Proteomic and metabolomic methodologies
In the general AD population, proteomic and metabolomic studies are under close scrutiny as inconsistency in outcomes and variability in preanalytic methods (sample collection, processing, storage, etc.) have led to inconsistent results thus making it difficult to directly compare studies. One significant contribution made over the last decade was the standardization of many preanalytic methods for studies using blood biomarkers associated with AD through an international working group [7]. Findings show that proteins derived from serum and plasma can be differentially expressed due to a variety of reasons such as clotting factors. For untargeted (unbiased) large-scale proteomic analyses, mass spectrometry (MS)-based methods are frequently utilized. In comparison, for targeted proteomic analyses an enzyme-linked immunosorbent assay (ELISAs) method that employs a plate-based assay approach is often considered to be the gold standard [8]. Furthermore, advances in biomedical technology have led to the ability to detect very low abundance proteins via ultrasensitive equipment such as single molecule array (SIMOA) immunoassays [9].
Similar technological advances have enabled metabolomic approaches to AD biomarker development in recent years. In less than a decade, MS-based analytical platforms have nearly tripled in their capacity to reproducibly identify and quantify human peripheral plasma lipids [10]. Preanalytic considerations in metabolomics, as in proteomics, have proven highly instrumental to these aims [11, 12]. Moving beyond viewing biomarker measurements as purely quantitative statistical values, emerging systems biology methods have also advanced our capacity to understand dementia-associated changes in a broader, semantic biochemical context [13].
Proteomic profiles and predictors of dementia
As individuals with DS age, the deposition of amyloid continues to increase as a result of trisomy 21 [14–16] (see Chapter 2). Therefore initial use of proteomics to detect AD among this population focused largely on amyloid beta (Aβ) peptides (see Chapter 6). Although Aβ has been examined across the lifespan among those with DS, proteomic studies focused on older adults have produced contradictory findings between Aβ and DS-AD despite the known neuropathological link. This inconsistency in findings spans Aβ peptides (Aβ1–40 and Aβ1–42) with a number of studies reporting elevations in both Aβ1–42 [5, 17–21] and Aβ1–40 [5, 17, 18, 22] among those with DS-AD, while others report a decrease (Aβ1–42 [22]; Aβ1–40 [19]) when compared to healthy neurotypical controls.
The focus of studies examining the link between Aβ peptides and dementia among adults with DS has mirrored that of research conducted in the general AD population. As with the general population, inconsistencies in study results and the emergence of evidence to suggest multiple disease pathways have strengthened the field’s resolve to examine proteins across a range of biological systems. One such study conducted by Iulita and colleagues (2016) explored the link between inflammatory pathways and DS-AD. Results revealed elevations across several proteins associated with inflammation, including tumor necrosis factor alpha (TNF-α), interferon gamma (INF-y), interleukin 6 (IL-6), IL-10, and IL-8 among individuals with DS-AD as compared to cognitively healthy individuals without DS [18].
A more recent study sought to generate biomarker profiles utilizing machine learning techniques to detect prevalent mild cognitive impairment among individuals with DS (MCI-DS) and DS-AD based on work utilizing identical plasma proteins in the general AD population [23]. MCI-DS cases included individuals with DS who presented with some cognitive and/or functional change; however, this change was not to such a degree that it met diagnostic criteria for dementia. Findings from this work revealed distinct proteomic profiles for prevalent MCI-DS as well as DS-AD with detection accuracy ranging from an area under the curve (AUC) of 0.92 to 0.89 [23]. Of note, AUC was used to distinguish between different diagnostic classification (MCI-DS and/or DS-AD from cognitive normal cases) with higher performance indicating better predictive ability of the model. Although the AUC was higher for prevalent MCI-DS as compared to DS-AD, overall detection when using an optimized cut-off score produced strong support for this application in detecting disease state. Variable importance plots revealed that inflammatory markers were highly important in both MCI-DS and DS-AD. Proteins such as IL-10, beta 2 microglobulin (B2M), c-reactive protein (CRP), thymus and activation regulated chemokine (TARC), and TNF-α were among the driving proteins for both proteomic profiles [23].
When the same targeted plasma proteins were used to detect incident cases (as opposed to previous work examining prevalent cases [23]) of MCI-DS and DS-AD, among the same cohort, the blood profile performed equally well with an AUC of 0.92 for incident MCI-DS and 0.88 for incident DS-AD [24]. Support vector machine (SVM)-based proteomic risk scores were then applied to Cox proportional hazard models. SVM is a form of machine learning and is primarily a classifier model. It is also considered to be among the best classification algorithms [25, 26]. Risk scores based on a dichotomized high/low cut point score predicted onset for both incident MCI-DS and DS-AD, when adjusting for covariates of age, gender, level of ID, ethnicity, and APOE e4 carrier status [24]. As with the prior study, a number of inflammatory-based proteins (CRP, IL-10, TARC, IL-5, IL-7) were among the top identified for both incident MCI-DS and DS-AD cases.
Understanding the proteomic profiles is important as it reflects the complexity of AD particularly among those at increased risk such as those with DS. As the studies mentioned before illustrate, the top proteins for both prevalent and incident cases of MCI-DS and DS-AD include a combination of antiinflammatory (IL-10) as well as pro-inflammatory (IL-7, IL-5, TARC) proteins [23, 24]. Prior work has shown a correlation between serum antiinflammatory (IL-10) and the pro-inflammatory proteins (TNF-α) among those with LOAD [27] and the work referenced before reflects similar findings as the variable importance plots highlight both the variability and the robust impact of inflammation. This combination of inflammatory proteins also serves to reflect the sheer complexity of the biological response across varying stages of AD.
A recent systematic review of blood-based biomarkers among adults with DS was completed in 2019 [28]. This review included 21 studies and, of those studies, all but one [29] focused on proteins other than those derived from plasma. This focus largely on plasma is consistent with the work on AD in the general population. However, in the general AD population, several studies have validated a serum-based proteomic profile for detecting AD across multiple cohorts [30, 31], assays, species, and tissues [32]. The application of serum proteomic profiles has also been extended to other neurodegenerative diseases including Dementia due to Parkinson’s disease [33] and Lewy Body Dementia [34] in the general population.
A few studies have directly examined the ability to use either serum or plasma proteins to detect AD in the general population. One study that looked at the comparability of proteomic biomarkers across serum and plasma found that less than half of the proteins examined shared over 50% of variance across fractions (i.e., plasma vs serum) [35]. Because the majority of work conducted on proteomic biomarkers of MCI and AD among adults with DS have looked at plasma-based proteins, we conducted a recent study to examine the utility of both plasma and serum proteomic profiles to determine (1) how the proteomic profile for serum differed from the proteomic profile derived from plasma in detecting disease status (MCI-DS, DS-AD) and (2) compare the same proteins across fractions to determine correlations [36]. Findings from this study revealed that when distinguishing MCI-DS from cognitively stable people with DS, the serum and plasma proteomic profiles performed similarly well with AUCs of 0.98. Similar results were shown for DS-AD with AUCs of > 0.98 [36]. As with other studies that have examined proteomic profiles of prevalent and incident MCI-DS and DS-AD, a number of inflammatory-based proteins were shown toward the top of the variable importance plots (MCI-DS: IL-10, CRP, Factor7, IL-6, B2M; DS-AD: IL-10, IL-5, Factor7, IL-18, IL-6) [36]. Additionally, in this study, a number of proteins correlated highly across fractions; however, only Eotaxin3, IL-6, IL-10, CRP, IL-18, SAA, and FABP3 produced > 80% shared variance [36].
Neurofilament light chain (Nf-L) is also being increasingly explored among adults with DS given its support within the general AD population as a marker of neurodegeneration [37]. Early work comparing the utility of Nf-L to other plasma biomarkers commonly associated with AD neuropathology (Aβ, tau) revealed that Nf-L alone produced a higher accuracy (AUC = 0.88) for distinguishing cognitively stable individuals with DS from those with prodromal AD when compared to other plasma biomarkers (AUCs ranged from 0.53 to 0.74) [5]. In this same study, the derived AUC increased to 95% when Nf-L was applied to distinguish individuals with DS who were cognitively stable from those with AD dementia [5]. Recent work from a large NIH multisite study (Alzheimer’s Biomarker Consortium-Down Syndrome; ABC-DS) found comparable results as plasma Nf-L alone produced an AUC of 0.65 for distinguishing cognitive stable individuals with DS from those with MCI-DS; however, Nf-L alone yielded an AUC of 0.90 for distinguishing DS-AD from cognitively stable adults with DS [38].
A recent study examined plasma Aβ42, tau (NT1 tau), and Nf-L across the lifespan (3–68 years old) among individuals with DS as compared to age-matched neurotypical control cases. This study found that Aβ was elevated among people with DS compared to control cases across the lifespan; however, the difference between the groups decreased with advancing age [39]. In contrast, Nf-L was shown to be relatively similar between DS and neurotypical controls until age 30, at which time, individuals with DS showed a greater increase in Nf-L levels [39]. NT1 tau levels were higher among adults age > 50 years of age with DS compared to age-matched neurotypical controls [39].
Metabolomics: A practical primer
Metabolomics workflows suitable for biomarker discovery are logistically complex and represent an active area of research and development within the field [12, 40]. The complexity of these workflows remains a challenging aspect of metabolomics and a potentially limiting factor to the discussion of emerging metabolomic findings among those studying DS-AD broadly. Metabolomics, in the most general sense, is the systems-level, “-omic” methodology attempting to extensively survey low molecular weight (e.g., less than 1.5 kDa) biomolecules, typically within a fluid matrix such as blood plasma or serum [6, 41]. Analytical chemistry methods such as gas chromatography mass spectrometry (GC-MS), liquid chromatography mass spectrometry (LC-MS), and proton nuclear magnetic resonance spectroscopy (1H NMR) are used to detect and quantify metabolite features [42]. In particular, LC-MS is frequently used to simultaneously profile several thousand metabolite mass features within a given clinical blood specimen. Each of these features represents a mass spectral peak arising from detection by the mass spectrometer. These peaks themselves represent electrically ionized (electrospray ionization; ESI) molecular fragments emerging from reverse phase liquid chromatography, in which the interface between a liquid solvent gradient and a hydrophobic column produces variability in the retention time (RT) of individual metabolites on the column.
Thus, as metabolites elute from the column at different rates, they are differentially detected by the mass spectrometer. For a single blood specimen, this results in several thousand (~ 3000–8000) metabolite features identifiable by RT on the column and their mass-to-charge ratio as determined by the mass spectrometer [43]. Relative abundances of these mass features are derived with respect to an internal standard. To expand analytical coverage, samples are ionized in both positive and negative modes resulting in paired acquisitions for each specimen. Importantly, these LC-MS mass features do not directly reflect single metabolites, but molecular fragments arising from the ionization of these metabolites [44]. Annotation of mass features consequently represents a significant bottleneck in biomarker development and validation [45, 46], with as many as ~ 30% of detected mass features being unannotatable within a given experiment. Limitations of this “untargeted” metabolomics approach have been addressed by several kit-based, targeted platforms that use internal standards to quantify a priori specified compounds with high confidence [47–50]. This provides the additional benefit of measurements made in absolute valued units (e.g., μM) desirable for ultimate clinical use. Although analytical coverage of the targeted metabolome is less extensive than untargeted approaches, targeted methods demonstrate superior potential for reproducibility and interpretability of findings, assuming targeted assay platforms employed are well optimized and possess acceptable quality control [12].
Metabolomics, both broadly and within AD, remains an emerging field in which standardization of methods, and even refinement of aims, is ongoing [51, 52]. We emphasize that this may be a significant consideration in the design of multicohort, multisite, and confirmatory/validation studies in which a lack of uniformity may adversely affect statistical power to detect metabolic alterations of interest [11]. For a more complete treatment of data analytical considerations in the peripheral blood metabolomics of neurodegenerative disease, we refer the reader to Gross et al. [12].
Down syndrome-Alzheimer’s disease: A metabolic dementia?
Over the past decade, multiple research groups have utilized metabolomic approaches to profile peripheral blood alterations associated with cognitive decline and dementia in AD within the general population [53, 54]. These studies suggest that broad clinical status (cognitively stable, mild cognitive impairment, AD), AD-associated central nervous system pathophysiology, and cognitive performance are indeed reflected in alterations to the peripheral blood metabolome [53]. The specific metabolite classes/pathways thus far implicated in AD are diverse, but our group and others have increasingly suggested that aberrant peripheral lipid and fatty acid metabolism may be particularly important [55–58]. This has been substantiated mechanistically [59, 60], observed in both brain parenchyma and peripheral blood [61], and may suggest druggable or otherwise therapeutically modifiable biochemical pathways moderating the relationship between cardiometabolic syndrome and elevated AD risk [62–64].
The association between cardiometabolic syndrome and AD may be of particular interest in DS-AD. This is due to the observation that while individuals with DS develop AD neuropathology by the age of 40 years (see Chapter 2), this occurs in the presence of elevated metabolic risk factors (e.g., elevated triglyceride levels, total cholesterol, greater adiposity) despite lower rates of cardiovascular co-occurring illnesses (e.g., atherosclerosis, hypertension—see Chapter 11) [65]. These latter vascular pathologies, either manifesting alone or in combination with AD, are sufficient to precipitate cognitive decline in other vulnerably aging populations possessing a typical chromosomal complement [65]. Infarcts and vascular dementia, however, present rarely in DS-AD, as compared to genetically typical, aging adults who will develop AD (late-onset AD; LOAD). Indeed, very little is understood about the relationship of the peripheral metabolome to DS-AD specifically. At the broadest level, it is not clear if clinical staging and/or cognitive status are reflected in the plasma metabolome of at-risk adults with DS, as has been shown in alternative models of AD risk. Furthermore, even if metabolomic alterations are evident, it remains unclear if specific metabolites, metabolite classes, or metabolic pathways implicated in AD in the general population are also impacted in DS-AD.
Supporting the involvement of altered lipid metabolism potentially intrinsic to DS itself, Adelekan and colleagues [66] have demonstrated that prepubertal children with DS demonstrate less favorable fasting serum lipid profiles than their euploid siblings even when the body mass index (BMI) is considered as a covariate. Consistent with intrinsic alterations in mitochondrial bioenergetic metabolism in a pediatric DS cohort, Caracausi and colleagues [67] found increased levels of pyruvate, succinate, fumarate, lactate, and formate, which were generally consistent with the 3:2 gene dosage model of trisomy 21. Few groups, however, have examined age-related changes to the peripheral metabolism as they vary with aging in DS. Borelli and colleagues [68] found overall plasma protein hypogalactosylation (i.e., reduced posttranslational modification of proteins by galactose monosaccharide N-terminal addition) in adults with DS when compared to adults without DS, but also an age-related increase in several glycomic features suggesting an accelerated aging phenotype in people with DS. These results suggest that glycosylation may be associated with an aging phenotype in adults with DS that only partially overlaps with aging in euploid adults. They also suggest that altered glycosylation may be associated with DS-AD, given the age-related risk of DS-AD. However, this was not specifically examined.
To directly address the role of peripheral metabolism in at-risk or dementing people with DS, our group has recently contrasted the peripheral metabolome of cognitively stable versus prevalent cases of DS-AD [69]. Using an initial untargeted LC-MS survey approach, metabolic network analysis revealed that metabolite features differentially expressed between the two groups were concentrated within pathways related to pro-inflammatory bioactive lipid signaling, glycerophospholipid metabolism, and components of central carbon metabolism such as the pentose phosphate pathway and hexose metabolism.
Further suggesting a link between lipid homeostatic and bioenergetic processes, de novo pathway reconstruction over those metabolites differentially expressed (DE) in plasma between cognitive stable adults with DS as compared to DS-AD appeared to recapitulate an interface between phospholipid/lyso-phospholipid acyl chain remodeling and fatty acid oxidation via the mitochondrial carnitine shuttle (i.e., Lands’ cycle). This was in addition to our finding that lactate—the terminal product of fermentative, nonmitochondrial glucose metabolism—was upregulated in DS-AD plasma. Potentially possessing relevance for phospholipid biosynthesis and hexose metabolism, we further noted that the pyrimidine nucleoside uridine was downregulated in people with DS-AD compared to those who were cognitively stable. Reduced pyrimidine nucleoside uridine is consistent with a wider literature noting the same pattern in AD broadly for which mechanistic relationships to synaptic membrane integrity have been proposed [70–72]. Contrary to recent studies in AD in the general population, it was not our finding that free amino acid abundances (specifically branched chain amino acids) were significantly different as a function of cognitive status in adults with DS [49].
Although lipid homeostatic and bioenergetics outcome measures suggest metabolic changes characterizing prevalent DS-AD, it remained unclear if the peripheral metabolome could distinguish prevalent cases of prodromal MCI-DS and DS-AD from cognitively stable adults with DS. To evaluate this, we analyzed an initial set of ~ 9000 metabolite features derived from an independent, expanded cohort of at-risk adults with DS (ABC-DS) [73]. We prioritized a subset of these according to DE on the basis of clinical status where cognitively stable MCI-DS and DS-AD were considered as statistically separate groups. Although there were no significant DE metabolites between cognitively stable and MCI-DS at the multiple-testing corrected FDR < 0.05 level, such significant group differences were detected between metabolites in the MCI-DS vs DS-AD and cognitively stable vs DS-AD contrasts. Identified sets of metabolites were used to construct machine learning models discriminating MCI-DS vs DS-AD and cognitively stable vs DS-AD plasma. In both cases, parsimonious models of fewer than 10 metabolite features demonstrated strong (ROC AUC > 0.85) classification of participant bloods by cognitive/clinical phenotypic groups with respect to these pairwise contrasts. This general finding was invariant to the specific choice of classification algorithm and model fitting regimen employed. Importantly, we also submitted DE metabolites (at the nominal P < .05 level) for the cognitively stable vs DS-AD, cognitively stable vs MCI-DS, and MCI-DS vs DS-AD contrasts to metabolic network analysis. Recapitulating our earlier findings, we again noted enrichment for fatty acid metabolism; however, this was specifically implicated in contiguous disease state transitions (cognitively stable vs MCI-DS, MCI-DS vs DS-AD). Findings of fatty acid dysmetabolism require further replication and their underlying biological significance remains unclear; however, it may indicate that nonlinear metabolic programs evident in peripheral circulation may characterize if not drive AD progression, at least in DS-AD [6, 74–77].
Proteomic and metabolomic biomarkers for precision medicine and outcomes for clinical trials
Medical advances have produced both considerable advantages and disadvantages for individuals with DS who are now living well into older ages. The extended life expectancy is also coupled with an increased risk for age-related conditions such as AD (see Chapter 1). Historically, however, few clinical trials have been conducted among adults with DS to either treat or delay onset of AD (see Chapter 16). Given the fact that nearly all adults with DS will have underlying amyloid accumulation by the age of 40 [78] (see Chapter 2), this population is uniquely suited to a novel precision medicine approach for trials. Such an approach takes into account individual biological variability with the overarching goal of treating patients based on their distinct biological composition. For adults with DS, an omics (genomic, proteomic, metabolomic) approach to treatment has the potential to help substratify so that people receive the most effective treatment, thus mirroring the model that has been successfully applied across the field of oncology with identifiable improvements in patient outcomes [79–85]. In fact, the INCLUDE initiative has a clear focus on advancing clinical trials for the DS population. A novel precision medicine approach that can modernize these trials could have tremendous impact particularly among this population given the impact on study cost, participant (and caregiver) burden, trial duration, as well as speed to market for successful molecules.
There are a number of context of use (COU) for novel blood-based biomarkers, which are characterized by the FDA as “a statement that fully and clearly describes the way the medical product development tool is to be used and the medical product development-related purpose of the use” [86]. One COU for blood-based biomarkers would be their potential to serve as the first step in a multitier screening process for clinical trials. As is the case in the general population, clinical trials targeting DS-AD tend to occur only in specialized clinics, which drastically limits access and awareness. Also consistent with the general population, most people with DS are seen by primary care providers. Therefore, a blood-based tool for rapid, large-scale screening of people directly in primary care clinics would drastically increase access to novel trials by aiding with participant selection (COU 1) (see Fig. 1). As highlighted before, recent work clearly sets the stage for such a system [30, 87, 88]. As is the case with all screening instruments in primary care, the first step is to rule out the disease therefore producing a high negative predictive value (NPV). A blood-based tool with an NPV > 90% would rule out nearly all individuals who do not need to undergo follow-up testing [30]. Such a tool could be offered to primary care offices that are in proximity to dementia specialty clinics where the trials are ongoing (e.g., ACTC-DS sites). If positive, the doctor could refer the patient to the dementia specialty clinic for follow-up analyses. This approach would be cost effective and scalable, which would then also ensure more rapid enrollment into the trials.

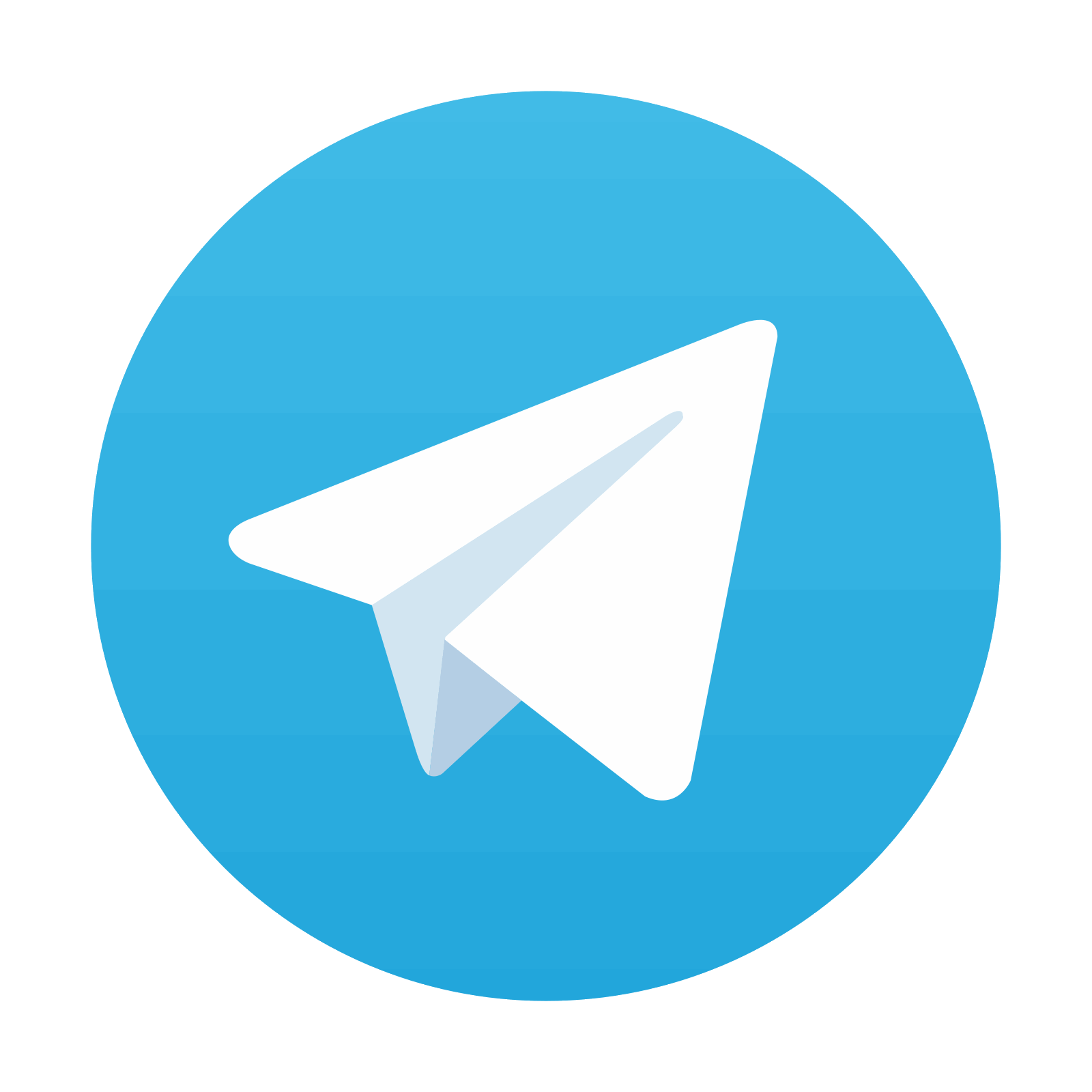
Stay updated, free articles. Join our Telegram channel

Full access? Get Clinical Tree
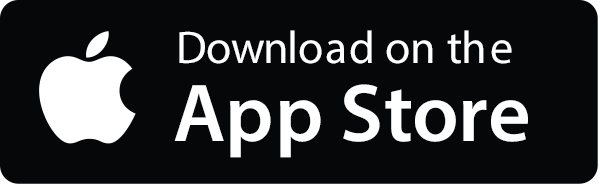
