Digital electroencephalography (EEG) is the simple recording and interpreting of routine EEG using computer-based technology. Quantitative electroencephalography (QEEG) is the processing of digital EEG with various algorithms and displays. There are many types of QEEG. The EEG can be searched for spikes and seizures. Frequency content can be calculated and compared to prior or normative values. Topographic scalp maps or three-dimensional brain maps can display EEG features, a process often labeled EEG brain mapping.
EEG frequency analysis has been studied for nearly 80 years, and phase and coherence have been studied for more than 50 years. Technical development was slowed by the substantial computer processing required. A routine 20-minute EEG is more than 10 megabytes in size. In the 1970s, there was great enthusiasm for building a computer that could “analyze the EEG” and generate a draft report for each individual clinical record. This goal proved unfeasible. Interpretation still requires expert input from a knowledgeable user. QEEG data can be used to aid in assessing the EEG results. Quantitative analysis acts as a ruler for measuring specific EEG features, which are then presented to a knowledgeable user for further interpretation.
Techniques
EEG Acquisition and Storage
Routine clinical digital EEG commonly involves recording from all 21 of the electrode locations in the 10–20 system, as well as from several extra artifact-control channels. Digital equipment can record much larger numbers of channels: for example, on epilepsy units, where systems can record from hundreds of channels. Digital EEGs usually are recorded in a referential format with open filter settings, allowing for subsequent review in any montage and with different filter settings.
Digital EEG is stored in public or commercial data formats. The most common public data format is the European data format (edf). Each instrumentation company has its own proprietary format. Some third-party vendors have generic reading stations able to read records from any of several commercial or public formats.
Storage is on digital disks or similar mass storage devices. One problem now is the eventual obsolescence of the data formats and reading hardware. There is no immediate solution for this, and major difficulty may occur with record retrieval in future years. Another problem is reading records run elsewhere on equipment different from that in one’s own EEG laboratory. Some companies facilitate such remote reading by providing special reading software that is copied onto discs along with copies of EEGs to be sent elsewhere. This works well because programs often run well on generic office computers.
Display Techniques
Montage reconstruction can be accomplished when the EEG has been recorded and stored in a referential montage. The EEG can be played back in any bipolar or referential montage as long as all needed electrode sites were in the original recording. This technique was developed and used in clinical patient care originally by Williams and Nuwer. Reconstruction is accomplished by subtracting referential channels from each other.
Data are acquired with open filter settings. These settings are adequate as long as the data are not contaminated heavily by artifact. Filter settings can be adjusted during replay to a more restricted setting. For example, a record can be made with filter settings at 0.1 and 100 Hz, and later reviewed at 1.0 and 70 Hz. Similarly, the digital EEG can be replayed with a variety of “paper speeds” or screen compression factors. An interesting segment of record can be replayed on an expanded time scale to look at the timing and phase reversal of spikes and sharp waves in greater detail.
Topographic maps are a controversial way to display some EEG features. EEG voltage or other features are plotted on a scalp map with contour lines or color coding to identify similarly valued regions. Use of color adds an aesthetic touch.
Such EEG brain maps superficially resemble axial cuts of a computed tomography (CT) or magnetic resonance imaging (MRI) scan but, in fact, they differ in a fundamental way. Topographic maps actually represent very few real data points, typically equal to the number of scalp recording electrodes. The remainder of the map, more than 99 percent of the pixels, is nothing more than an interpolation. The interpolation provides no new real data. This is the opposite of the situation with MRI or CT, which has very little redundancy and for which each display pixel corresponds to real data. Color on EEG topographic maps can be helpful or misleading. Sometimes, it points out small differences in data that might otherwise have been overlooked. On other occasions, maps overemphasize meaningless differences.
Low-resolution brain electromagnetic tomography (LORETA) estimates simple EEG sources at superficial or deep sites. Extrapolations from scalp sources suggest the most likely locations of epileptic spike dipoles or frequency component generators. The results are displayed on simulated cerebral topography. These displays more closely resemble CT scan or MR images, but again the data points are mostly extrapolations. The number of actual independent data points underlying LORETA images is the number of scalp electrodes used to record them. The calculations assume simple models for generators, whereas many actual generators may be broad, diffuse, or multifocal.
Analysis Techniques
Many types of analytic and display techniques are available for digital EEG and QEEG. Epileptiform spikes and sharp waves, periodic lateralized epileptiform discharges (PLEDs), triphasic waves, and other transients can be viewed most simply on the routine digital EEG. Dipole source localization can aid in estimating generator locations.
Artifacts can be identified best on the routine digital EEG. Artifact identification channels should be run along with the scalp acquisition channels. Digital removal or attenuation of certain artifacts has achieved mixed success through advanced processing techniques.
Event detectors can aid in identifying epileptic spikes or subclinical seizures, especially those in long recordings. Ambulatory EEG automated systems can scan 24- to 72-hour EEG recordings in this way. Candidate events are detected and presented to a reader for interpretation. False-positive identifications are common.
Frequency analysis involves calculating the amount of EEG in each major frequency band (e.g., the alpha band). Sometimes even smaller bands are used, down to a fraction of a cycle per second. The amount of EEG in each frequency band then is displayed in a numerical table or on a topographic scalp map. Frequencies are usually analyzed for alert subjects but are also used in surgical and critical care monitoring.
The frequency data may be presented as either absolute or relative amounts, and scaled as proportional to either the EEG voltage (amplitude) or the power . Power is the square of the voltage, often referred to as the power spectrum . Absolute voltage scaling corresponds to the EEG amplitudes as observed visually. EEG in each voltage amplitude scaled frequency band is calculated with a root-mean-square (RMS) algorithm, which makes the computer-derived RMS amplitude appear one-third as big as the usual peak-to-peak measurement used in EEG visual analysis ( Fig. 8-1 ). Relative voltage or relative power scaling also is used commonly. In relative scaling, the amount of EEG in each frequency band is divided by the total voltage or power across all EEG bands for that electrode site.The amount of EEG across all frequency bands totals 100 percent at each site. Such relative EEG values are much less variable than absolute EEG values among the general population. Many systems can display EEG frequency content in both ways.

More complex algorithms are available. A commonly used technique is the subtraction of left from right hemisphere activity, resulting in a display or calculation of the asymmetry between the hemispheres. This asymmetry helps to emphasize subtle focal EEG features; however, it makes an asymmetric feature appear farther from the midsagittal plane than it really is. Coherence is the tendency of EEG in two different channels to rise and fall in synchrony. Generally, it is calculated within specific frequency bands. If 20 scalp channels are recorded, there can be 20 × 19 (i.e., 380) individual coherence measurements within each frequency band. Sometimes, this is simplified by looking only at the coherence between homologous channels over the two hemispheres or between frontal-posterior pairs of channels in the same hemisphere. Coherence is still mainly a research tool.
Phase determinations measure which of two coherent channels leads or lags the other. An event or rhythmic activity may be seen earlier in one channel, which is then said to lead the other. Phase relationships are often stated in degrees of angle that correspond to a complete sinusoidal cycle at a particular frequency.
Multiparametric analysis considers many separate EEG features together. Such analyses include discriminant analysis . Dozens or hundreds of EEG features are entered into a predetermined formula. Such a complex calculation can be tuned to look for specific useful traits in EEG signals. Investigators have tried such complex techniques to “diagnose” specific diseases. Some such discriminant features are purported to diagnose specific diseases, including dementia, depression, head injury, and psychiatric and neurobehavioral disorders. These diagnostic discriminants remain a highly controversial part of QEEG. Some groups believe strongly in the validity of these techniques, whereas other groups believe that these techniques have not been validated sufficiently yet to allow their use in routine clinical practice.
Statistical techniques have been applied to determine whether individual simple EEG features are “within normal limits” using the z-score method. This assumes that the distribution of a particular EEG feature forms a gaussian or normal bell-shaped curve when sampled in a large population of normal control subjects. The value for an individual patient is compared to this bell-shaped normative distribution with z-score statistics, measuring how much that individual differs from the control group mean. This difference usually is expressed in terms of standard deviations. A patient’s EEG feature scored as z = 2.0 (i.e., 2.0 standard deviations above the normative group mean) has a value greater than that seen in 95 percent of normal control subjects. Such z-score techniques can help to highlight areas in which an individual patient differs substantially from an age-matched control group. However, interpretation becomes difficult when a very large number of z-score values are assessed, because chance alone will cause some z-scores to be very high. EEG artifacts, other technical problems, and confounding clinical situations can also cause high z-scores. Even if all possible sources of error are ruled out, statistical “abnormality” does not necessarily mean physiologic abnormality. Therefore z-scores never should be interpreted by themselves, but only in the context of all the other available EEG data.
Problems
Simple paperless digital EEG recordings can be interpreted in a manner analogous to the interpretation of traditional paper EEGs. Problems are more difficult for the more advanced QEEG techniques. Topographic mapping, frequency analysis, discriminant analysis, and large-scale statistical analysis techniques are often confounded by artifacts, problematic clinical factors, and overinterpretation of statistical variations.
Artifacts
Many artifacts are present in routine digital EEGs. They show up in various, sometimes subtle or confusing ways for advanced QEEG analysis. Artifacts showing up in new and unusual ways make difficult the electroencephalographer’s task of interpreting artifact-contaminated data. Some artifacts in QEEG show up in interesting ways ( Fig. 8-2 ).

The only acceptable way of dealing with artifacts is to follow the EEG data carefully through the several steps of the analysis process. A clinical reader must be able expertly to identify and interpret the EEG tracings that form the basis of any analytic QEEG process. Only by understanding such EEG tracings thoroughly can the reader hope to understand the artifacts and problems that occur in any advanced analysis based on those data.
There is widespread misunderstanding about the use of automatic “artifact rejection” in QEEG processing. Many users have the mistaken impression that automated digital artifact screening techniques are able to eliminate artifacts from the EEG record, so that the computer-based frequency analysis and topographic maps are free from artifact contamination. Nothing could be further from the truth. Artifact identification techniques are primitive and often identify only high-amplitude transients such as large muscle artifact or eye-blinks. Other kinds of artifacts are detected less easily. Again, the lesson is clear: a reader must evaluate both the EEG tracings and analyzed data together to understand the results of the advanced EEG analysis.
Confounding Clinical Factors
A variety of clinical situations can interfere with QEEG analyses, especially those using normative databases. Even drowsiness can interfere with normative EEG comparison techniques because the normal control subjects were fully awake. Statistical normative EEG analysis in a drowsy subject therefore is very “abnormal.” Medication can affect the EEG; normal control groups are generally medication-free. Other confounding clinical problems include fluid shunting, nystagmus, skull defects, and other problems with the scalp or skull that may not affect brain function. These factors can alter topographic maps, frequency analyses, and statistical analyses substantially, even though they represent no actual cerebral impairment.
The EEG contains phenomena that are of dubious clinical significance: the so-called normal variant waveforms and transients. One example is the mu rhythm in the central region, which is known to have no clear clinical significance. Yet it can affect the statistical calculations and alter the topographic maps of alpha-band activity substantially.
For the reader to be able to understand and evaluate properly the clinical problem at hand, it is necessary to analyze the whole situation, including the clinical problem, medications used, and EEG tracings, as well as the results of QEEG analysis.
Technical Diversity
EEG analytic techniques differ among vendors and among specific analytic programs. Many of these technical differences impact significantly on the results. Because of these differences, the results obtained on one commercial machine are not directly comparable to those obtained on a different machine. Some QEEG techniques are found on certain machines only and are not available on others. Some of this incompatibility is caused by proprietary restrictions or patenting of techniques. In other cases, incompatibility is caused by individual technical decisions made by specific manufacturers. Discriminant or normative comparison studies run using one vendor’s program cannot simply be applied to recordings with a different technique using a different program on a different machine. Different techniques used on different machines may each need to be validated clinically.
Statistical Problems
Complex statistical techniques (e.g., z-scores and discriminant analysis) depend on data meeting certain assumptions. In general, data from EEG tests are not distributed in the general population in a gaussian or normal bell-shaped curve.
Because of this, simple parametric statistical analysis is often in error, sometimes by substantial degrees. Such error can be corrected partially by transforming the data in certain mathematical ways. The most common transformations are log (x) and log ( <SPAN role=presentation tabIndex=0 id=MathJax-Element-1-Frame class=MathJax style="POSITION: relative" data-mathml='x(1−x)’>x(1−x)x(1−x)
x ( 1 − x )
). Even where transformation corrections are used, the reader or interpreter needs to exercise caution in accepting the results of any purely statistical analysis. In general, the reader might use statistical techniques to help point out features of possible clinical significance but should not make clinical interpretations based on statistical results alone.
Many advanced EEG analytic techniques produce enormous numbers of statistical results, sometimes hundred or thousands of z-score tests. False-positive results are common. Separating the numerous false-positive findings from true-positive findings can be difficult or impossible. This is compounded by the prevalence of artifact contamination and confounding clinical factors previously mentioned.
Use in clinical settings
Cerebrovascular Disease
QEEG is more sensitive than routine EEG for detecting cerebral hemispheric abnormalities related to cerebrovascular disease. EEG, whether quantified or routine, can detect abnormalities but cannot differentiate between kinds of pathology; nor does it have the exquisite localizing ability of CT or MRI. Nevertheless, it is inexpensive, noninvasive, reproducible, and sensitive, and it can be done in a variety of settings (e.g., the intensive care unit [ICU] or operating room), even on a continuous monitoring basis.
Routine EEGs are abnormal in about 40 to 70 percent of patients with cerebrovascular accidents (CVAs). In one study, QEEG prospectively classified 64 normal control subjects and 94 patients (54 with stroke and 40 whose symptoms cleared within a week). The classification was abnormal in 90 percent of the patients, compared with only 3 percent false-positive among the normals. This included QEEG abnormalities in 84 percent of patients whose EEGs had been read as normal in routine visual EEG assessment. In three further studies of 15 to 20 patients each, QEEG was abnormal in 85 percent of patients, but no abnormal results were found in control subjects. Routine EEG was abnormal in only one-half of these patients, and often was just diffusely abnormal or poorly localizing. However, QEEG did not do well in precise localization.
QEEG changes correspond well to regional cerebral blood flow, regional oxygen extraction, and metabolism. Relationships are particularly good for relative delta or alpha activity and the ratio of slow to fast rhythms. Several groups have found correlation coefficients of 0.67 to 0.76 relating such EEG features to metabolic parameters.
QEEG does not differentiate among types of focal cerebral pathology, e.g., ischemic infarction, intracranial hemorrhages, brain tumors, and head trauma. The kinds of EEG changes seen were similar despite obvious differences in the pathology, leading the investigators to conclude that these tests may be sensitive but are nonspecific ( Fig. 8-3 ).


Degree of slowing or loss of fast activity corresponded to National Institutes of Health Stroke Scale (NIHSS) outcome and disability at 30 days and 6 months. Degree of QEEG impairment corresponded to the stroke volume, and preserved QEEG cortical function often corresponded to subcortical lacunes as the pathology. Subcortical vascular dementia is more likely to cause a loss of fast activity, which helps to differentiate it on QEEG from Alzheimer dementia, which causes more slowing of the posterior dominant alpha mean frequency.
In most patients, the clinical and MRI findings are already sufficient, and EEG studies provide no useful additional information to help in the care of the patient. In the occasional instance, QEEG is used for patients who cannot obtain imaging.
EEG Monitoring in the Intensive Care Unit
Continuous ICU EEG monitoring has become a commonly used tool for monitoring the brain in certain critically ill patients. QEEG measurements are used to display trends of frequency content. Those trends can help to identify changes over time and can measure the variability of the frequencies. Variability is a helpful sign that is not apparent when looking at the routine EEG tracings. Variability corresponds to changes in frequency content over long time periods, in contrast to EEG reactivity , which corresponds to short-term changes often induced by environmental stimulation. Trends are commonly used in the ICU along with the routine EEG tracings. EEG tracings and trends can be viewed remotely from off-site as well as at the bedside, thereby extending the physician’s ability to evaluate the patient.
Monitoring QEEG in the ICU can detect nonconvulsive seizures or unwitnessed convulsive seizures. They are associated independently with increased midline shift and a poorer overall prognosis. The findings of continuous ICU EEG monitoring result in changes in antiepileptic drug orders in up to half of the patients monitored, based on identification of seizures on EEG. Nonconvulsive seizures or nonconvulsive status epilepticus occur in 15 to 25 percent of neurologically critically ill patients. Seizures can be identified by trending of QEEG frequency content or by automated seizure detection software, and can be tracked to measure the efficacy of treatment ( Fig 8-4 ). Because such seizures sometimes occur only every few hours, a routine 30-minute EEG may miss them. The presence of nonconvulsive seizures in these patients is an independent risk factor associated with poorer outcome and midline shifts. The seizures may provoke secondary damage in marginally compensated brains.

After subarachnoid hemorrhage, trended frequency analysis shows reduced variability and relative alpha during clinical deteriorations. Such an electrographic deterioration precedes overt clinical changes by up to 2 days before an episode of vasospasm. This early warning can prompt early intervention to prevent clinical complications.
EEG changes occur within seconds of the onset of ischemia. The QEEG trending can demonstrate dysfunction when the ischemia is severe enough to disrupt function but not so severe as to cause infarction such as that associated with decreased perfusion pressure. This immediate feedback makes QEEG trending in the ICU clinically useful for monitoring.
ICU EEG trending has been used to track improvements in ICU patients treated with mannitol or albumin for increased intracranial pressure. The time course of the changes paralleled the several-hour effectiveness of the drugs, suggesting that the QEEG trending techniques effectively monitor reproducible therapeutic effects in lowering intracranial pressure and antiedema effects.
In the neonatal ICU, a simplified version of QEEG ICU trending often is used. This measures the total integrated amplitude over minute-long portions of the monitoring for two channels. Goals are similar to those for the adult ICU: identify seizures or other complications and monitor therapy. Normal neonates and infants show age-dependent amplitude and discontinuity features that can be measured on the trending. The trending results correspond clinically to the severity of impairment as judged by other means. The trending can detect seizures. Clinical outcome at 15 months corresponds in general to the trending findings.
In surgery, similar trending can assist in measuring frequency content over the hours of a case. Traditional EEG has long been used during carotid endarterectomy to help assess brain function during carotid clamping. In turn, this can be used to help determine whether a vascular shunt is required, or whether any other surgical or anesthetic intervention is needed urgently. Quantified EEG testing can assist in detection of abnormalities with carotid clamping more often than is seen with routine EEG.
Epilepsy
Quantitative EEG is used in several ways in evaluating patients with epilepsy. Careful analysis of the scalp voltage fields of epileptiform spikes can shed light on the location of their cortical generators and contribute prognostic information. Automated paradigms may detect spikes and subclinical seizures in prolonged recordings.
Three-Dimensional Dipole Source Localization
Three-dimensional spike generator localization is difficult in routine EEG recordings. Visual inspection can lateralize a spike much better than it can localize its likely generator site. By modeling the epileptic spike generator as a single discrete point source or localized region and quantifying its scalp electrical potential field, the three-dimensional location of the likely epileptic generator can be calculated ( Fig. 8-5 ). Averaging together individual occurrences of the epileptic spike reduces background EEG noise. Spikes need to be categorized before processing, so spikes from a single putative generator source are used together, and spikes probably arising from other sites are processed separately. Least square error estimates of scalp potential maps are calculated for various proposed intracranial dipole sources. Some models assume electrical characteristics for brain, cerebrospinal fluid, skull, and scalp. Those models should be adjusted for differences in conductivity through known lesions. Larger numbers of scalp electrodes improve localization accuracy. Deep sources are localized more poorly than are superficial ones, averaging 12-mm error in some studies of deep sources. Mesial temporal discharges may not contribute to scalp recordings, so scalp recordings may be measuring regional cortical discharges. All these models have limitations both technically and in the assumptions on which they are based.

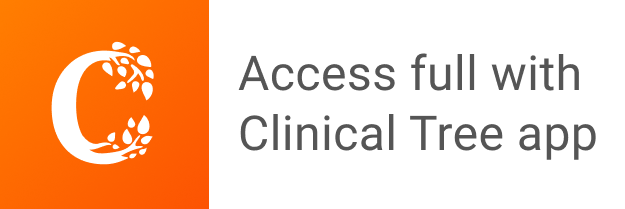