10.1 Introduction
Focal epilepsy was in 2005 redefined as a disease where seizures occur as a result of abnormal brain network activity in confined areas of the brain.1 This is a clear shift from more traditional definitions of focal seizures as arising from excessive activity or synchrony within a population of neurons (see Fisher et al.2 for a good discussion on this topic dating back to Hughlings Jackson’s work on epilepsy and seizures). In parallel with the increased interest in epilepsy and networks, our ability to analyze brain imaging data as complex networks has increased exponentially through connectomics.3 This is particularly reflected in number of peer-reviewed articles in this research field over the last five years (Figure 10.1). Connectomics has now evolved into a research field with a clear final aim of systematically studying functional and structural connections of the brain by means of innovative imaging techniques.4, 5 The maps, called connectomes, can be constructed on a variety of spatial levels, including single neurons (microscale), populations of neurons (mesoscale), and the whole brain (macroscale). In addition to analyzing the structural wiring of the brain, connectomics allows functional connectivity patterns to be mapped, enabling inferences about information transfer within the brain.
Figure 10.1. An overview of peer-reviewed publications with the keywords “connectome” and “epilepsy networks” between 2005 and 2015.
The purpose of this chapter is to highlight the insight connectomics has offered into focal epilepsy as a disease that affects brain networks, and it is outside its scope to provide a comprehensive methodological overview of connectomics and its associated mathematical measures (including graph theory analyses). We refer the reader to Bullmore and Sporns6 and Rubinov and Sporns7 for thorough methodological overviews on connectomics.
10.2 Interictal Brain Network Findings in Focal Epilepsy
Several studies have in the last few years attempted to apply brain network metrics to patients with focal epilepsy using fMRI, diffusion MRI, and EEG, during the interictal state.8–15 Van Diessen et al.16 conducted a meta-analysis of brain network studies used in the focal epilepsies. They focused on two of the most commonly applied network measures, (1) clustering coefficient and (2) shortest path length. These two measures come from graph theory, which aims to quantify topological features of networks.17 In brief, clustering coefficient counts the number of connected triangular nodes in a network, and is an estimate of network “cliquiness” (Figure 10.2, left). The shortest path length, on the other hand, counts the minimum number of links (i.e., connections) that connect two nodes, and is an index of efficient information transfer in the network (Figure 10.2, right). Brain networks have high clustering coefficient and low path length. This is associated with the well-known “small-world” network topology that is able to support both network segregation and integration (see Table 10.1 for a brief summary).18 The result of the aforementioned meta-analysis demonstrated that the brains of focal epilepsy patients had significantly higher clustering coefficient and shortest path length, compared to controls. This finding accords with a regular network topology where brain nodes may isolate themselves from the wider networks, thus becoming less globally integrated. There is also evidence that this network configuration become more “extreme” during days with a high seizure load. Otte et al.19 used task-free fMRI in rats with extratemporal focal epilepsy and reported that rats displayed more regularized brain network topology via increased clustering coefficient and shortest path length during days when they experienced an excessive amount of seizures. Thus, increased segregation and decreased integration of networks may be related to severity as well as frequency of seizures.
Figure 10.2. Two simple networks with high clustering coefficient (red node on the left) and low shortest path length (the links connecting the two red nodes on the right). These are features of an efficient network topology, often termed “small-worldness.”
Table 10.1 Glossary of Brain Network Analysis
Connectomics | A new scientific field aiming to map the brain’s functional and structural substrates |
Graph theory | An established mathematical field on the study of network topology |
Connectome | Representation of brain regions (nodes) and their interconnections (edges) |
Clustering coefficient | Interconnectedness of (topologically) local brain nodes |
Shortest path length | Number of shortest steps between two nodes—i.e., likely to represent efficiency in information transfer between nodes |
Small-world network | A network with high segregation and integration among its nodes—high clustering coefficient and low shortest path length |
Regular network | A network with high network segregation and low network integration—high clustering coefficient and high shortest path length |
Random network | A network with low network segregation and high network integration—low clustering coefficient and low shortest path length |
These studies largely investigated whole-brain network function in focal epilepsy. A question that naturally arises at this point is whether network regularity is a brain-wide effect or is an exclusive feature of the seizure focus and/or surrounding epileptic brain regions. In an attempt to answer this, we calculated clustering coefficient and shortest path length using task-free fMRI data from within the lesional cortex in patients with polymicrogyria,20 a malformation of cortical development resulting in widespread cortical lesions often associated with focal seizures.21 Specifically, we compared clustering coefficient and shortest path length between the abnormal polymicrogyric cortex and the unaffected contralateral homologue. We observed that brain network regularity (increased clustering coefficient and shortest path length) was significantly higher within polymicrogyric lesions compared to the contralateral normal homologue. This finding suggests that the abnormal cortex itself may drive widespread network abnormalities and is in line with epilepsy syndromes where a complete resection of a small (and highly epileptogenic) lesion can reverse seizures and cognitive disturbances.22, 23 Further evidence for this is demonstrated by a series of electrophysiology studies investigating dynamic seizure networks.
10.3 Peri-ictal Brain Network Findings in Focal Epilepsy
Le Van Quyen et al.24 outlined a (preconnectomics) model of how brain networks may change before, during, and after a focal seizure. In this model, the brain undergoes drastic topological changes during the preictal state where the local seizure network (nodes within and proximate to the seizure focus) isolates itself through inhibitory processes. As focal seizures progress, the local seizure network gradually becomes more integrated with wider brain networks allowing for seizure termination.
Since the proposal of this model, several electrophysiological studies have considered time-varying changes of seizure networks during epileptic events using the clustering coefficient (network segregation) and shortest path length (network integration). Both scalp-level and invasive electrophysiological recordings have been utilized to measure peri-ictal changes of brain networks. A common finding across these studies is that brain network properties undergo temporal changes during epileptic events (interictal discharges and/or seizure). During seizure initiation and propagation, there is an increase of clustering coefficient and shortest path length (Figure 10.3, black dotted line), resembling a regularized, or isolated, network topology (see Figure 10.3, upper left network).25–28 In a network that is regularized, local clusters of neurons may isolate themselves from the rest of the network. Khambhati et al.29 describe this topological seizure configuration as “network tightening,” suggesting a mechanistic aim to contain seizure-related activity that has been brewing over a long period of time. Perhaps paradoxically, the studies presented above show a common pattern that upon termination of seizures, functional brain networks diverge their topology toward a more randomized configuration (Figure 10.3, lower left brain network). When a network enters a “random” mode, it consumes great effort and energy due to its emphasis on globally integrated (expensive) processes rather than locally segregated (cheap) processes30 and may represent a seizure termination mechanism.
Figure 10.3. Network changes between and during focal seizures: (A) Three network topologies. Upper brain = regular network; middle brain = small-world network; lower brain = random network. (B) Summary of the interictal meta-analysis by van Diessen et al.16 Patients with focal epilepsy (red circle) display a brain network topology skewed toward network regularity compared to healthy controls (blue circle). (C) Summary of dynamic network activity during focal seizures, based on electrophysiological data.
In sum, these findings broadly support Le Van Quyen’s seizure network model and show that epileptogenic brain networks display characteristic abnormal behavior. Network regularity appears to be a promising brain configuration that may explain focal epilepsy phenotypes—whether these represent mechanisms to protect the brain against recurrent seizures, inhibit seizure spread or instigate seizures, remain uncertain.
10.4 “Common” Brain Networks in Focal Epilepsy?
The studies presented in the two previous sections raise an important question. Do patients with heterogeneous focal epilepsy (in terms of the seizure focus and symptomatology) have brain network abnormalities in common? In an attempt to answer this question, a few studies have used simultaneous EEG and fMRI. The first article to report “common” brain regions amongst focal epilepsy patients was Laufs et al.,31 followed by studies from Fahoum et al.32 and Flanagan et al.33 These studies all found common brain regions on fMRI that were activated during epileptiform discharges, despite having variable seizure foci including both temporal lobe and neocortical epilepsy. One brain area found to be common to focal epilepsies was the ipsilateral piriform cortex. This cortex is a deep brain region involved in olfactory processing, and has been demonstrated to be highly epileptogenic in animal kindling models of epilepsy.34 Other potential common brain regions of focal epilepsy include the thalamus, insula, cerebellum, and cingulate cortex.32 We have also replicated these findings using fMRI connectivity measures in patients with extratemporal focal epilepsy.35 It is therefore tempting to postulate that brain regions including the piriform cortex, cingulate cortex, cerebellum, and frontoinsular regions compose a common epilepsy network in focal epilepsy.
10.5 Future Directions I: Antiepileptic Drugs, Comorbidities, and Networks
A general limitation of studies summarized in this chapter is that patients are often on multiple antiepileptic drugs and have often endured seizures over a number of years before being considered for surgery. This raises a question of whether brain network abnormalities in focal epilepsy are related to commonly observed comorbidities and/or antiepileptic drug treatment.
Focal epilepsy patients often suffer from cognitive disturbances including attention problems, memory problems, depression, and anxiety.36, 37 A question regarding epilepsy and secondary behavioral problems is whether these debilitating cognitive and/or psychiatric problems exist prior to the onset of seizures, or does persistent seizure activity cause behavioral problems? Taylor et al.38 showed that patients with new-onset epilepsy display significantly more problems of memory and psychomotor speed than healthy control subjects. This finding suggests that cognitive problems are present before the onset of the first seizure. On the contrary, Thompson and Duncan39 demonstrated that patients who experience more frequent secondary generalized tonic-clonic seizures scored worse between two sessions of cognitive tests spaced ten years apart than patients with infrequent secondary generalized tonic-clonic seizures. This finding suggests that recurrent seizures result in more severe cognitive problems. These two divergent results amplify the conundrum of whether cognitive comorbidities are present before or after the first overt seizure. Keezer et al.40 have outlined several possible scenarios of comorbidities in epilepsy. A plausible theory is that seizures and comorbidities share a symbiotic relationship. That is, comorbidities may lead to worse seizures, and seizures may lead to worse comorbidities.
Antiepileptic drug treatment is also likely to impair brain network function. Jokeit et al.41 found that the extent of fMRI activation in the temporal lobes was negatively correlated with levels of carbamazepine dosage. Valporate has also been found to be associated with reduced cortical thickness of the parietal lobes,42 whereas Yasuda et al.43 demonstrated that toprimate affects cognitive brain networks (particularly the default mode network) using task-based fMRI data. Using task-free fMRI, Haneef and Chiang10 found that epilepsy patients who were on carbamazepine displayed lower brain network activity compared to epilepsy patients who were not using carbamazepine. Combined, these studies suggest that antiepileptic drugs may affect brain network structure and function.
To overcome a potential bias due to effects of psychiatric/neuropsychological comorbidities and antiepileptic drugs, we need to conduct longitudinal studies where these factors can be monitored over time.
10.6 Future Directions II: Patient-Specific Brain Network Abnormalities
A machine learning approach such as multivariate pattern analysis has in recent years received widespread attention because it allows for classification of brain network data at the level of the individual.44–47 First, multivariate pattern analysis searches for distinct brain patterns (e.g., clustering coefficient and shortest path length) between epilepsy and control groups (“training”). After training the data, we can make a single-subject inference by introducing a new subject into the analysis (“classification”). When classifying the new subject, the machine learning algorithm judges whether its brain patterns resemble the “epilepsy” or “control” group. The most common way to quantify the accuracy of such a classifier is via a leave-one-out cross-validation scheme. This cross-validation scheme excludes one subject and trains a model based on the remainder of the subjects. This is repeated, leaving out a different subject each time, until every subject has been excluded once. The final classifier accuracy is estimated based on the single-subject inferences achieved during the cross-validation procedure, i.e., percentage of subjects correctly classified (see Cristianini and Shawe-Taylor48 and Rasmussen et al.49 for methodological overviews). Some studies have already applied machine learning algorithms to epilepsy and neuroimaging data (refer to Bernhardt et al.,50 Pedersen et al.,35, 51 and Yang et al.52 for examples). However, its full validity in predicting single-subject network characteristics in focal epilepsy remains uncertain at this stage. Further work with larger and homogenous “training” cohorts is needed to test the feasibility of machine learning as a reliable way of delineating patient-specific networks in focal epilepsy.
10.7 Conclusion
Both interictal and peri-ictal focal epilepsy networks are associated with “network regularity” expressed as increased clustering coefficient and shortest path length. Future brain network studies will benefit from controlling for “nonepileptic” variables such as common comorbidities and antiepileptic drug effects. Analyzing connectomic data at the level of the individual, enabled by machine learning approaches, is also likely to become a major contributor in enhancing our understanding of focal epilepsy as a brain network disease.
Acknowledgments
We acknowledge support by the National Health and Medical Research Council (NHMRC) of Australia (628952). The Florey Institute of Neuroscience and Mental Health acknowledges the strong support from the Victorian Government and in particular the funding from the Operational Infrastructure Support Grant. GDJ is supported by an NHMRC practitioner fellowship (1060312). MP was supported by the University of Melbourne international scholarships.
References









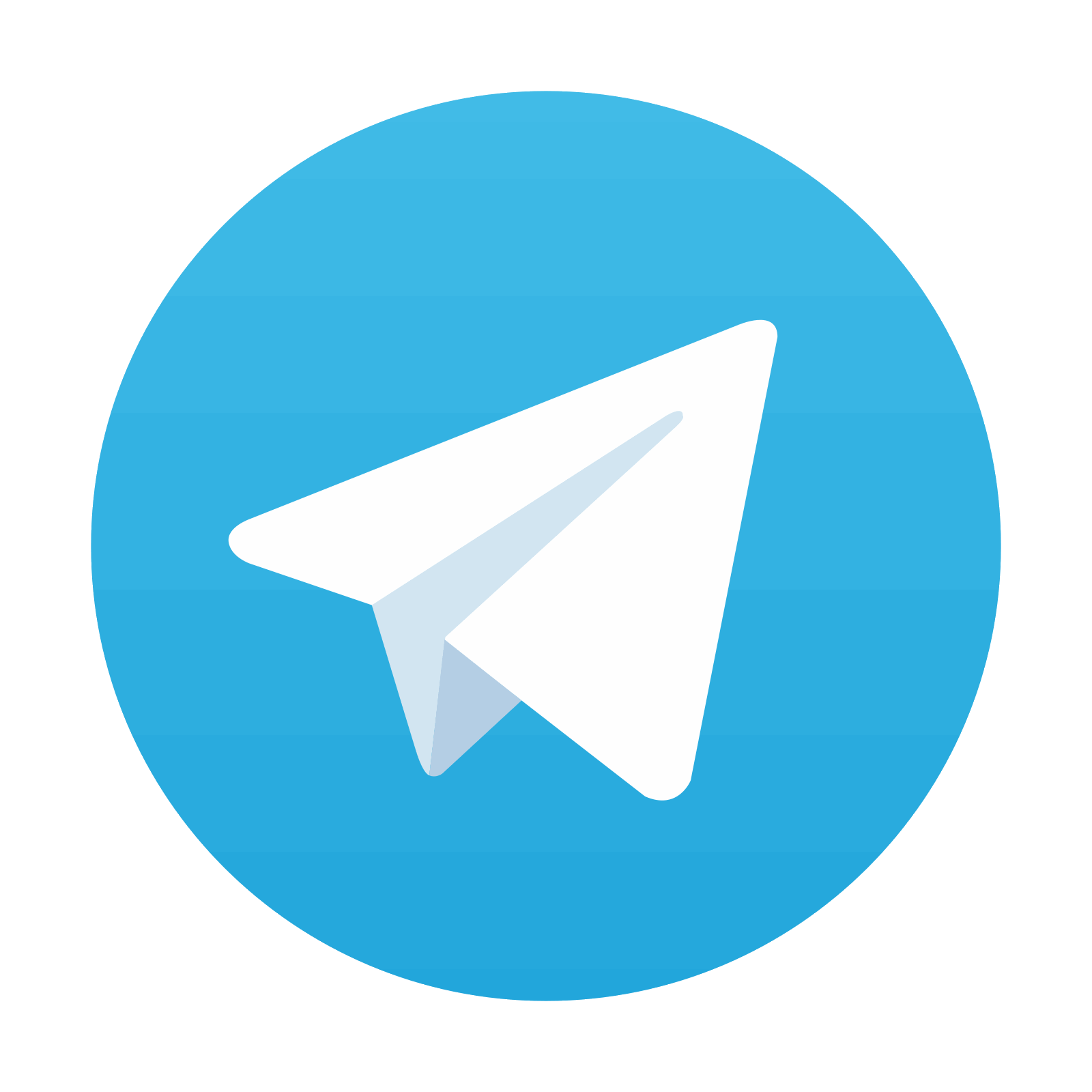
Stay updated, free articles. Join our Telegram channel

Full access? Get Clinical Tree
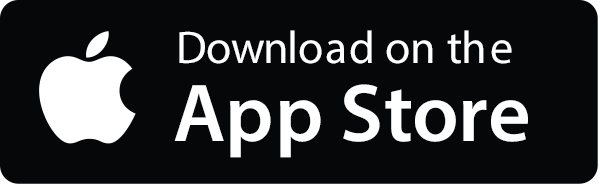

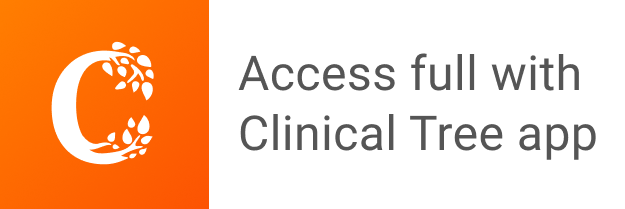