents, distributed within the entire brain volume or constrained at the cortical surface. Contrary to the localization model though, there is no intrinsic sense of distinct, active source regions per se. Explicit identification of activity issued from discrete brain regions usually necessitates complementary analysis, such as empirical or inference-driven amplitude thresholding, to discard elementary sources of nonsignificant contribution according to the statistical appraisal. In that respect, MEG source images are very similar in essence to the activation maps obtained in fMRI, with the supplementary benefit of time resolution (Fig. 9.2).
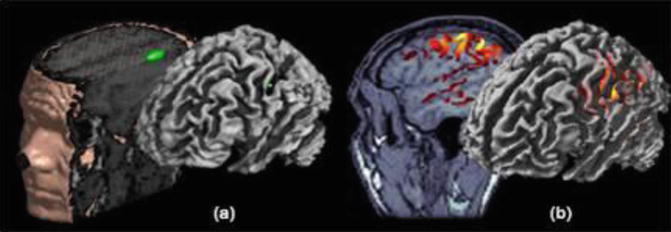
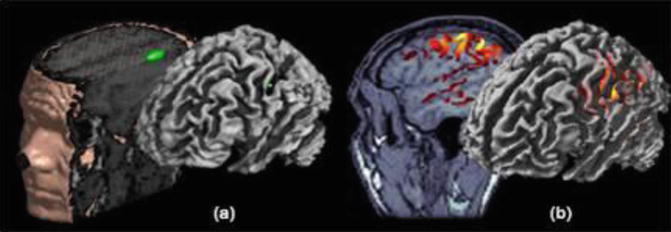
Fig. 9.2
Inverse modeling: localization (a) vs. imaging (b) approaches. Source modeling through localization consists in decomposing the MEG/EEG generators in a handful of elementary source contributions, the simplest source model in this situation being the ECD. This is illustrated here with experimental data from testing the somatotopic organization of primary cortical representations of hand fingers. The parameters of the single ECD have been adjusted on the 20–40 ms time window following stimulus onset. The ECD was found to localize along the contralateral central sulcus as revealed by the three-dimensional rendering obtained after the source location has been registered to the individual anatomy. In the imaging approach, the source model is spatially distributed on a large number of ECDs. Here, a surface model of MEG-EEG generators was constrained to the individual brain surface extracted from T1-weighted MR images. Elemental source amplitudes are interpolated onto the cortex, which yields an image-like distribution of the amplitudes of cortical currents
Just like in the context of source localization where, for example, the number of sources is a restrictive prior as a remedy to ill-posedness, imaging models also must be complemented by a priori information. Several academic software packages have addressed this issue by implementing variations of the well-described minimum norm model (Lin, et al., 2006; Lin, Belliveau, Dale, & Hämäläinen, 2006). For present purposes, it is important only that readers be aware of the equivalent need for prior information in both the localization and imaging approaches. These priors are necessary to determine a workable solution to a problem with an infinite number of solutions, in theory.
A final set of approaches involve signal classification and spatial filtering (e.g., “beam forming”) to translate the source localization problem into a signal detection issue. In essence these are scanning techniques that systematically sift through the brain space to evaluate how a predetermined elementary source model would fit the data at every voxel of the brain volume. These techniques are efficient alternative approaches in that respect and have gained considerable momentum in the MEG community in the recent years. They have proven especially fruitful in several applications (for both source estimation and artifact suppression) and the interested reader is referred to a review article by Hillebrand, Singh, Holliday, Furlong, and Barnes (2005) for an introduction to the approach.
Each of these three approaches, MEG source localization, imaging, and scanning, makes use of phenomenally complex methodology and modeling techniques to noninvasively localize the source of magnetic neural activity within the brain. As with any experimental observation, they are subject to error and benefit from explicit assessments of their sensitivity to measure noise and modeling approximations (see, e.g., Darvas, et al., 2005; Mosher, Spencer, Leahy, & Lewis, 1993). Ultimately, an informed consideration of their various assumptions, strengths, and weaknesses, as described above, can guide their optimal application to any given clinical evaluation.
Practical Considerations
Despite the many complex aspects of magnetic source imaging, the preceding exposition can easily be reduced to a handful of essential points to consider before beginning a program of MEG presurgical evaluation. As with fMRI, an efficiently functioning MEG laboratory requires the effort of a multidisciplinary team. The various requirements would ideally be met by a group of individuals with collective expertise in MEG physics, data acquisition, software engineering, task design and development, cognitive evaluation, and neuroanatomy, epileptology, neuro-oncology, etc.
Developing a standardized data acquisition and analysis protocol simply requires explicitly planning through the steps outlined above (e.g., subject preparation, data acquisition, quality control, source modeling, etc). It is important to remember that the tasks, stimuli, and analysis approach in MEG protocols should be designed in a coordinated and complementary fashion and specifically with sensitivity to how the temporal aspects of the stimuli, and expected behavioral and neural responses will condition the access to a subset of methods for data analysis. For example, it might be discussed whether or not to collect behavioral data simultaneously with MEG or whether the data should be analyzed as stimulus-locked vs. response-locked epochs. Event-related responses in cognitive tasks most often contain both early components (50–200 ms post-stimulus) reflecting the primary sensory processing and integration of the stimulus and later components (>200 ms post-stimulus) that are typically thought to reflect activity in association cortices. It has therefore been argued that the mapping of higher cognitive functions with MEG may require restricting analyses to the later components of the brain responses (Simos, Papanicolaou, Castillo, & Buchanan, 2009). Decisions such as duration of stimulus presentation and length and variability of interstimulus intervals (ISIs) need to be guided by the specifics of the task modality and of the underlying scientific hypothesis to be tested (e.g., visual stimuli tend to elicit saccades, auditory stimuli unfold over time, etc.) and the expected nature of the neural responses of interest (Salmelin & Parkkonen, 2010). There are several academic or commercial software packages for MEG data analysis, and the major MEG vendors provide their own software for many aspects of data processing. Additionally, the reference papers for several excellent and freely available software packages can be found at: http://www.hindawi.com/journals/cin/2011/si.ebm/.
As discussed above, MEG recording is greatly facilitated if caution is taken prior to scanning to remove easily eliminated sources of magnetic artifacts (e.g., pieces of metal in garments). To this end, it is a good investment of time to briefly conduct a noise run with the subject sitting or laying under the MEG dewar to help identify any unsuspected artifacts, before subject preparation is completed by applying HPI coils and, optionally, EEG leads. This step has the additional benefit of familiarizing the patient with the MSR environment before the clinical scan per se. For the most part, the prior recommendations regarding behavioral monitoring and testing outside of the instrument in the context of fMRI also apply to MEG. Also like fMRI, it has been reported that language-related activity is impacted by factors such as subject alertness, task compliance, and IQ (Merrifield, Simos, Papanicolaou, Philpott, & Sutherling, 2007; Simos et al., 2009), though there are ongoing efforts to develop MEG language protocols that are better suited for use with pediatric and cognitively lower-functioning individuals. Unlike the highly magnetized MRI environment, patient safety concerns within the MSR primarily relate to clinical concerns. The most important of these is always having appropriately trained personnel ready to rapidly enter and assist the patient within the enclosed MSR, in case of an acute seizure or other medical emergency.
Clinical Use of MEG by Neuropsychologists
For the same reasons outlined in the fMRI chapter of this volume, neuropsychologists can clearly play an appropriate and valuable role in the design, administration, and interpretation of MEG studies of higher cognitive functions. In 2009, the American Academy of Neurology published a policy regarding MEG, including indications to “localize and preserve eloquent cortex during resective surgery,” in the context of surgeries for tumors and arteriovenous malformations, in addition to MEG indications for epilepsy surgical planning (see Wu et al., 2010). MEG CPT codes have been issued for these clinical conditions http://acmegs.org/id77.html under “MEG recording and analysis” for localization of language, as well as sensory and motor functions, with the former representing an excellent venue for integrating neuropsychological expertise. As of this writing, we are not aware of any official position statements regarding the role of neuropsychologists in the clinical use of MEG. Given the historical importance that localization of cognitive functions played within the discipline of clinical neuropsychology, as well as ongoing discussions about expanding roles for neuropsychologists (Bilder, 2011; Miller, Elbert, Sutton, & Heller, 2007), this may be a timely topic for discussion within neuropsychological professional organizations and training programs.
Tasks
There are a number of tasks that have been utilized to map language-specific cortical regions with MEG. Fewer studies have examined episodic memory with MEG in ways that are immediately relevant to presurgical epilepsy evaluations. By far, the most widely studied and best-replicated task for evaluation of the laterality of language functions with MEG is the continuous recognition memory (CRM) paradigm developed by Papanicolaou and colleagues (Frye, Rezaie, & Papanicolaou, 2009). A common version of the task involves presenting the subject with a list of target words (abstract English nouns) immediately prior to the scan, which they are asked to study and accurately repeat. The acquisition scan involves presenting the subject with frequent spoken targets that are randomly interspersed with novel distractor items. Subjects are instructed to immediately issue a manual response (a finger lift) upon presentation of a target and otherwise make no response. Thus, the task essentially represents an auditory verbal recognition memory paradigm.
The analysis stream most often utilized for this task involves identifying the locations of successive dipolar sources that account for the magnetic flux distribution in the “late” (i.e., >200 ms) portion of the post-stimulus period. The acquisition run is often divided into two subsets where the spatial and temporal overlap of the obtained dipoles is assessed, and those which are reliable between the two subsets are retained. The resulting dipole maps depict clusters of reliable source locations. In a manner similar to fMRI, a laterality index can be calculated by taking the difference between the number of right- and left-hemisphere sources divided by their sum, thereby providing a hemispheric dominance index (LI) that varies between 1 and −1 (see Simos et al., 2009 for additional details). This task has been shown to localize activity within posterior language areas (i.e., in the vicinity of the superior and middle temporal gyri) in a number of empirical reports (e.g., Breier et al., 2001; Merrifield et al., 2007; Papanicolaou et al., 1999) including large samples of epilepsy patients (Papanicolaou et al., 2004), in both auditory and visual stimulus modalities (Papanicolaou et al., 2006) and in samples of non-English speakers.
As recently reviewed by Pirmoradi, Béland, Nguyen, Bacon, and Lassonde (2010), many other tasks have also been investigated for functionally mapping language functions with MEG. In addition to variants of the CRM task outlined above, they cite numerous other paradigms designed to either determine hemispheric language dominance or localize systems related to specific functions (Pirmoradi et al., 2010). These include: passive listening and counting paradigms (Kim & Chung, 2008; Martin et al., 1993; Szymanski et al., 2001; Szymanski, Rowley, & Roberts, 1999); grammatical or semantic categorization, matching, or judgment (Härle, Dobe, Cohen, & Rockstroh, 2002; Kamada et al., 2006, 2007; McDonald et al., 2009; Simos, Breier, Zouridakis, & Papanicolaou, 1998); and note/tone vs. vowel comparisons (Gootjes, Raij, Salmelin, & Hari, 1999; Kirveskari, Salmelin, & Hari, 2006).
Other reports have more explicitly examined neural systems involved in language output, via both isolated and combined studies of covert reading and picture naming (Breier et al., 2001; Hirata et al., 2010; Kober et al., 2001), delayed and overt naming (Fisher et al., 2008; Levelt, Praamstra, Meyer, Helenius, & Salmelin, 1998; Salmelin, Hari, Lounasmaa, & Sams, 1994) and reading (Salmelin, Schnitzler, Schmitz, & Freund, 2000), and delayed, covert, and overt word or verb generation tasks (Bowyer et al., 2005; Fisher et al., 2008; Pang, Wang, Malone, Kadis, & Donner, 2011; Ressel et al., 2006; Yamamoto et al., 2006). Despite over a decade of research, and many reliable findings, the MEG language and memory mapping literature is still at somewhat of an earlier stage of development than that of fMRI, owing largely to the smaller MEG community and the more recent maturation of well-delineated source modeling approaches and the availability of software tools.
Activation and Contrast Tasks
The source localization approach has been the dominant paradigm in MEG cognitive mapping for a number of years, with applications of imaging approaches emerging more recently. For studies utilizing ECDs the number of successive dipoles within a region of interest (or their difference between the hemispheres) has generally been utilized as a measure of the “extent” of neural activation. The report of Bowyer et al. (2005) is one notable exception in that they utilized an imaging approach and the relative duration of activity within left- and right-hemisphere regions for calculating LIs, thereby highlighting the diverse metrics available for studying language lateralization with MEG. Given that focal source models necessarily spatially constrain what is presumably distributed activation (at least in the case of higher cognitive functions), source imaging and spatial filtering approaches naturally provide superior indices of the spatial distribution of language-related neural activity.
Contrast tasks have been utilized less frequently in MEG language research than in fMRI, though this trend is changing, especially with the wider application of source imaging models (e.g., Kamada et al., 1998; Kober et al., 2001; McDonald et al., 2009). Whereas changes in the BOLD signal are measured on the order of seconds, in many instances MEG paradigms are designed to detect only the inherently transient changes, which are thought to be more phase locked than time locked to stimulus presentation. This has been suggested as a reason for lack of correspondence with fMRI results (Liljeström, Hultén, Parkkonen, & Salmelin, 2009; Pang et al., 2011). Devising effective MEG contrasts is thus complicated by the additional dimension of time, since the timing of component neural processes might meaningfully differ depending on the features of probe and contrast tasks (Salmelin & Parkkonen, 2010). In such cases, a simple subtraction of contrast and probe task maps could lead to erroneous conclusions if the contrast does not optimally equate for processes peripheral to the study question (e.g., visual processing, attention) and in a way that does not alter their timing. As a solution to this issue, Levelt et al. (1998) argued that if the component processes of a given behavior can be separated into their temporal stages (i.e., object recognition, lexical access, etc., in the case of picture naming), one can manipulate any given stage and only alter the time course of the subsequent stages. The absence of activity in the subtraction maps at early latencies provides some assurance that probe and contrast tasks were well matched in terms of their perceptual attributes (McDonald et al., 2009). Finally, like fMRI, the extent of “activated” cortex detected by an MEG source model strongly depends on the nature of the task, modeling approach, and the statistical thresholds applied (Liljeström et al., 2009).
Behavioral Monitoring
Due to the large magnetic artifacts created by the facial muscles involved in speaking, overt language tasks present a challenge for monitoring spoken responses in MEG. Nonetheless, MEG has been successfully utilized for localizing both receptive and expressive aspects of language functions, including with paradigms that permit behavioral monitoring. A number of studies report localization of language-related activity without recording behavioral data (Hirata et al., 2010; Yamamoto et al., 2006) though most paradigms have utilized covert reading or naming in conjunction with manual responses to ensure accurate task performance. Some groups have utilized vigilance trials which are unrelated to the task, such as a randomly interspersed stimulus prompting a button press, to help ensure attention to the task. Overt and delayed responses have also been successfully collected in MEG studies (Salmelin et al., 1994), including for challenging contexts such as chronic stuttering (Salmelin et al., 2000).
Although behavioral monitoring is clearly desirable to ensure task compliance and/or equate for difficulty between conditions, functional language mapping has been less successful in individuals who are unable to comply with task demands. For this subset of patients, it would be advantageous to have paradigms capable of establishing hemispheric language dominance using tasks requiring minimal behavioral compliance. At least two reports have approached this by examining lateralization of the 100 ms auditory response (N100m) to speech sounds in a simple behavioral condition (Gootjes et al., 1999; Kirveskari et al., 2006). In these studies healthy subjects listened to pairs of tones or spoken vowel sounds and were asked to respond with a finger lift when they perceived that the two stimuli were identical. This task is arguably minimally cognitively demanding. Nontarget sounds were analyzed and in both reports results demonstrated stronger N100m responses to speech sounds but not pure tones in the left compared to the right hemisphere, in right-handed subjects. The latter report also included weakly right-handed and left-handed subjects, and LIs showed trends for less left-lateralized N100m responses to speech sounds in the those groups; an effect that significantly differed between strong right-handers and left-handers (Kirveskari et al., 2006).
Separately, Kim and Chung (2008) employed an auditory oddball task involving two spoken monosyllabic words, with each being the standard and deviant stimulus, respectively. Participants were epilepsy patients who were merely asked to ignore the auditory stimuli and watch a movie during the recording, making no response to the spoken words. LIs generated from the oscillatory mismatch negativity response to deviant stimuli demonstrated 71 % concordance with Wada results for a posterior superior temporal gyrus ROI and between 76 % and 94 % concordance for a posterior inferior frontal gyrus ROI.
Episodic Memory
There have been relatively few MEG studies that have specifically examined memory functions in epilepsy. One study utilized a variant of the continuous recognition memory paradigm to examine medial temporal lobe activity in a group of healthy subjects and patients with left mesial temporal sclerosis (LMTS) (Ver Hoef, Sawrie, Killen, & Knowlton, 2008). The authors reasoned that since the performance of the CRM task involves both language and memory processes, the paradigm might elicit lateralized medial temporal lobe activity in addition to the robust activation it elicits in posterior temporal areas. A variant of the auditory CRM protocol was investigated in a final sample of six patients with LMTS and seven healthy controls, and a source localization approach involving clusters of dipoles was utilized. Across two runs of the CRM task, left mesial temporal dipole clusters were observed in the majority of control subjects but not LMTS patients (86 vs. 33 %). Conversely, right medial temporal dipole clusters were observed in all LMTS patients (100 %), relative to only 2 of 7 control subjects (28 %), with the difference being statistically significant. The direction of medial temporal LIs also significantly differed between groups, with patients demonstrating rightward mesial temporal laterality, relative to left lateralization in controls. Finally, patients and controls also significantly differed with regard to the number of runs on which each respective group showed right medial temporal activity (67 vs. 7 %).
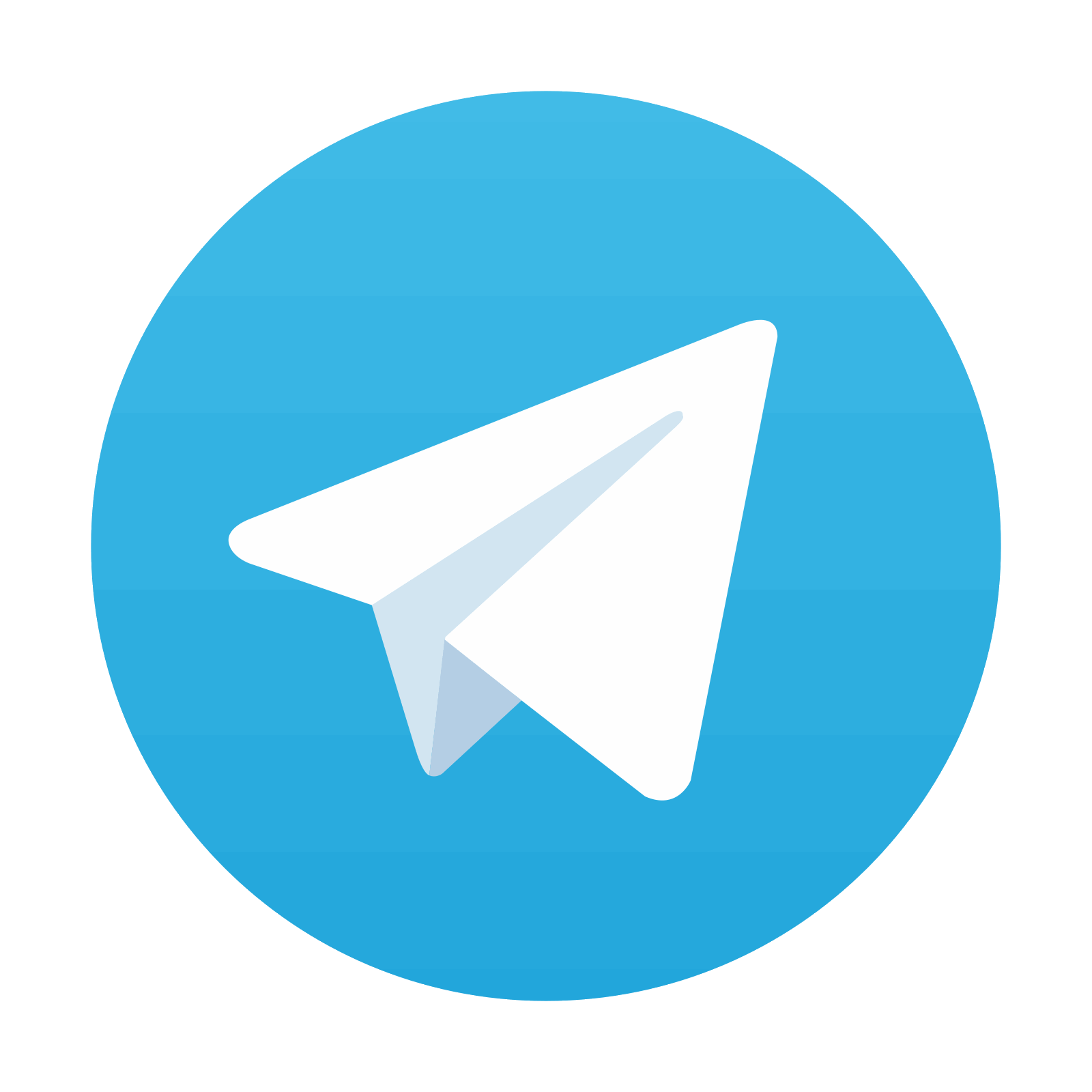
Stay updated, free articles. Join our Telegram channel

Full access? Get Clinical Tree
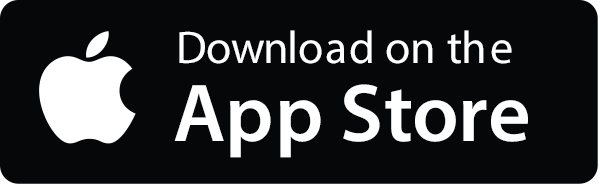
