Fundamental Constraints on the Evolution of Neurons
A. Aldo Faisal and Ali Neishabouri
7.1 Introduction
Nervous systems are responsible for perceiving, integrating, and responding to complex and diverse stimuli, such as reading and typing this text. On an abstract level, our brain and a computer have to solve similar computational tasks and, thus, show similarities in their design. However, the brain’s basic building blocks are fundamentally different from those of conventional electronics—it uses neurons and synapses as computational components, which are made up of proteins instead of transistors, fat (bilipid membrane) as insulators, and salty water instead of gold or copper as a conducting core.
Processing and transmission of information in neurons is accomplished by altering the membrane potential through movement of ions. The cell membrane is largely impermeable to ions and acts as a capacitance with a finite response speed, determined by the membrane time constant. The finite response range of neurons—signals range over 100 mV in amplitude and less than 1 kHz in action potential frequency—imposes limits on the total information throughput (Stemmler & Koch, 1999). Rates of synthesis, release diffusion, and uptake of chemical transmitters also limit the performance of neural fibres.
Random fluctuations are present at all levels of nervous systems (reviewed in Faisal, Selen, & Wolpert, 2008). Ion channels are subject to thermodynamic noise that may cause their spontaneous opening or closing (Faisal, White, & Laughlin, 2005; White, Klink, Alonso, & Kay, 1998), which is called channel noise. Synaptic vesicle release, diffusion, and molecular interactions (Laughlin, 1989) are all stochastic processes. The existence of these sources of variability undermines reliable processing and transmission of information in neurons. Creation and maintenance of a nervous system is very metabolically expensive: this includes the cost of producing and maintaining neurons, their connections, and support cells (astrocytes, oligodendrocytes, and Schwann cells), to which we must add the cost of generating and propagating neural signals (Attwell & Laughlin, 2001; Harris & Attwell, 2012; Laughlin, de Ruyter van Steveninck, & Anderson, 1998). The metabolic cost of APs in the human brain alone accounts for 22% of the resting metabolic consumption (Alle, Roth, & Geiger, 2009; Laughlin, 2001; Sengupta, Stemmler, Laughlin, & Niven, 2010).
Finally, in the case of very dense circuits such as the brain, or in very small organisms, neural fibres are constrained by volume (see Niven & Farris, 2012, for a review of miniaturization of nervous systems). There is evidence that the wiring of the brain optimizes the volume occupied by axons to reduce metabolic cost and conduction delays (Wang et al. 2008). The size of axons directly interacts with all 4 physical constraints: bigger axons increase the overall volume of nervous system and have a higher associated metabolic cost, while smaller axons conduct APs slowly. Moreover, noise imposes a lower limit on the diameter of axons (Faisal et al., 2005).
How do these differences affect brain function and design? How have they channelled the evolution of the nervous systems? The brain’s building blocks are several orders of magnitude less reliable than those of computers, yet computers with the computational capability and reliability of the brain would require a small power plant while the brain completes all its function with less power than a light bulb needs to illuminate this text. The likely reason for the brain’s efficiency lies in its design: It uses circuits arranged in large, massively parallel networks and molecular components operating on the nanometer scale. With the advent of synthetic biology and current efforts to engineer living machines ab initio, it has become important not only to have a mechanistic understanding of functional and structural drivers of nervous system’s evolution but also to uncover the essential the design principles of biological “devices.”
We will focus on two fundamental constraints that apply to any form of information processing system, be it a cell, a brain or a computer: 1. Noise (random variability) and 2. Energy (metabolic demand). We will show how these two constraints are fundamentally limited by the basic biophysical properties of the brain’s building blocks (protein, fats, and salty water) and link nervous system structure to function. The understanding of the interdependence of information (and its “nemesis” noise) and energy has profoundly influenced the development of efficient telecommunication systems and computers. However, in biology and neuroscience this fundamental relationship between information and energy is little investigated, although it bears important implications for understanding evolution (see Figure 7.1).

Figure 7.1 Basic Constraints on the Design of Neural Circuits.
Energy, noise, speed, and volume are linked to each other by basic biophysical principles. For example, reducing the diameter of an axon will decrease its volume and its metabolic cost. However, smaller axons are noisier, and conduct action potentials more slowly.
7.2 Noise as a Fundamental Limit on Axon Diameter
When Adrian began to record from neurons in the 1920s, he observed that neural responses were highly variable across identical stimulation trials and only the average response could be related to the stimulus (Adrian, 1928; Adrian & Matthews, 1927). Biology viewed this variable nature of neuronal signaling as “variability”, engineers called it “noise.” The two terms are closely related, but as we shall see imply two very different approaches to think about the brain—one operating at the systems level, the other operating at the molecular level. On the one side, the healthy brain functions efficiently and reliably, as we routinely experience ourselves. On the other side, variability is a reflection of the complexity of the nervous system.
In the classical view of neurobiology it is implicitly assumed that averaging large numbers of such small stochastic elements effectively wipes out the randomness of individual elements at the level of neurons and neural circuits. This assumption however requires careful consideration for two reasons:
- Neurons perform highly nonlinear operations involving high‐gain amplification and positive feedback. Therefore, small biochemical and electrochemical fluctuations of a random nature can significantly change whole cell responses.
- Many neuronal structures are very small. This implies that they are sensitive to (and require only) a relatively small number of discrete signaling molecules to affect the whole. These molecules, such as voltage‐gated ion channels or neurotransmitters, are invariably subject to thermodynamic fluctuations and hence their behavior will have a stochastic component that may affect whole cell behavior.
All forms of signaling in the brain are in the end controlled by proteins embedded in the cell membrane or within the cell. These proteins operate with an element of randomness due to thermodynamic fluctuations, which can have important consequences in terms of how the nervous system is designed and functions. This suggests that unpredictable random variability (noise) produced by thermodynamic mechanisms (e.g., diffusion of signaling molecules) or quantum mechanisms (e.g., photon absorption in vision) at the molecular level can have a deep and lasting influence on variability present at the system level. We, on the other hand, have come to expect a near deterministic experience of our nervous system. We do not expect to see or hear anything unless there is something to be seen or heard. We generally seem to see the same thing if we look twice. This deterministic experience implies that the design principles of the brain must mitigate or even exploit the constraints set by noise and other biophysical factors.
This prowess can only be fully appreciated when we realize that noise cannot be removed from a signal once it has been added to it. Since signals can easily be lost, and noise easily added, this sets a one‐sided limit on how well information can be represented. Noise diminishes the capacity to receive, process, and direct information, the key tasks of the brain. Investing in the brain’s design can reduce the effects of noise, but this investment often increases energetic requirements, which is likely to be evolutionary unfavourable.
7.3 Molecular Noise as a Fundamental Limit on Wiring Density
In our brain the action potential (AP) is used as the basic signal for communication in neural networks. The AP is carried by the spread of membrane depolarization along the membrane and is mediated by voltage‐gated ion channels: The depolarization is (re)generated by nonlinear voltage‐gated sodium conductances acting as positive feedback amplifiers, and is terminated by leak conductances and voltage‐gated potassium channels that repolarize the membrane (Hille, 2001; Weiss, 1997).
How small can neurons or axons be made before channel noise effects disrupt action potential signaling? This is clearly a neuronal design question that had no systematic answer as recently as a few years ago—anatomists had previously shown that axons as fine as 0.08 µm to 0.1 µm are commonly found in the central nervous system. Hille (1970) suggested that in very fine axons the opening of a few sodium channels could generate an AP. Detailed theoretical and simulations (Faisal et al., 2005) showed that spontaneous opening of sodium channels can, in theory, trigger random action potentials below a critical axon diameter of 0.15 µm to 0.2 µm diameter.
This is because at these diameters the input resistance of a sodium channel is comparable to the input resistance of the axon. The single, persistent opening of a single sodium channel can therefore depolarize the axon membrane to threshold. Below this diameter, the rate at which randomly generated APs appear increases exponentially as diameter decreases (see Figure 7.2A). This will disrupt signaling in axons below a limiting diameter of about 0.1 µm, as random action potentials cannot be distinguished from signal‐carrying action potentials. This limit is robust with respect to parameter variation around two contrasting axon models, mammalian cortical axon collaterals and the invertebrate squid axon. This robustness shows that the limit is mainly set by the order of magnitude of the properties of ubiquitous cellular components, conserved across neurons of different species. The occurrence of random action potentials (RAP) and the exponential increase in RAP rate as diameter decreases is an inescapable consequence of the AP mechanism. The stochasticity of the system becomes critical when its inherent randomness makes it operationally infeasible, that is, when random APs become as common as evoked APs.


Figure 7.2 Noise Limits the Miniaturization of Unmyelinated Axons.
(A) SAP rate versus axon diameter for a pyramidal cell axon collateral (open triangles, 23 °C; closed triangles, 37 °C) and a squid axon (circle) of 1 mm length. Spontaneous AP rate increases sharply below a critical diameter of 0.15 µm to 0.2 µm. (Inset) Semilogarithmic plot of the data shows the exponential character of the dependence of spontaneous AP rate on diameter below the critical diameter. The arrow highlights how little changing the signal AP rate from 4 to 20 Hz affects the limiting diameter (the diameter at which SAP rate equals half the signal AP rate). (B) Scale drawing illustrating how essential components can be packed into the cross‐section of an axon of 50 nm diameter (see text for details). The unfilled circle illustrates the finest known AP‐conducting axons, whose diameter, 100 nm, corresponds to the channel‐noise limit derived in this study. (C) Diameters of fine AP‐conducting axons in a wide range of species and tissues (Berthold & Rydmark, 1978; Braitenberg & Schüz, 1998; Easton, 1971; Guillery, Feig, & van Lieshout, 2001; Heck & Sultan, 2002; Hsu, Tsukamoto, Smith, & Sterling, 1998; Keynes & Ritchie, 1965; Olivares, Montiel, & Aboitiz, 2001; Shepherd & Harris 1998; Small and Pfenninger, 1984; Sugimoto, Fukuda, & Wakakuwa, 1984; Williams & Chalupa, 1983; Wozniak & O’Rahilly, 1981). The finest AP‐conducting axons reach the limiting diameter of 0:1 µm (dotted line); the few exceptions are developing fibers of 0:08 µm diameter (arrowhead).
Adapted from Faisal et al. 2005. Reproduced with permission of Elsevier publishing.
7.4 Higher Body Temperature, Lower Neuronal Noise: Why Warmer Brains Are More Reliable
Temperature is not only a key factor in determining the speed of biochemical reactions such as ion channel gating; it also controls the amount of ion channel variability (Faisal & Matheson, 2000; Faisal et al., 2005). While commonly overlooked, temperature—and via its effects on ion channel kinetics, channel noise—can vary greatly across the nervous system: Cold‐blooded insects can warm up their body to over 40 °C prior to taking flight, while human extremities and the sensory and motor neurons therein can be exposed to temperature differences of up to 10 °C or more between their dendrites, cell bodies, and axon terminals as they span from cold extremities to the warmer spinal cord.
The rate of RAPs triggered by channels noise counterintuitively decreases as temperature increases—just the opposite of what one would expect from electrical Johnston noise. Stochastic simulations (Faisal et al., 2005) showed that RAP rate is inversely temperature dependent in the cortical pyramidal cell and the squid axon which operated at 36 °C and 6.3 °C, respectively. Increasing temperature has a well‐known accelerating effect on ion channel kinetics. Higher temperatures speed up the movement of charged gating particles, which, in turn, decreases the time between changes of conformation, i.e., opening and closing of channels. This means that a spontaneously opened channel will spend less time in the “open” state as the temperature increases. This reduction of the duration of spontaneous depolarizing currents means that the membrane is less likely to reach AP threshold (this effect prevails over the increased rate of spontaneous channel openings). In other words, increasing temperature shifts channel noise to higher frequencies where it is attenuated by the low‐pass characteristics of the axon (Faisal et al., 2005; Steinmetz, Manwani, Koch, London, & Segev, 2000). This may suggest that increasing temperature allowed homeothermic animals, such as mammals, to develop more reliable, smaller, more densely connected and thus faster neural circuits.
7.5 Channel Noise and Channelopathies
Channelopathies are disorders due to abnormalities in ion channels. Generalized epilepsy with febrile seizures, a condition without clear trigger, is, for instance, associated with a mutation of the b4 subunit in sodium channels, while benign familial neonatal epilepsy is associated with a reduced expression of slow, KCNQ‐type K channels. Intriguingly, in a simulation study of action potential initiation under different channelopathies, we found that these altered channel kinetics did not always result in a change of the neuron’s average behavior, yet produced clinical symptoms.
We advance the following hypothesis that could provide a general framework of explanation and highlight a novel aspect of “stochastic diseases”: Channelopathies may cause ion channels to display the same average behavior, but greatly change the afflicted channel’s trial‐to‐trial variability around this average behavior. Wild‐type ion channel fluctuations can cause random, spontaneous APs even in absence of synaptic input (while deterministic models of the same channels do not produce any spontaneous activity). Should channelopathies alter channel kinetics in such a way so as to make ion channels more unreliable (as suggested by our preliminary stochastic simulations of an NaV 1.2 sodium channel mutant), then we would expect to see a greatly increased rate of spontaneous neuronal activity in much larger nerves and neurons.
The altered probabilistic behavior of ion channels—whether in absence of or in addition to changes to the average behavior of the channel—has previously neglected implications for understanding epilepsy and neuropathic as the result of increased unwanted neuronal activity. One way to test this hypothesis is to screen the rapidly growing literature on channelopathies and the relevant ion channel kinetics. These data are typically described as Hodgkin–Huxley‐type deterministic kinetics, but can be easily converted into a stochastic Markov‐model‐type kinetics.
Such studies should investigate the diversity (including channelopathies) of voltage‐gated Na+ and K+ channels from a functional perspective and ultimately in their evolutionary context. While the phylogeny of ion channels has been extensively studied in genetic and molecular terms, a systematic analysis of the functional implications of ion channel variations is so far missing. Taking the view that the primary role of an axon is to transmit information, one can assess the role of an axon’s component from a functional perspective. Thus, an ion channel can be thought of as representing a particular choice in trade‐offs between the 4 basic constraints on information processing.
7.6 Are There Other Biophysical Limits to Axon Size?
How small can a functioning axon be constructed, given the finite size of its individual components? Faisal et al. (2005) showed, using a volume exclusion argument, that it is possible to construct axons much finer than 0.1 µm diameter (see Figure 7.2). Neural membrane (5 nm thickness) can be bent to form axons of 30 nm diameter because it also forms spherical synaptic vesicles of that diameter. A few essential molecular components are required to fit inside the axon; these include an actin felt work (7 nm thick) to support membrane shape, the supporting cytoskeleton (a microtubule of 23 nm diameter), the intracellular domains of ion channels and pumps (intruding 5 nm to 7 nm), and kinesin motor proteins (10 nm length) that transport vesicles (30 nm diameter) and essential materials (<30 nm diameter). Adding up the cross‐sectional areas shows that it is possible to pack these components into axons as fine as 0.06 µm (60 nm). Indeed, the finest known neurites, those of amacrine cells in Drosophila laminae, are about 0.05 µm in diameter, contain microtubules, and connect to extensive dendritic arbours but do not transmit APs. The fact that the smallest known AP‐conducting axons are about twice as large as the steric limit to axon diameter (0.1 µm cf. 0.06 µm, see Figure 7.2B), whereas electrically passive axons reach the physical limit, supports our argument that channel noise limits the diameter of AP‐conducting axons to about 0.1 µm.
Furthermore, other molecular limits to axon diameter are well below the noise‐limited diameter of 0.1 µm, thus AP‐conducting axons finer than 0.1 µm could, in theory, exist. Yet anatomical data across many species, invertebrate and vertebrate, including extremely small insects and large mammals, shows an identical lower limit of diameter for AP‐conducting axons of 0.1 µm. This suggests that channel noise limits axon diameter, and thus the wiring density of the central nervous system, and therefore ultimately the size of the cortex. Curiously, the anatomical literature (see Figure 7.2C) demonstrated a common lower value for the diameter of axons for over 30 years, yet this was not noticed till a systems biology view on the study on stochastic limits to cell size prompted a search for the smallest known axon diameters (Faisal et al., 2005).
7.7 Is Behavioral Variability the Cause of Molecular Noise?
Neurons are variable, in that we observe both irregular spontaneous activity (activity that is not related in any obvious way to external stimulation) and trial‐to‐trial variations in neuronal responses to repeated identical stimuli—and both are often considered signs of “noise” (Shadlen & Newsome, 1995; Softky & Koch, 1993). Whether this neuronal trial‐to‐trial variability is indeed just noise (defined in the following as individually unpredictable, random events that corrupt signals), a result of the brain being too complex to control the conditions across trials (e.g., the organisms may become increasingly hungry or tired across trials), or rather the reflection of a highly efficient way of coding information, cannot easily be answered. In fact, being able to decide whether we are measuring the neuronal activity that is underlying the logical reasoning and not just meaningless noise is a fundamental problem in neuroscience (Rieke, Warland, de Ruyter van Steveninck, & Bialek, 1997). There are multiple sources contributing to neuronal trial‐to‐trial variability: deterministic ones, such as changes of internal states of neurons and networks, as well as stochastic ones, noise inside and across neurons (Faisal et al., 2008; White, Rubinstein, & Kay, 2000). To what extent each of these sources makes up the total observed trial‐to‐trial variability remains unclear.
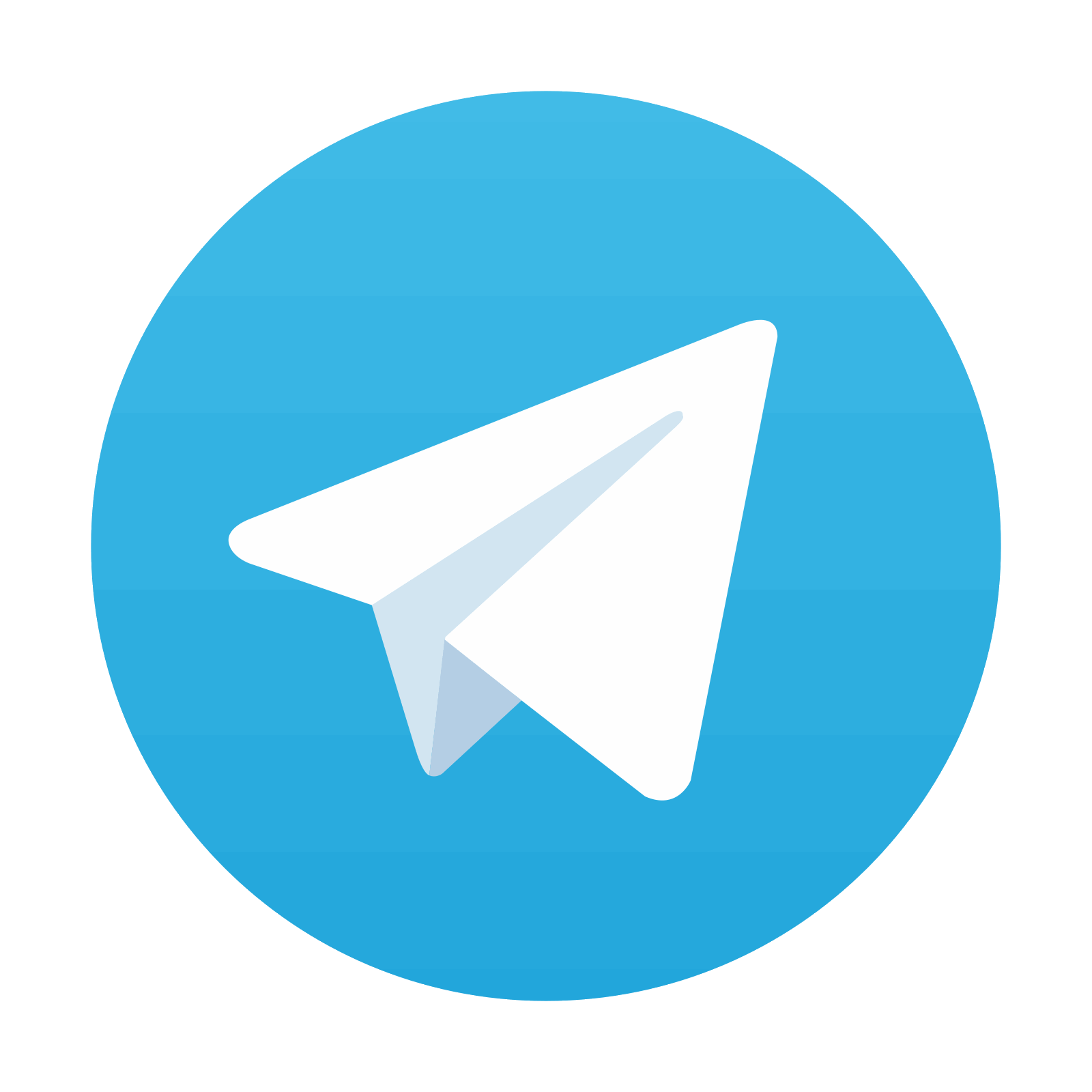
Stay updated, free articles. Join our Telegram channel

Full access? Get Clinical Tree
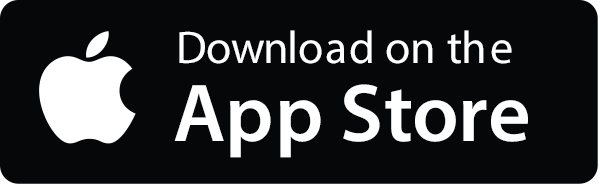
