6.1 Introduction
Epilepsy, a disorder of the brain characterized by an enduring predisposition to generate epileptic seizures, affects over 50 million people worldwide. Underlying etiologies include structural, metabolic, and genetic abnormalities.1 More than one-third of these patients suffer from seizures that are resistant to antiepileptic drugs. Consequences of recurrent seizures are severe and include an increased risk of injury, psychosocial and economic impairment, and death.2, 3 In cases of drug-resistant focal onset epilepsy, when a structural abnormality is identified, its surgical resection offers patients the chance at a complete cure.4 Temporal lobe epilepsy related to mesiotemporal sclerosis (TLE) and extratemporal lobe epilepsies secondary to cortical developmental malformations, particularly focal cortical dysplasia (FCD), are the two most common drug-resistant epilepsy syndromes amenable to surgery,5 accounting for 60–80% of investigations at tertiary centers. Importantly, early identification of a lesion allows timely resective surgery, limits the long-term effects of recurrent seizures and medication, and has been shown to have positive consequences on cognitive outcome and brain development.6
Magnetic resonance imaging (MRI) provides a unique and versatile, noninvasive method for computer-aided lesion detection and brain-wide evaluation of the epileptic process (Figure 6.1). This chapter provides an overview of advanced quantitative image analysis methods used to investigate main epilepsy syndromes: TLE, neocortical extratemporal epilepsy related to cortical dysplasias, and idiopathic generalized epilepsy.
Figure 6.1. Computer-aided image analysis. Computational analysis of MRI, the most common imaging modality in epilepsy, extracts information invisible to the naked eye and provides quantitative surrogate markers of the disease process. Specifically, morphometric measurements, derived from T1-weighted images (e.g., volume and shape), provide markers of cell loss. Gradient and texture metrics derived from the analysis of T2/FLAIR intensities provide markers of gliosis. On the other hand, diffusion MRI allows quantifying increasingly sophisticated markers of tissue microstructure, such as mean diffusivity (MD), fractional anisotropy (FA), fiber density (FD), and neurite orientation and dispersion estimates (NODDI), while the analysis of BOLD signal fluctuations probes abnormal local neuronal activity (via measures such as regional homogeneity, ReHo, and amplitude of low frequency fluctuations, ALFF). Importantly, the surface-based analytical framework allows for an optimal integration of modalities and intersubject correspondence.
6.2 Computational Imaging of Temporal Lobe Epilepsy
6.2.1 Analysis of Mesiotemporal Structures
In TLE, the epileptogenic network generally encompasses variable degrees of neuronal loss and astrogliosis across hippocampal subfields, the amygdala, and the entorhinal cortex, a pathological disease hallmark referred to as mesiotemporal sclerosis (MTS).7 Based on earlier qualitative studies and recent quantitative MRI-histopathological correlation analyses of the hippocampus, it is now clearly established that cell loss and gliosis relate to atrophy and increased T2-weighted signal, respectively.8 In many patients, MTS can be visualized on MRI as noticeable hippocampal atrophy and increased T2-weighted signal. However, the visual identification of morphological and signal characteristics of the hippocampus is highly subjective and depends heavily on the experience of the reader. For at least two decades, manual MRI volumetry has been a commonly employed quantitative technique to reliably assess mesiotemporal lobe atrophy, as it has been demonstrated to be more sensitive than visual evaluation.9 This method, generally performed on high-resolution T1-weighted structural MR images, allows indeed for a reliable lateralization of the seizure focus. Volumetry of the entorhinal cortex, amygdala, and temporopolar region, as well as the thalamus, may play also roles in lateralization of the seizure focus.10 Specifically, in patients with a normal-appearing hippocampal structure imaging of visual inspection of conventional contrasts, entorhinal cortex atrophy detected by volumetry provides accurate lateralization of the seizure focus in 25% of cases.11 In sum, quantification of mesiotemporal structural damage is strongly recommended when considering epilepsy surgery in order to detect subtle atrophy or abnormal signal increases ipsilateral to the seizure focus, and to establish objectively the degree of integrity of the contralateral structures. Indeed, bilateral mesial temporal lobe atrophy raises concerns of markedly reduced chance of seizure freedom after surgery and an increased risk of memory impairment.12
From a practical perspective, manual segmentation of mesiotemporal lobe structures requires highly specialized expertise in neuroanatomy and is time-consuming, often precluding its widespread clinical use. Over the years, this challenge has motivated the development of increasingly sophisticated automatic segmentation algorithms, mostly deriving segmentations of the entire hippocampus from T1-weighted 3D volumetric acquisitions with isotropic millimetric resolution. Key design elements have been the use of a multitemplate framework to account for interindividual anatomical variability13 and patch-based techniques to compactly represent shape, anatomy, texture, and intensity.14 While most methods generally demonstrate excellent performance in healthy subjects, accuracy in patients drops significantly due in large part to the atypical shape, positioning, and size of the hippocampus secondary to incomplete unfolding (or malrotation). Indeed, about 40% of TLE patients present with malrotation, a marker of abnormal brain development characterized by a round appearance and vertical orientation of the hippocampus, which tends to be medially positioned, and a deep collateral sulcus.15 To overcome this challenge, our group has developed an automated hippocampal segmentation algorithm that integrates deformable parametric surfaces and multiple templates in a unified framework,16 which provided flexibility to model disease-related shape deformations and atrophy. Compared to other state-of-the-art algorithms, our method maintains high-level performance regardless of the presence of abnormal morphology.
Recent developments in MRI hardware together with the use of multichannel head coils offer new opportunities to appraise brain anatomy with unprecedented details. Indeed, it is now possible to obtain high-contrast 3D images at isotropic resolutions of 400–600 µm in clinically plausible scanning times,17 allowing increased visualization of the internal hippocampal architecture. However, challenges remain, particularly when attempting to separate individual CA subfields from one another, thereby compromising reliability within and across analysts, as well as automated techniques. Opting for reliability, we recently developed a segmentation protocol that divides the hippocampal formation into consistently identifiable subregions guided by intensity and morphology of the densely myelinated molecular layer, together with few geometry-based boundaries flexible to overall mesiotemporal anatomy.17 Notably, while the boundary definition of individual subfields even at high-resolution 3T MRI remains subject to debate, several automated approaches have been developed for automated delineation of hippocampal subregions.13, 18, 19 Among them, the FreeSurfer pipeline incorporates a Bayesian inference and fusion of landmarks derived from a single statistical atlas.13 Other methods propose a multitemplate framework to propagate labels through intermediate templates prior to majority voting,20 multiplying the number of segmentation candidates out of a restricted number of templates.21 Conversely, the Automatic Segmentation of Hippocampal Subfields (ASHS) algorithm22 combines label fusion with post hoc segmentation correction. These methods either operate on 2D anisotropic T2-weighted images or 3D T1-weighted with submillimetric or millimeter resolution images. Overall, segmentation accuracy seems higher when using T2-weighted MRI.22 We recently proposed SurfPatch,19 a fully automated subfield segmentation procedure operating on 3D T1-weighted images only, which combines surface-based processing with a patch-based template library. Based on an openly available dataset containing T1-weighted images with 0.6 mm isotropic resolution, SurfPatch achieved excellent accuracy (Dice index between automated and manual labels = 83–88% across subfields). Accuracy was similarly high in patients with temporal lobe epilepsy in whom structural hippocampal pathological compromise was histologically verified. Dice indices remained above 80% when the size of the training library was reduced or when segmentation was carried out based on standard millimetric resolution T1-weighted MRI. Furthermore, automatically generated subfield labels from both submillimetric and millimetric acquisitions enabled lateralizing the seizure focus with >85% accuracy in patients.
Normal whole-hippocampal volumetry represents an ongoing clinical challenge in more than 50% of TLE epilepsy surgery candidates currently labeled as “MRI-negative.” Although preliminary evidence, mainly based on manually segmentation, suggests a role for subfield volumetry for detecting subtle MTS found on histology in these cases,23 such analysis remains uncommon, motivating alternative approaches to assess the structural integrity of this pivotal mesiotemporal structure. Indeed, analytical resolution can be increased even beyond the subfield level via surface-based shape techniques to further refine the MRI correlates of hippocampal pathology. These methods have been initially applied to the outer hull of the entire hippocampus and other mesiotemporal structures, as well as the thalamus.24–27 In particular, we have developed and validated a 3D surface-based method relying on spherical harmonic shape descriptors that localizes submillimetric variations of volume between a given structure and a template while guaranteeing anatomical correspondence across subjects,28 a requisite for reliable statistics. In our experience, this technique is effective in detecting subtle atrophy in patients with normal whole hippocampal volume. Our most recent method extracts a medial sheet for each subfield (i.e., a surface running along its central path), allowing for dense feature sampling with only minimal partial volume contamination (Figure 6.2). In addition to local volume, surface-based integration of other MRI contrasts, such as T2 intensity, and diffusion parameters allows one to obtain an increasingly detailed catalogue of metrics for a comprehensive in vivo phenotyping of mesiotemporal pathology in individual patients.24, 25 Importantly, this approach accurately lateralizes the seizure focus and predicts pathological MTS grades in single patients.
Figure 6.2. Multiparameter hippocampal subfield subface analysis. (A) Hippocampal subfield labels are generated on high-resolution T1-weighted MRI images. (B) Labels are then converted to surfaces and parameterized using a spherical harmonics approach, providing shape inherent subject correspondence. For each subfield surface, a medial sheet is extracted by solving a three-dimensional Hamilton-Jacobi equation from the outer hull. The sheet inherits correspondence from the parameterized hull by vertex propagation along a Laplacian field. (C) This representation allows for dense sampling of volume (derived from T1-weighted MRI), T2-signal intensity, and diffusion-weighted MRI features (MD = mean diffusivity; FA = fractional anisotropy) with minimal partial volume effect along the entire anatomical extent of each subfield (CA1-3, CA4-dentate gyrus, and subiculum).
The contemporary shift toward evidence-based practice promotes computational neuroimaging as a means to provide novel biomarkers for diagnostics and prognostics. In this context, data mining is a method of choice to extract critical patterns from high-dimensional datasets that might otherwise be missed. Combining shape analysis with machine learning, we were able to partition a seemingly homogeneous cohort of drug-resistant TLE with a unilateral seizure focus into distinct classes, with distinct histopathological signature, based on patterns of subregional volume variations across the hippocampus, amygdala, and entorhinal cortex.26 Importantly classifiers operating on these classes accurately predicted surgical outcome in >90% of patients, outperforming learners based solely on conventional MRI volumetry. Overall, this work highlights the role of computational modeling and machine learning to assist clinical decision making.
6.2.2 Beyond Mesiotemporal Structures
Beside mesiotemporal damage in the hippocampus, amygdala, and entorhinal cortex, histopathological examination of postmortem and surgical specimens indicates an extended distribution of abnormalities in TLE, preferentially involving limbic structures. Findings include impaired fiber arrangement of the fimbria-fornix, neuronal loss and gliosis in the ipsilateral mediodorsal thalamic nucleus, abnormal myelination and axonal degeneration of the temporal pole, and gliotic changes of the frontal pole and orbitofrontal cortex,29 anomalies indicative of widespread network alterations in this condition.
In addition to providing an increasingly detailed in vivo characterization of lesions, neuroimaging research has been instrumental in demonstrating network pathology in epilepsies traditionally considered as focal. In TLE, MRI morphometry has contributed to our understanding of system-level pathology by revealing widespread atrophy that extends beyond the mesial temporal into the lateral temporal and frontocentral cortices,30, 31 as well as the thalamus.32–34 Cortical thinning is thought to be secondary to excitotoxic effects of seizure spread through thalamocortical pathways. Indeed, the location and extent of neocortical atrophy relate to degree and distribution of thalamic pathology, lending support to the concept that this deep brain structure is an important hub in the pathologic network of TLE.32 Additional morphological alterations include increased folding complexity of the insular and cingulate regions, mainly ipsilateral to the seizure focus,35 and decreased surface area, particularly in the temporal lobe.36 The presence of such anomalies in the asymptomatic siblings suggests, at least in part, genetic influence.37
With respect to the white matter, diffusion MRI studies have shown altered fractional anisotropy and mean diffusivity suggestive of decreased axonal density and altered myelin membranes in limbic tracts, including the fornix and cingulum, and frontotemporal tracts, such as the uncinate and arcuate fasciculi, the temporo-occipital tract, the inferior longitudinal fasciculus, as well as the corpus callosum.38, 39 Notably, a track-based segment analysis revealed a centrifugal pattern of mean diffusivity increases in major tracts carrying temporal lobe connections.40 These anomalies likely reflect astrogliosis and microstructure derangement related to seizure activity in the vicinity of the focus, which may serve as a dynamic marker of the epileptic activity and lateralize the seizure focus, particularly in patients with unremarkable MRI. Given its proximity to the neocortex and key role in cortico-cortical connectivity, superficial white matter (SWM) is a key candidate region to evaluate the interplay between structure and function. We recently designed a surface-based method allowing for continuous sampling of diffusion parameters in this compartment,41 a region so far neglected in TLE neuroimaging studies. Our analysis revealed ipsilateral anomalies in the temporolimbic regions that were independent from cortical thinning; conversely, they related to hippocampal volume. Interestingly, grouping patients based on histopathology showed higher load of SWM diffusion alterations in cases with cell loss and gliosis compared to those with isolated gliosis,41 cross-validating the relationship between mestiotemporal sclerosis and SWM microstructural anomalies. Notably, the proximity of SWM alterations to the mesiotemporal lobe and close relationship to hippocampal pathology may corroborate connectivity-based models of regional susceptibility, in which regions anatomically connected to the disease epicenter may undergo most marked structural alterations.42 Importantly, we showed that SWM damage, mediated by hippocampal atrophy, resulted in large-scale default mode anomalies,41 a link to be targeted by future longitudinal studies in new-onset epilepsy, ideally complemented by behavioral phenotyping to understand the cognitive consequences of this causal chain.
6.3 Computational Imaging of Focal Cortical Dysplasia
Malformations of cortical development are found in 20% of specimens obtained during epilepsy surgery. Among them, FCD is the most common, accounting for 71% of cases.5 In FCD Type I, an elusive pathological entity, microcolumns are typified by more than eight vertically aligned neurons, predominantly located in layers 3 and 4.43 Compared to healthy cortices, regions harboring microcolumns may present with reduced cell size, increased neuronal density, as well as a tendency for decreased cortical thickness.44 Notably, as the microcolumnar pattern resembles the unit of the radial lineage model of corticogenesis, its presence suggests a remnant of perturbed events affecting the final, postmigrational neuronal positioning.45 Currently, Type I dysplasias cannot be seen on MRI. Indeed, the strictly intracortical character of FCD Type I lesions together with the lack of large-scale cytological anomalies has so far precluded a clear definition of their signature even on high-resolution 3T MRI.46 Moving toward ultra-high-field 7T MRI offers the possibility to increase spatial resolution to the range of 350–500 µm, a scale at which cortical layers may be visualized in vivo.47 Paralleling increases in resolution, quantitative contrasts provide time-efficient measurements of longitudinal T1 and transverse T2 relaxation times, which may distinguish cortical myelin and iron,48 two determinants of cortical laminar architecture. Case reports have shown encouraging increased sensitivity for the detection of subtle FCD Type II lesions,49 setting the conceptual basis for the use in Type I lesions.
Cortical dysplasias Type II are typified by both disorganization of layers and cytological anomalies,43 including dysmorphic neurons and balloon cells. Ectopic neurons, and hypertrophic astrocytes may be present in the juxtacortical white matter, which may also show demyelination. On MRI, FCD Type II lesions are typically characterized by visible increased cortical thickness, blurred gray-white matter interface, and increased T2-weighted signal, particularly apparent on FLAIR images.9 Notably, these lesions may vary greatly in size, ranging from those that affect extended cortical territories to a few voxels only. The transmantle sign, a funnel-shaped hyperintensity extending from the FCD to the lateral ventricle and thought to represent the footprint of disrupted neuronal migration along radial glial processes may help detecting such smaller lesions. In addition, subtle dysplasias may be associated with unusual gyral patterns and are preferentially located at a bottom of an abnormally deep sulcus.50 As a result, conventional radiological assessment, even when carried out by expert observers, often fails to detect such lesions.9 This clinical challenge has motivated the development of computer-aided methods aimed at objectively detecting FCD lesions by means of tissue modeling, detailed in the next section.
6.3.1 Automated Detection of FCD Type II Lesions
While voxel-based morphometry was originally developed to quantify group differences in gray matter density derived from T1-weighted MRI, several studies have used this approach to identify “excessive” gray matter associated with FCD in individual patients. Applying an arbitrary threshold (e.g., >1 SD above the mean gray matter concentration in healthy controls) as indicative of abnormality, areas of increased density colocalize with lesional voxels in 63–86% of cases.51 Notably, the marked hyperintensity of some lesions leads to gray matter misclassification, reducing considerably the sensitivity of the technique.51 Moreover, while this method may give diagnostic confidence in detecting medium to large lesions, its yield in small FCD has not been demonstrated. The analysis of MRI intensities or quantitative MRI contrasts, such as T2 relaxometry, double inversion recovery, and magnetization transfer ratio imaging, are also amenable to voxel-based comparison. These approaches have a sensitivity of 87–100% in detecting obvious FCD lesions,9 but have low yield in cases with negative conventional MRI.
Our group pioneered the design of computer-based algorithms modeling the distinctive characteristics of FCD Type II.52, 53 Initial methods targeted cortical thickening and blurring of the GM-WM boundary on T1-weighted MRI, enhancing visual detection rates by 30% relative to standard evaluation. Other groups have replicated our techniques with similar results.54–56 Notably, sensitivity and specificity may be hampered by the fact that various maps are inspected visually. In addition, the yield and diagnostic confidence depend heavily on the reader’s ability to integrate the diverse and complex information embedded in the various maps, an expertise that only few centers have developed so far. Limited generalizability also stems from the fact that such approaches have been validated using only large lesions easily recognized by routine radiology.55 Another source of difficulty may arise from the paucity or lack of localizing clinical and EEG findings to direct the search for FCD, particularly in patients with seizures originating in the frontal lobe, the preferential location of this malformation. To overcome these challenges, over the years, we have developed a series of increasingly sophisticated algorithms for automatic FCD detection, operating on 1.5 T MRI data, which initially relied on voxel-based texture analysis combined with a Bayesian classifier.57 These tools were validated with mid- to large-sized lesions visible on routine radiological inspection; nevertheless, classification failed in up to 20% of cases. Such performance is unsatisfactory given current referral patterns to epilepsy surgery centers, with an increasing number of patients with nondiagnostic clinical MRI, even at 3.0 Tesla. Indeed, the absence of a visible lesion is one of the greatest challenges in epilepsy surgery and has led to an increase in invasive EEG studies with implanted intracranial electrodes. Yet, without informed, image-guided implantation, even with widespread coverage, EEG sampling errors may occur in up to 40% of cases; consequently the target cannot be defined and the outcome of surgery, if considered, is poorer.4 In these patients, lesions are subtle, with morphological characteristics that may differ only slightly from normal tissue.
As an alternative approach to voxel-based techniques, surface-based methods preserve cortical topology and allow quantifying sulco-gyral anomalies, at times the only sign of dysgenesis. We thus recently opted for a surface-based framework and combined various morphological (cortical thickness, curvature, and sulcal depth), intensity features (relative intensity and intensity gradients), microstructure (mean diffusivity and fractional anisotropy), and function, taking advantage of their covariance to unveil subthreshold tissue properties not readily identified by a single modality. To characterize in vivo MRI signatures of FCD Type IIA and Type IIB, we have designed a multisurface approach that systematically sampled intra- and subcortical lesional features.58 In addition, geodesic distance mapping quantified the same features in the lesion perimeter. Logistic regression assessed the relationship between MRI and histology, while supervised pattern learning was used for individualized subtype prediction. In this comprehensive analysis, we demonstrated that FCD Type IIB is characterized by abnormal morphology, intensity, diffusivity, and connectivity across all surfaces, while Type IIA lesions present only with increased FLAIR signal and reduced diffusion anisotropy close to the gray-white matter interface. Similar to lesional patterns, perilesional anomalies in all domains were more marked in Type IIB, extending up to 16 mm from the manual lesion label. Importantly, structural MRI markers correlated with categorical histological characteristics. Finally, a profile-based classifier predicted FCD subtypes with equal sensitivity of 85%, while maintaining a high specificity of 94% against healthy and disease controls. This work demonstrated that image processing applied to widely available MRI contrasts has the ability to dissociate FCD subtypes at a mesoscopic level. Integrating in vivo staging of pathological traits with automated lesion detection may be clinically relevant, as it could conceivably assist emerging approaches, such as minimally invasive thermal ablation, which do not supply tissue specimen. It may also help guiding and monitoring novel lesion-specific drug treatments, such as mTOR inhibitors.
We recently designed a surface-based two-step classifier for automated FCD detection of “MRI-negative FCD.59 This procedure, which relies on a linear discriminant classification scheme of multiple features derived from T1-weighted MRI, first recognizes vertices with the highest detection rate and subsequently removes false positive clusters using tissue texture characteristics and spatial priors (Figure 6.3A). Given that all subjects were initially diagnosed as MRI-negative, this fully automated approach yielding 74% sensitivity in healthy and disease controls offers a substantial gain in sensitivity over standard radiological assessment (Figure 6.3B). When applying our classifier trained on 3.0 Tesla images to an independent dataset of patients with histologically proven FCD acquired at 1.5 Tesla, we maintained high sensitivity (71%), supporting generalizability. Notably, using T1 and FLAIR intensities, we subsequently achieved even higher sensitivity (83%) and specificity (92%).60
Figure 6.3. Automated detection of focal cortical dysplasia (FCD) Type II. (A) After surface-based extraction of morphology and intensity-based features modeling the in vivo characteristics of FCD, a vertex-wise classification identifies putative lesions (red), whereas the subsequent cluster-wise classification discards false positives except the cluster (blue) colocalizing with the manual label (green). The axial T1-weighted MRI sections show the region containing the FCD (dashed square). The magnified panel displays two manually segmented FCD labels (dotted squares) and their volume; the label is projected onto a surface template. (B) The flow diagram and study design of the only classifier with Class II evidence for diagnostic accuracy are shown. Sources of spectrum bias were ruled out by evaluating the specificity of the algorithm against healthy individuals and clinically well-characterized disease controls. To minimize incorporation bias, the classifier trained on 3.0 Tesla data was tested on an independent cohort of patients and controls examined at 1.5 Tesla.
6.3.2 Assessing Brain-Wide Integrity in Cortical Dysplasias
Because of the crucial role in defining the surgical target, MRI studies in FCD have been primarily dedicated to lesion detection in single patients, as discussed in the previous section. Aside from a few case reports describing gray matter and white anomalies,61 whole-brain cohort-specific structural brain anomalies have been only rarely assessed. To fill this knowledge gap, we recently examined systematically whole-brain MRI morphology in dysplasia-related frontal lobe epilepsy46 and found that, relative to controls, patients with FCD Type I display multilobar cortical atrophy that is most marked in ipsilateral frontal cortices. Conversely, in Type II FCD, anomalies beyond the primary lesions are typified by multilobar frontocentral cortical thickening. Cortical folding also diverged, with increased complexity in prefrontal cortices in Type I and decreases in Type II. We hypothesized that cortical thickening in Type II may indicate delayed pruning, while a thin cortex in Type I likely results from combined effects of seizure excitotoxicity and the primary malformation. In addition, group-level patterns successfully guided automated subtype classification (Type I: 100%, Type II: 96%), seizure focus lateralization (Type I: 92%, Type II: 86%), and outcome prediction (Type I: 92%, Type II: 82%), thereby demonstrating clinical relevance.
We assessed large-scale brain organization across the entire spectrum of prevalent cortical malformations.62 Based on experimental evidence suggesting that distributed effects of focal insults are modulated by stages of brain development, we postulated differential patterns of network anomalies across subtypes of malformations. Graph theoretical analysis of structural covariance networks indicated a consistent rearrangement toward a regularized architecture characterized by increased path length and clustering, as well as disrupted rich-club topology, overall suggestive of inefficient global and excessive local connectivity. Notably, we observed a gradual shift in network reconfigurations across subgroups, with only subtle changes in FCD Type II, moderate effects in heterotopia, and maximal effects in polymicrogyria (Figure 6.4). Analysis of resting-state functional connectivity also revealed gradual network changes, with most marked rearrangement in polymicrogyria; contrary to findings in the structural domain, however, functional architecture was characterized by decreases in both local and global parameters. These findings support the concept that time of insult during corticogenesis impacts the severity of topological network reconfiguration. Specifically, late-stage malformations, typified by polymicrogyria, may disrupt the formation of large-scale cortico-cortical networks and thus lead to a more profound impact on whole-brain organization than early stage disturbances of predominantly radial migration patterns observed in cortical dysplasia Type II, which likely affect a relatively confined cortical territory.
Figure 6.4. Structural network alterations across the spectrum of malformations of cortical development. (A) Covariance networks based on cortical thickness correlations in healthy controls (n = 82), and patients with malformations of cortical development (n = 154), including focal cortical dysplasia Type II (FCD-II), heterotopia (HET), and polymicrogyria (PMG). The structural covariance matrix is obtained by calculating the cross-correlation coefficient of mean cortical thickness between 78 pairs of regions i and j across subjects. (B) For each cohort (i.e., controls, FCD-II, HET, and PMG), a group-level matrix is obtained. For display purposes, parcels are color coded according to brain regions listed beside the matrices. The color bar indicates the correlation strength. (C) Significant group differences in interregional correlations corrected for multiple comparisons at FDR < 0.05. Increase/decrease in patients relative to controls are shown in red/blue in the matrices and corresponding network graphs. In (B) and (C), L and R refer to left and right hemispheres, respectively.
6.4 Computational Imaging of Idiopathic Generalized Epilepsy
Idiopathic generalized epilepsies (IGE) form a group of electroclinical syndromes characterized by absence seizures, myoclonic jerks, and generalized tonic-clonic seizures (alone or in varying combinations) in association with generalized discharges of spikes and waves in the presence of a normal EEG background. A handful of postmortem observations have reported heterotopic neurons in the white matter, an indistinct cortical boundary and dyslamination;63 nevertheless, these findings have not been reproduced.64 Advanced quantitative image postprocessing of structural MRI has identified subtle thalamic and cortical changes.65, 66 Notably, however, findings from manual MRI volumetry of the thalamus have been somewhat heterogeneous: initial studies were negative,67 while more recent data have shown atrophy68 or both atrophy and hypertrophy within a single cohort.65 Similarly, whole-brain, lobe-wise volumetry has provided uncertain results.69 These results may reflect not only the heterogeneity of structural anomalies across subsyndromes, but also interrater variability of manual segmentations. Indeed, evidence from VBM analyses showed consistent reduction in thalamic70 and neocortical gray matter density.71 Using cortical thickness measures and thalamic volumetry, we showed bilateral thalamic atrophy corresponding to known anatomical connections from sacrificial tracer studies and widespread cortical thinning that was most prominent in frontocentral areas, with a prevalence of up to 40%. In patients, thalamocortical network correlations increased in frontocentral and parietal regions, but decreased in limbic areas. Importantly, patients with poorly controlled seizures showed an even faster progression of atrophy of these neocortical regions, suggesting that neocortical atrophy in IGE is likely the consequence of generalized seizure activity inducing thalamocortical network remodeling.66 Similar distributions of neocortical atrophy have been reported in juvenile myoclonic epilepsy.72 One study demonstrated widespread changes in mean curvature, a surrogate marker of abnormal brain development, suggesting that structural anomalies in juvenile myoclonic epilepsy extend beyond the thalamus and frontal lobes.73 Studies employing structural and functional MRI also found abnormal network organization, with structure-function decoupling,74 although findings were only partially reproduced.75
6.5 Conclusion
By revealing subtle lesions that previously eluded visual inspection, quantitative image processing of structural MRI has clearly demonstrated increased sensitivity compared to traditional visual inspection. Overall, the most significant clinical impact of postprocessing is that cases with pharmacoresistant seizures considered MRI-negative at first have been increasingly become MRI-positive, thereby offering the life-changing benefits of epilepsy surgery to more patients. Aside from diagnostics, ongoing developments in computational imaging, combined with machine learning, lend us unprecedented opportunities to design novel biomarkers to stage and monitor the disease, and ultimately provide personalized therapeutic interventions.
References









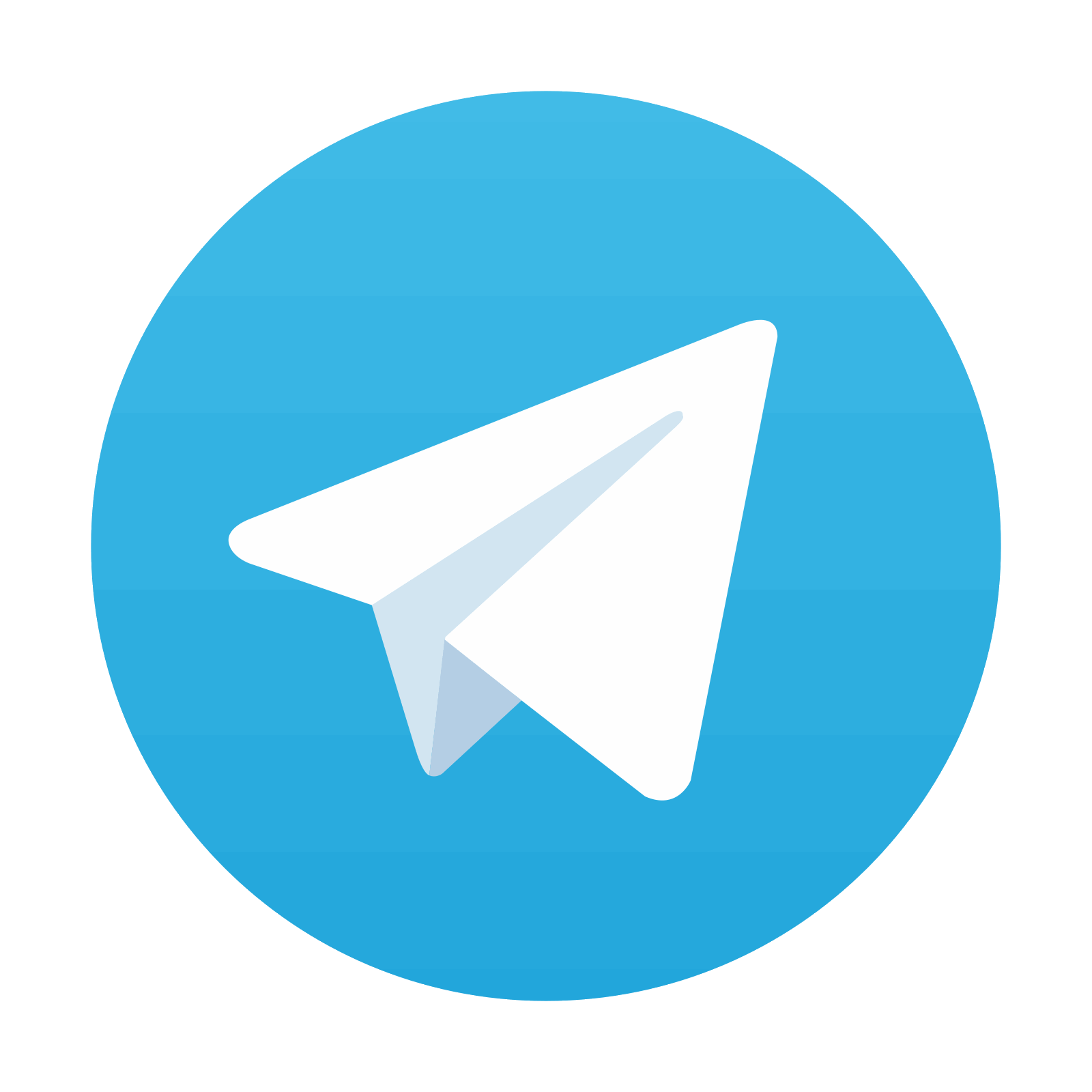
Stay updated, free articles. Join our Telegram channel

Full access? Get Clinical Tree
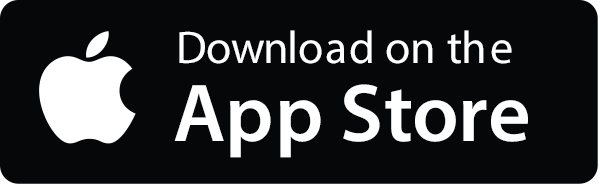

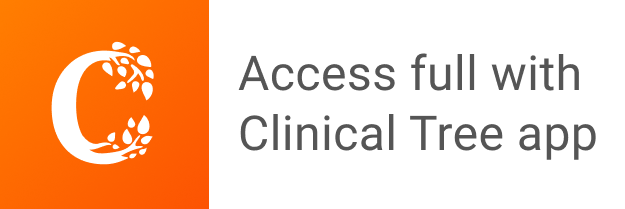